Microgrid Scheduling Optimization under False Data Injection Attack
2022 International Conference on Computing, Communication, Perception and Quantum Technology (CCPQT)(2022)
摘要
False data injection attacks (FDIAs) pose a great threat to the data security of microgrid. The day-ahead scheduling strategy of microgrid is in turn mainly based on microgrid operation data. Under FDIAs, it is important for the efficiency and economic cost of microgrid scheduling to properly repair the attacked data and optimize the scheduling using predictive data from deep learning. In this work, we model the economic cost of microgrid scheduling, use deep learning prediction algorithm to predict data based on historical values of microgrid operation, and repair the attacked data by the predicted data, and combine the optimization algorithm to optimize microgrid day-ahead power scheduling to make scheduling more economical and efficient. By this way, the malicious action was corrected and system recover as normal performance to avoid the economical lost that FDIAs aimed to attrite.
更多查看译文
关键词
FDIAs,microgrid,scheduling,optimization,Edge Computing
AI 理解论文
溯源树
样例
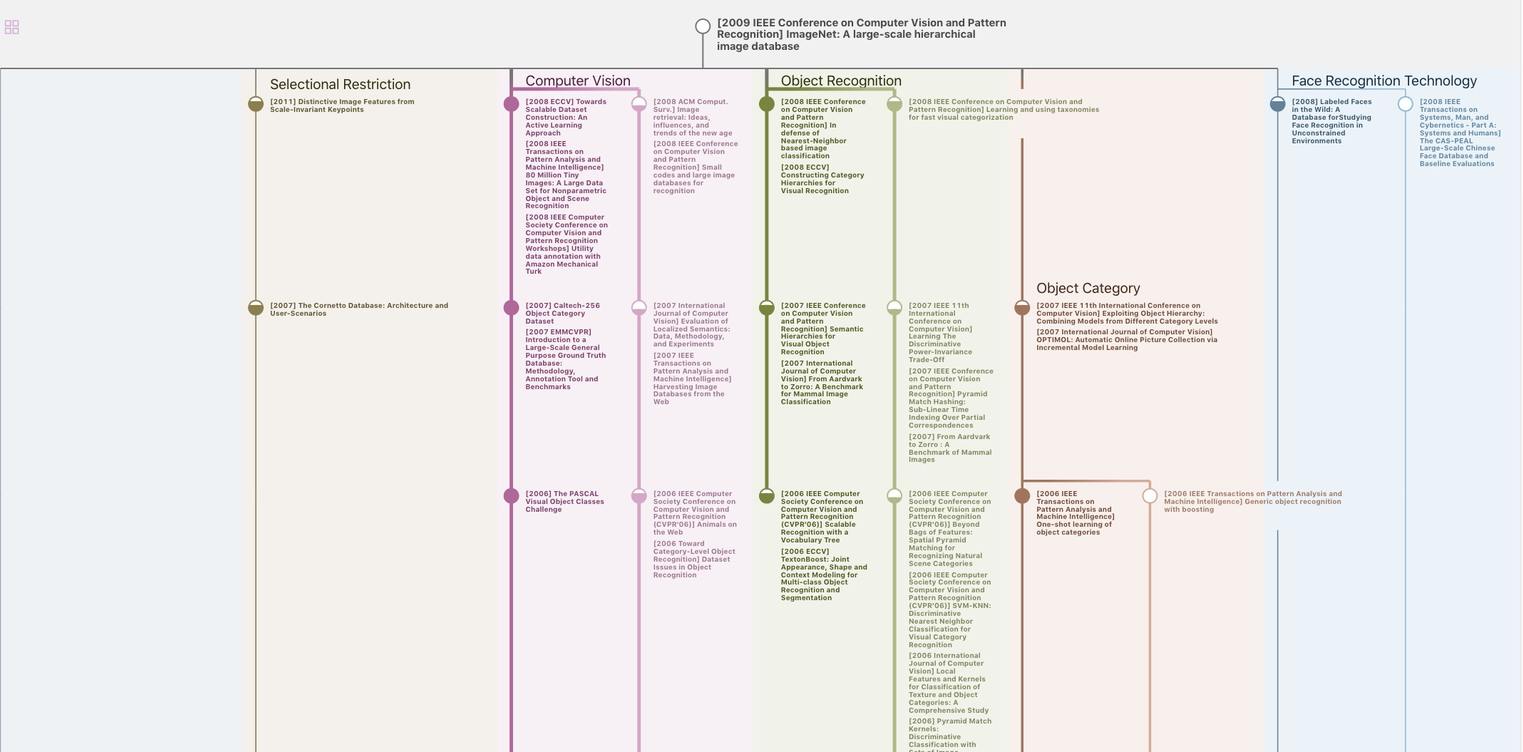
生成溯源树,研究论文发展脉络
Chat Paper
正在生成论文摘要