Deep Deterministic Policy Gradient in Acoustic to Articulatory Inversion
2022 12th International Conference on Computer and Knowledge Engineering (ICCKE)(2022)
摘要
This paper aims to utilize a deep reinforcement learning algorithm for the acoustic-to-articulatory inversion problem. A deep deterministic policy gradient (DDPG) based method is adopted to adjust the articulatory parameters of a speaker to minimize the cepstral difference among the main speech and the synthesized one. In traditional methods such as neural networks and Gaussian mixture models, a comprehensive dataset of both speech signal and articulatory information is needed for each speaker, but the proposed iterative DDPG is used to explore articulatory space for finding the best point, which maximizes the desired reward without any need for joint kinematic and articulatory data of the speaker. Acoustic signals are synthesized by VocalTractLab(VTL), a three-dimensional articulatory synthesizer, and represented by Mel-frequency cepstral coefficients (MFCCs). This method provides estimated parameters very close to those calculated by MRI and advanced processing.
更多查看译文
关键词
Speech synthesis,Acoustic-to-articulatory mapping,Reinforcement learning,DDPG,MFCC
AI 理解论文
溯源树
样例
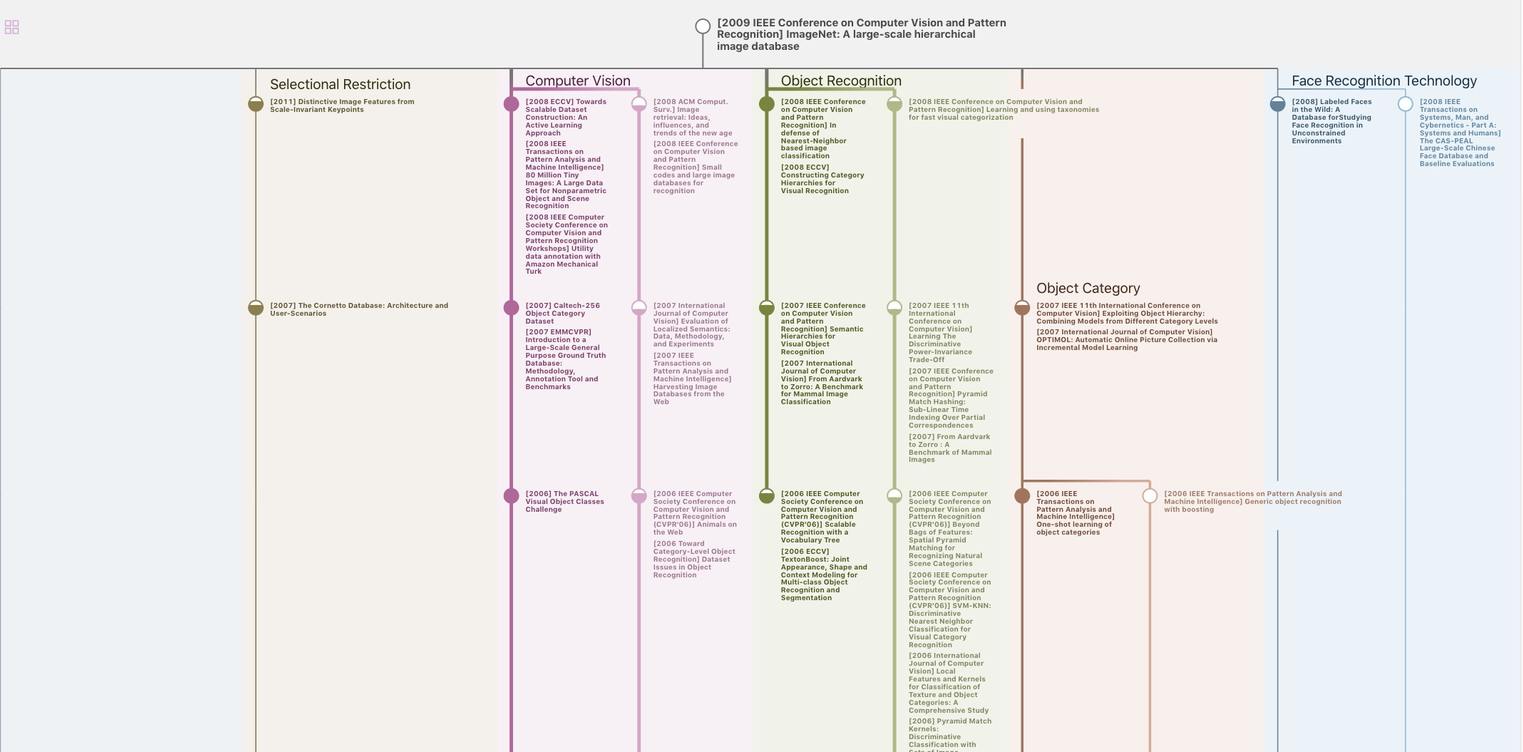
生成溯源树,研究论文发展脉络
Chat Paper
正在生成论文摘要