Exploring the effect of waterbodies coupled with other environmental parameters to model PM2.5 over Delhi-NCT in northwest India
Atmospheric Pollution Research(2022)
摘要
The problem of air pollution, especially, the concentration of PM2.5 is a long-standing issue over the national capital territory of India (Delhi- NCT). This study seeks to explore the effect of surface waterbody on the spatial variation of PM2.5 in Delhi-NCT. As moisture determines the concentration of PM2.5 and other pollutants in the atmosphere, the presence of surface waterbody plays a vital role in controlling PM2.5 in the local environment. We use distance to waterbodies, including other covariates of meteorology and surface characteristics, such as the surface greenness and land cover type, to model near the surface concentration of PM2.5. Different machine learning (ML) approaches were applied to predict the spatio-temporal behavior of PM2.5. Out of different ML-based models, the random forest regression produces the highest predictive accuracy (R2 = 0.68), while, gradient boosting, support vector machine and artificial neural net have R2 less than 0.66. The outcome of the feature important score from random forest regression reveals that proximity to the water surface is more important than precipitation and land use and land cover (LULC). The results find that up to a distance of 3 km, the waterbody exerts a significant effect in lowering PM2.5 concentration. The findings suggest that restoring the surface waterbodies – moderate to large in size, including the other prescribed measures could potentially restore the air quality standards for the Delhi-NCT and elsewhere in the world where particulate matter pollution is of great concern.
更多查看译文
关键词
PM2.5,Waterbody,Air quality,Delhi-NCT,Machine learning,Random forest
AI 理解论文
溯源树
样例
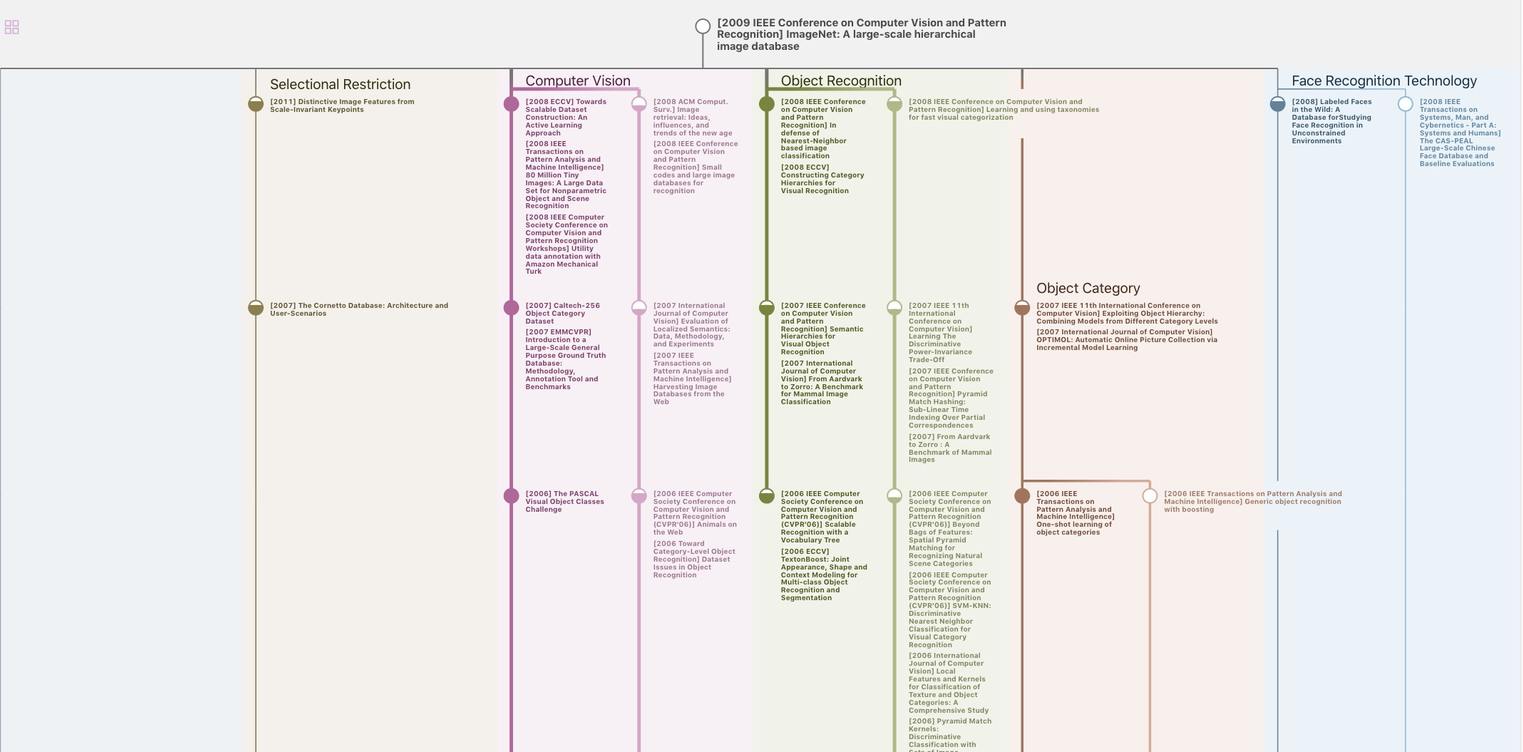
生成溯源树,研究论文发展脉络
Chat Paper
正在生成论文摘要