Updating VNF deployment with Scaling Actions using Reinforcement Algorithms
2022 23rd Asia-Pacific Network Operations and Management Symposium (APNOMS)(2022)
摘要
Softwarization of the internet network is promising for network service providers (NSPs) to satisfy various types of user requests while dynamically operating the networking system. However, it is challenging to provide optimal service quality as the complexity of the network increases. In particular, deployment of the VNF instances is a critical issue in providing better QoS while maintaining resources optimally. For the management of the VNF deployment task, dynamic programming algorithms are only feasible in small networks or rely on heuristics. In this paper, we propose a VNF deployment method based on reinforcement learning (RL) that can effectively satisfy the QoS with minimized resource consumption in the network. Our approach makes adjustment decisions, which are scale-in/keep/out for target nodes and target VNF types given ILP-based deployments. We formulate this VNF deployment task as RL by setting the reward as QoS and resources. Moreover, we propose a model architecture for our RL agent's policy, based on Graph Neural Network. In the experiment, our approach optimizes VNF deployment with improved QoS while keeping the similar or slightly less amount of resources, compared to ILP-based deployment.
更多查看译文
关键词
NFV,VNF deployment,Auto-Scaling,Reinforcement Learning
AI 理解论文
溯源树
样例
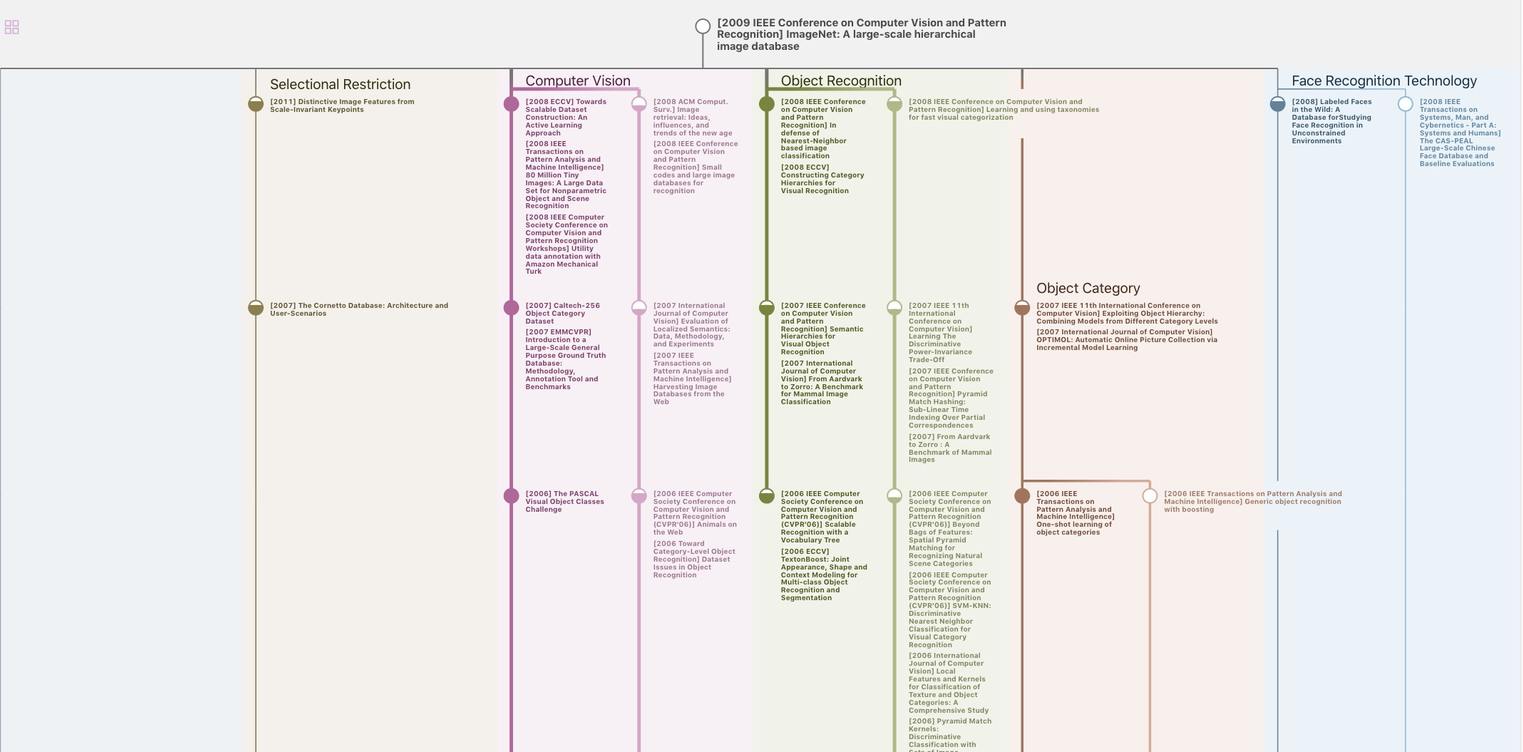
生成溯源树,研究论文发展脉络
Chat Paper
正在生成论文摘要