SMOTE-Stack for Network Intrusion Detection in an IoT Environment
2022 IEEE Symposium on Computers and Communications (ISCC)(2022)
摘要
In recent years, there has been a notable surge in the Internet of Things (IoT) applications. Increasingly, IoT devices are being attacked. Network intrusion detection is a tool to detect any presence of malicious activities in a network. Machine learning (ML) techniques are increasingly used for classifying network traffic. However, results from state-of-the-art studies have shown that training ML classifiers with imbalanced datasets affect their classification performance, resulting in net-work categories with fewer training instances getting classified wrongly. This study presents a Stack ensemble ML classifier for network intrusion detection in an IoT network using the Bot-IoT dataset for the classifier evaluation. According to preliminary results, the classifier showed lower metric scores for minority network categories. We applied Synthetic Minority Oversampling Technique (SMOTE) to address the class imbalance. Follow-up experiment results for the SMOTE-Stack outclassed Stack and other state-of-the-art classifiers.
更多查看译文
关键词
Network Intrusion Detection,Machine Learning,Ensemble Learning,Stack,SMOTE,SMOTE-Stack,IoT
AI 理解论文
溯源树
样例
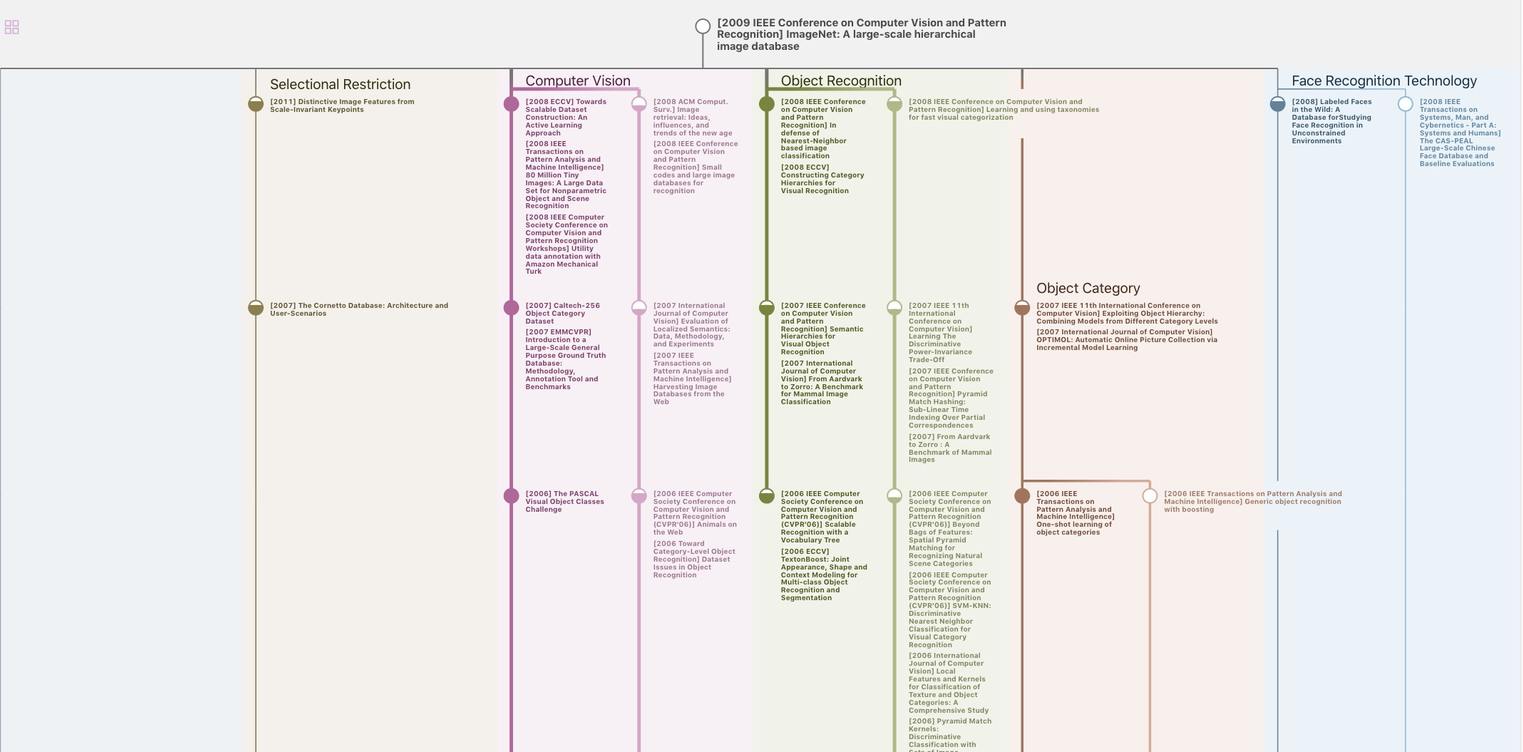
生成溯源树,研究论文发展脉络
Chat Paper
正在生成论文摘要