Adaptive Random Testing based on the Modified Metric-memory Tree and Information Entropy
2022 9th International Conference on Dependable Systems and Their Applications (DSA)(2022)
摘要
Adaptive Random Testing (ART) is a series of testing techniques proposed as an enhancement to random testing (RT). ART achieves better failure-detection than RT by distributing test cases more evenly throughout the input domain. However, the process of selecting test cases leads to significant computational costs and the increase of dimension leads to the decrease of effectiveness. In this paper, we propose a new ART approach to improve the efficiency and effectiveness of Fixed-size-Candidate-set ART (FSCS-ART) by applying a modified Metric-Memory tree and distance information entropy selection strategy, namely FSCS based on the modified MM-tree and information entropy (MMIE-FSCS). Simulations and empirical studies are conducted to examine the efficiency and effectiveness of the approach. The experimental results show that the approach reduces the computational cost of FSCS-ART and improves failure-detection effectiveness.
更多查看译文
关键词
Adaptive random testing,Fixed-size-Candidate-set ART,Metric-Memory tree,Information entropy
AI 理解论文
溯源树
样例
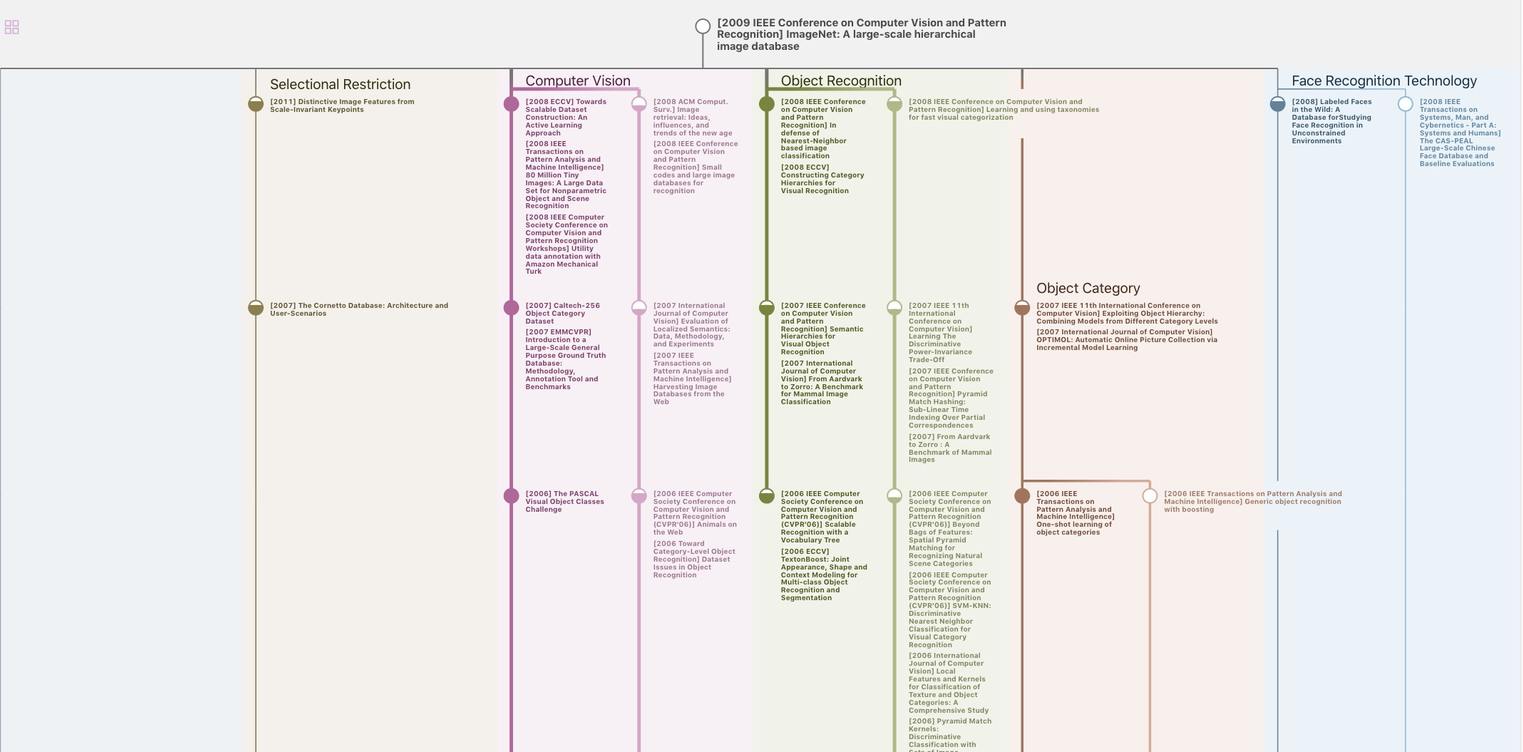
生成溯源树,研究论文发展脉络
Chat Paper
正在生成论文摘要