Deep Inside Tor: Exploring Website Fingerprinting Attacks on Tor Traffic in Realistic Settings
2022 12th International Conference on Computer and Knowledge Engineering (ICCKE)(2022)
摘要
In recent years, with the new advances in the areas of machine learning, Tor’s advertised anonymity has been widely threatened. Despite all the protection mechanisms employed by Tor, attackers can now draw inferences on the online activities of a Tor user. Although such an study is critical for users of Tor, most of the existing works in this regard are based on unrealistic assumptions and settings. In this work, we explore the effectiveness of fingerprinting attacks under realistic settings. We focus on identifying the target websites and applications visited or used by a Tor user, through analyzing the heavily encrypted traffic that any local eavesdropper can also see. Unlike existing works, we focus on small groups of consecutive packets, which allows us to study more complex user behavior. By modifying our Tor client to label the Tor cells with the name of the destined application or website name, could label the packets even when multiple websites and applications were simultaneously using the Tor proxy. To label the network packets, the byte sequence of a labeled cell was located inside the packets, which, to the best of our knowledge, is the most accurate way for labeling the packets. In this way, we accomplished to gather an extensive dataset of Tor traffic, corresponding to different types of user’s behavior. Finally, we proposed several deep neural network structures to classify the packets of different websites and analyzed the effectiveness of Tor’s encryption methods in realistic settings by achieving an accuracy of 3% in classifying 100 different websites. The results show the effectiveness of Tor’s multi-layer encryption scheme.
更多查看译文
AI 理解论文
溯源树
样例
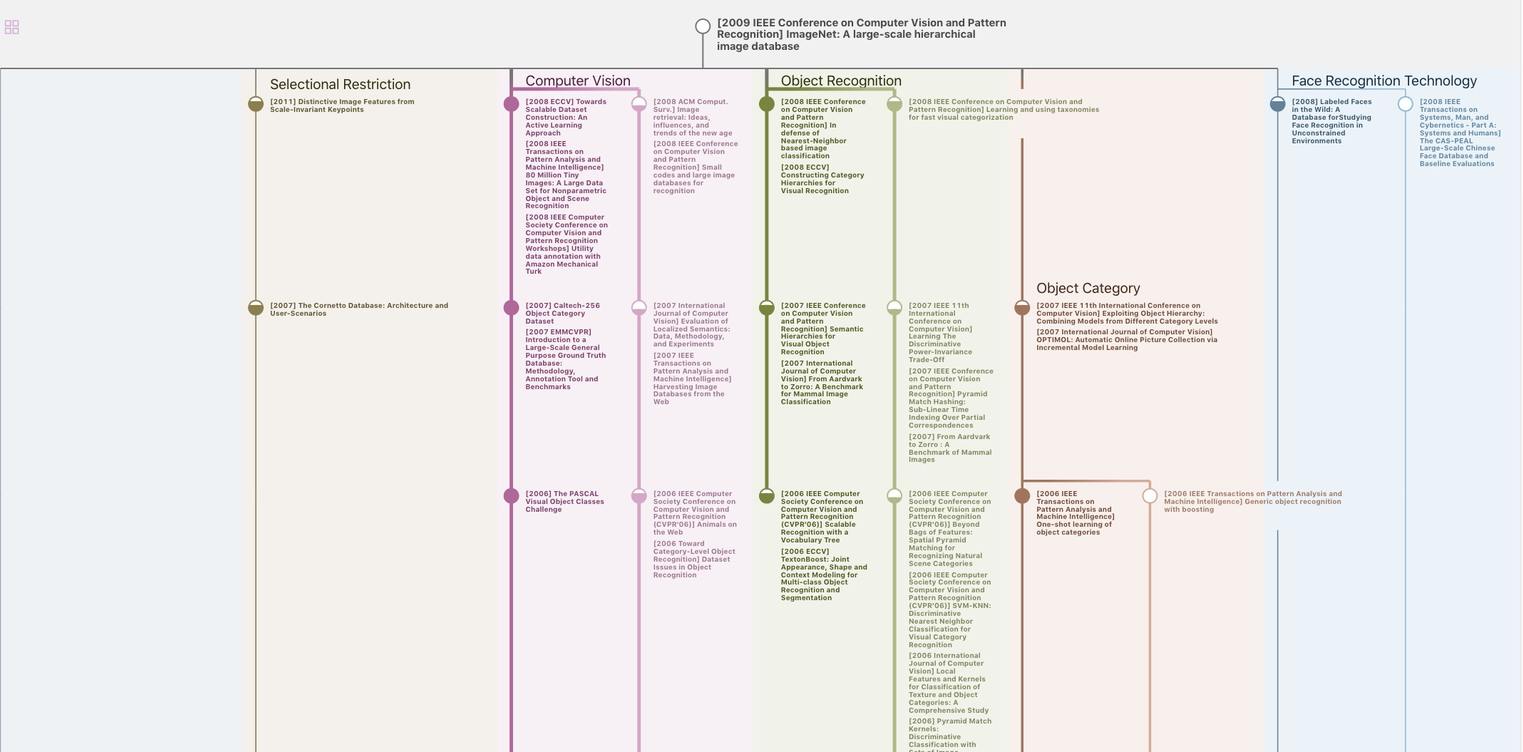
生成溯源树,研究论文发展脉络
Chat Paper
正在生成论文摘要