Assessing Anonymous and Selfish Free-rider Attacks in Federated Learning
2022 IEEE Symposium on Computers and Communications (ISCC)(2022)
摘要
Federated Learning (FL) is a distributed learning framework and gains interest due to protecting the privacy of participants. Thus, if some participants are free-riders who are attackers without contributing any computation resources and privacy data, the model faces privacy leakage and inferior performance. In this paper, we explore and define two free-rider attack scenarios, anonymous and selfish free-rider attacks. Then we propose two methods, namely novel and advanced methods, to construct these two attacks. Extensive experiment results reveal the effectiveness in terms of the less deviation with conventional FL using the novel method, and high false positive rate to puzzle defense model using the advanced method.
更多查看译文
关键词
federated learning,privacy data,free-rider attack
AI 理解论文
溯源树
样例
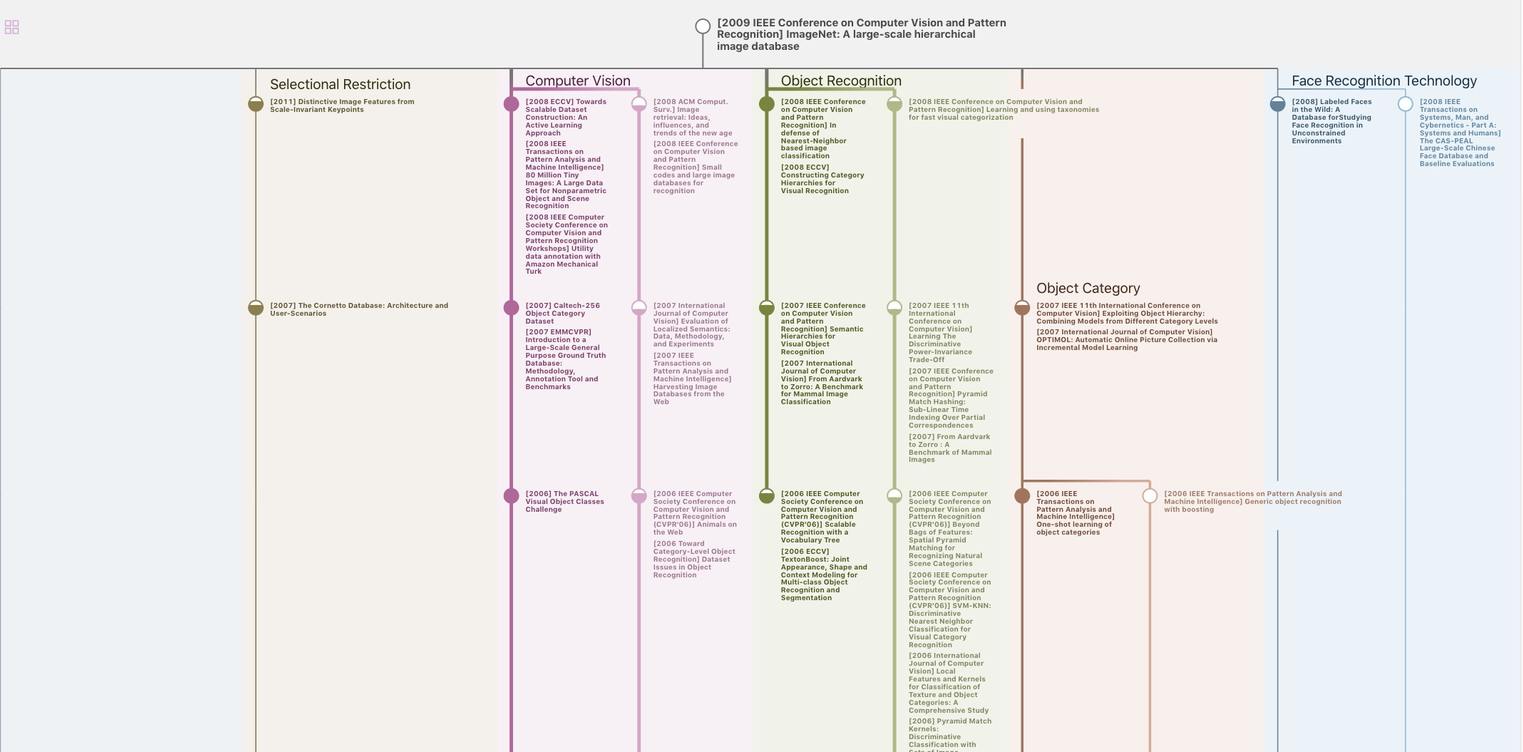
生成溯源树,研究论文发展脉络
Chat Paper
正在生成论文摘要