Monitoring Behaviors of Broiler Chickens at Different Ages with Deep Learning
ANIMALS(2022)
摘要
Simple Summary Animal behavior in the poultry house could be used as an indicator of health and welfare status. In this study, a convolutional neural network models (CNN) network model was developed to monitor chicken behaviors (i.e., feeding, drinking, standing, and resting). Videos of broilers at different ages were used to build datasets for training the new model, which was compared to several other deep learning frameworks in behavior monitoring. In addition, an attention mechanism module was introduced into the new model to further analyze the influence of attention mechanism on the performance of the network model. This study provides a basis for innovating approach for poultry behavior detection in commercial houses. Animal behavior monitoring allows the gathering of animal health information and living habits and is an important technical means in precision animal farming. To quickly and accurately identify the behavior of broilers at different days, we adopted different deep learning behavior recognition models. Firstly, the top-view images of broilers at 2, 9, 16 and 23 days were obtained. In each stage, 300 images of each of the four broilers behaviors (i.e., feeding, drinking, standing, and resting) were segmented, totaling 4800 images. After image augmentation processing, 10,200 images were generated for each day including 8000 training sets, 2000 validation sets, and 200 testing sets. Finally, the performance of different convolutional neural network models (CNN) in broiler behavior recognition at different days was analyzed. The results show that the overall performance of the DenseNet-264 network was the best, with the accuracy rates of 88.5%, 97%, 94.5%, and 90% when birds were 2, 9, 16 and 23 days old, respectively. In addition, the efficient channel attention was introduced into the DenseNet-264 network (ECA-DenseNet-264), and the results (accuracy rates: 85%, 95%, 92%, 89.5%) confirmed that the DenseNet-264 network was still the best overall. The research results demonstrate that it is feasible to apply deep learning technology to monitor the behavior of broilers at different days.
更多查看译文
关键词
poultry production,animal welfare,deep learning,behavior recognition,DenseNet
AI 理解论文
溯源树
样例
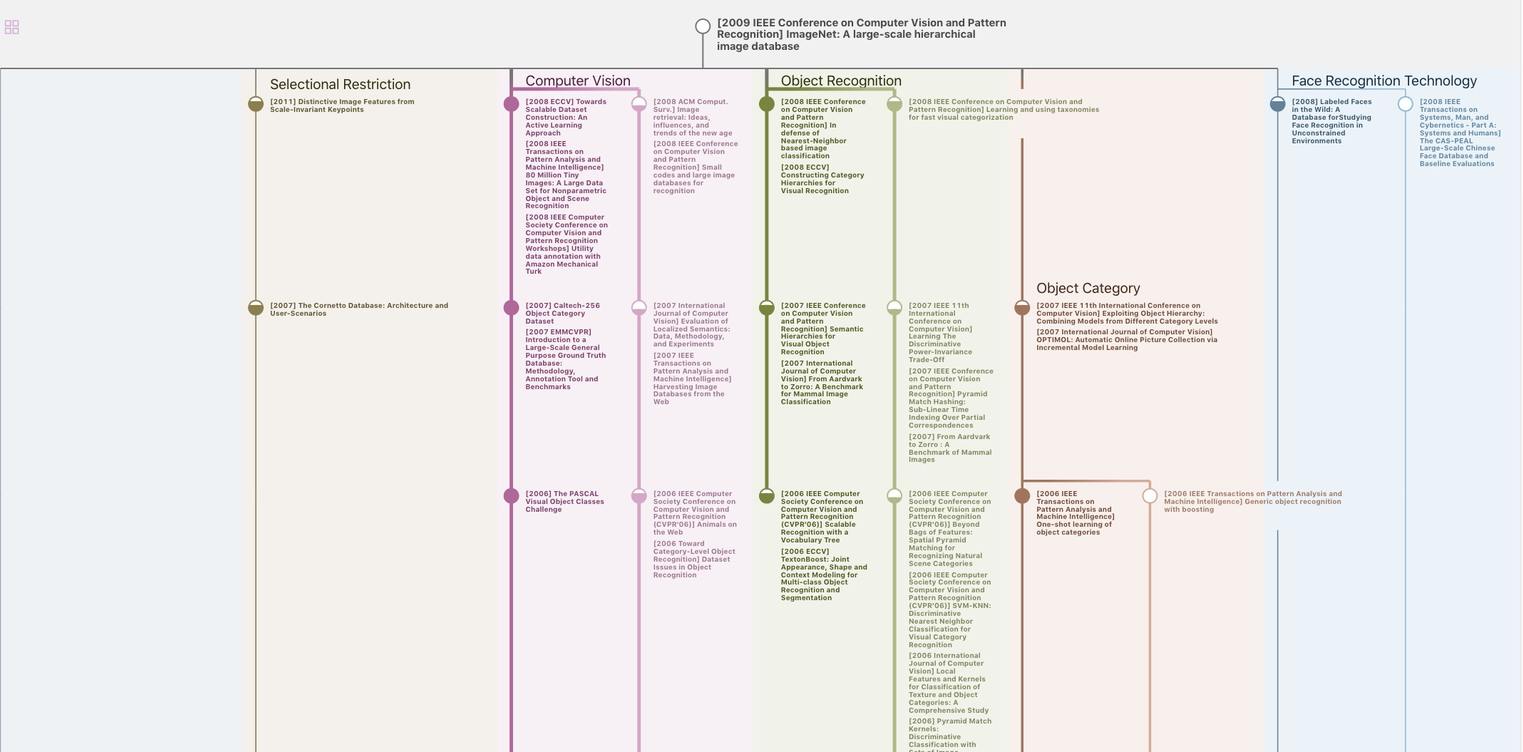
生成溯源树,研究论文发展脉络
Chat Paper
正在生成论文摘要