Effect of spectral resolution on the segmentation quality of magnetic resonance imaging data
2022 IEEE 26th International Conference on Intelligent Engineering Systems (INES)(2022)
摘要
The majority of the machine learning methods employed for brain tissue or tumor segmentation from multi-spectral MRI data, especially the ensemble learning methods, use hundreds of attributes for the characterization of the pixels, which leads to enormous storage space requirement. Dealing with hundreds of volumetric records under such circumstances also represents a severe computational burden. To facilitate the establishment and deployment of such data processing frameworks, this paper proposes to investigate, which is the best trade-off between segmentation accuracy and necessary storage space, via manipulating with the spectral resolution used for the attributes of the pixels. Three machine learning methods, two multi-spectral brain MRI datasets, and five statistical indicators of segmentation accuracy were involved in the experimental study, which revealed that an 8-bit color depth or spectral resolution of the feature data is sufficient to obtain the finest achievable segmentation accuracy, while allowing for up to 50% reduction of the memory required by the segmentation procedure, compared to the commonly deployed feature encoding techniques.
更多查看译文
关键词
image segmentation,spectral resolution,magnetic resonance imaging,machine learning,brain tumor segmentation,brain tissue segmentation
AI 理解论文
溯源树
样例
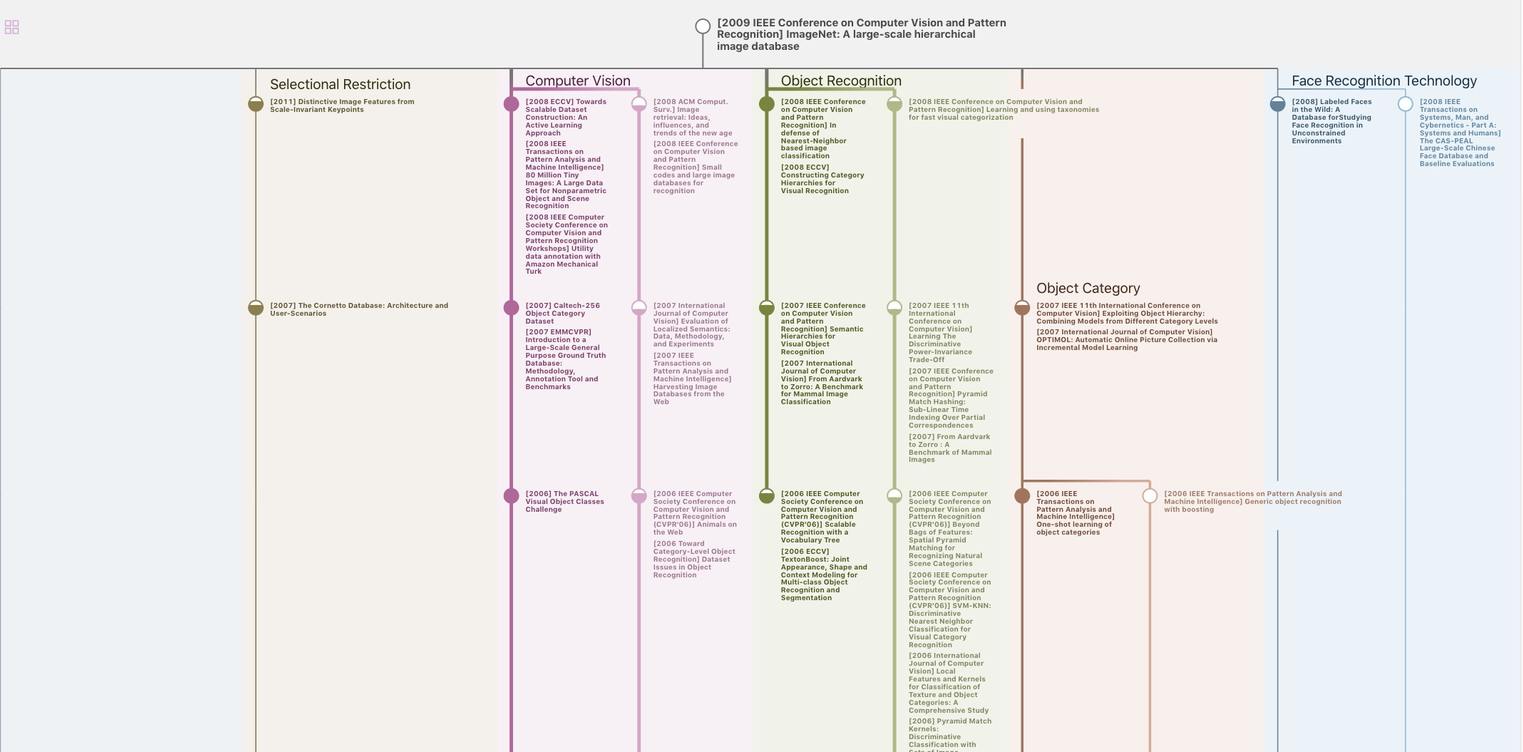
生成溯源树,研究论文发展脉络
Chat Paper
正在生成论文摘要