Low-Rank Representation With Morphological-Attribute-Filter Based Regularization For Hyperspectral Anomaly Detection
2022 12th Workshop on Hyperspectral Imaging and Signal Processing: Evolution in Remote Sensing (WHISPERS)(2022)
摘要
Hyperspectral anomaly detection (HAD) has attracted extensive interests because of its broad applications in both military and civilian. In recent years, morphological-attribute-filter based method has been applied to the HAD task achieving impressive performances. How-ever, how to introduce morphological attribute filters into convex-optimization-based HAD models is still an unsolved problem. In this paper, for the first time, we designed a morphological attribute filter-based regularizer to assist the low-rank representation model in utilizing morphological spatial structure information. The newly proposed model is called as background-suppression regularized low-rank representation (BSLRR). Furthermore, we design a customized automatic dictionary construction scheme for facilitating the practical applicability of BSLRR. Experiments show that BSLRR has certain advantages over benchmark methods.
更多查看译文
关键词
Hyperspectral image,anomaly detection,low-rank representation,dictionary construction,morphological attribute filter
AI 理解论文
溯源树
样例
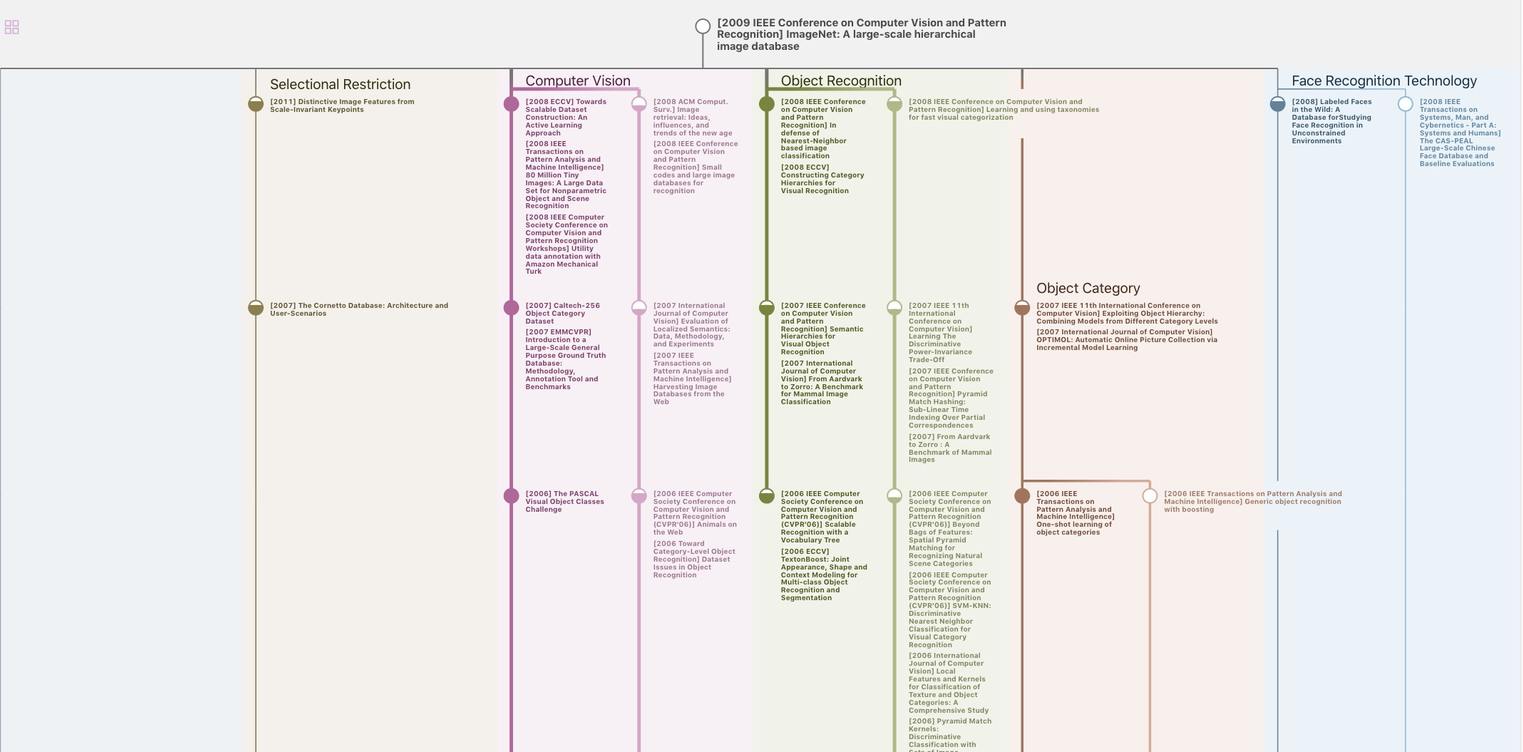
生成溯源树,研究论文发展脉络
Chat Paper
正在生成论文摘要