Causality Analysis with Information Geometry: A Comparison.
Entropy(2023)
摘要
The quantification of causality is vital for understanding various important phenomena in nature and laboratories, such as brain networks, environmental dynamics, and pathologies. The two most widely used methods for measuring causality are Granger Causality (GC) and Transfer Entropy (TE), which rely on measuring the improvement in the prediction of one process based on the knowledge of another process at an earlier time. However, they have their own limitations, e.g., in applications to nonlinear, non-stationary data, or non-parametric models. In this study, we propose an alternative approach to quantify causality through information geometry that overcomes such limitations. Specifically, based on the information rate that measures the rate of change of the time-dependent distribution, we develop a model-free approach called information rate causality that captures the occurrence of the causality based on the change in the distribution of one process caused by another. This measurement is suitable for analyzing numerically generated non-stationary, nonlinear data. The latter are generated by simulating different types of discrete autoregressive models which contain linear and nonlinear interactions in unidirectional and bidirectional time-series signals. Our results show that information rate causalitycan capture the coupling of both linear and nonlinear data better than GC and TE in the several examples explored in the paper.
更多查看译文
关键词
causality, information geometry, Transfer Entropy, Granger Causality, information causal rate, signal processing, nonlinear models, non-stationary, probability distribution
AI 理解论文
溯源树
样例
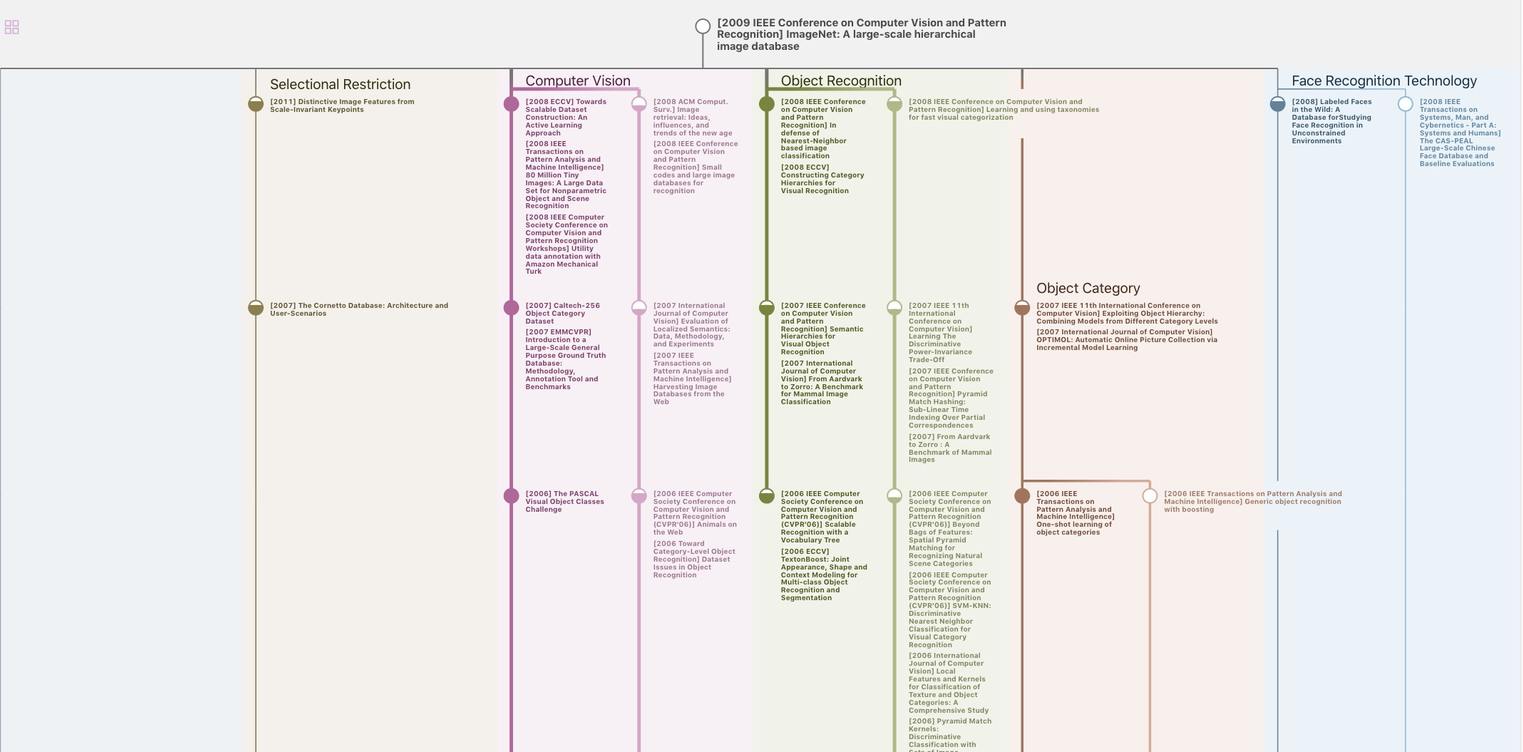
生成溯源树,研究论文发展脉络
Chat Paper
正在生成论文摘要