Look-Ahead Reinforcement Learning for Load Balancing Network Traffic
2022 IEEE Symposium on Computers and Communications (ISCC)(2022)
摘要
Given the growth in complexity and scale of computer networks and considering that the leading cause of failures is human error, there is an increasing interest in minimizing the role of humans in network management. In this context, we propose a two-step, machine learning approach for automatically balancing network flows. Firstly, we rely on identifying flows that can more heavily impact the network, i.e., elephant flows. Sec-ondly, we use reinforcement learning to determine the best action to be performed in the network, given its state. The intuition for this two-step approach is to amortize the computational costs of reinforcement learning and apply it only to flows that can cause a high impact on network performance. Our main contributions are (i) problem modeling as a function of states and actions to balance network traffic and (ii) an architecture that more judiciously uses reinforcement learning on flows of interest for load balancing.
更多查看译文
关键词
reinforcement learning,load balancing,network resources,traffic prediction,elephant flow
AI 理解论文
溯源树
样例
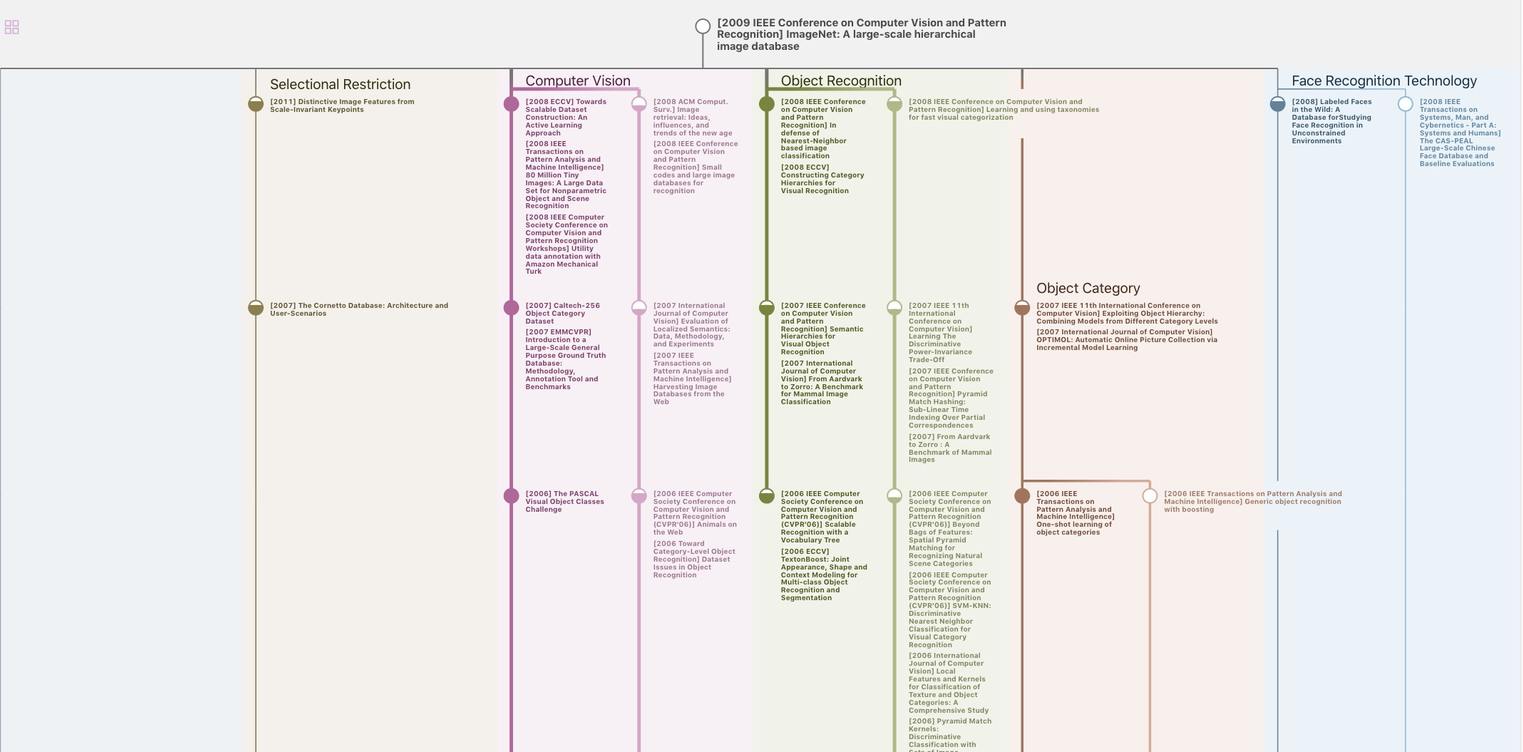
生成溯源树,研究论文发展脉络
Chat Paper
正在生成论文摘要