FPGA-Based Acceleration of Convolutional Neural Network for Gesture Recognition Using mm-Wave FMCW Radar
2022 IEEE Nordic Circuits and Systems Conference (NorCAS)(2022)
摘要
Convolutional Neural Networks (CNNs) have revo-lutionized many applications in recent years, especially in image classification, video processing, and pattern recognition. Another exciting area where CNNs are becoming popular is studying the interaction between humans and computers, also known as human-computer interaction (HCI). Our study uses CNNs to classify hand gestures (a common non-contact-based HCI) obtained using mmWave Frequency-Modulated Continuous Wave (FMCW) radar. For each gesture, features such as range, velocity, and angle, are obtained and fused in one feature map, forming one single input to the CNN model. The proposed CNN model has achieved exceptional accuracy of 99.33% on the test set. Additionally, we have discussed the complete workflow from radar data preprocessing and model compression (quantization and pruning) to the final implementation of the CNN accelerator on FPGAs. With a minimal accuracy degradation, the most optimized CNN accelerator can process 64474.53 samples per second and consume 11.1 times fewer DSP units, 1.27 times fewer BRAMs, 1.37 times fewer flip flops, and 2.52 times reduction in 100kup tables (LUTs) compared to its baseline model.
更多查看译文
关键词
FMCW,radar,FPGAs,convolutional neural network,quantization
AI 理解论文
溯源树
样例
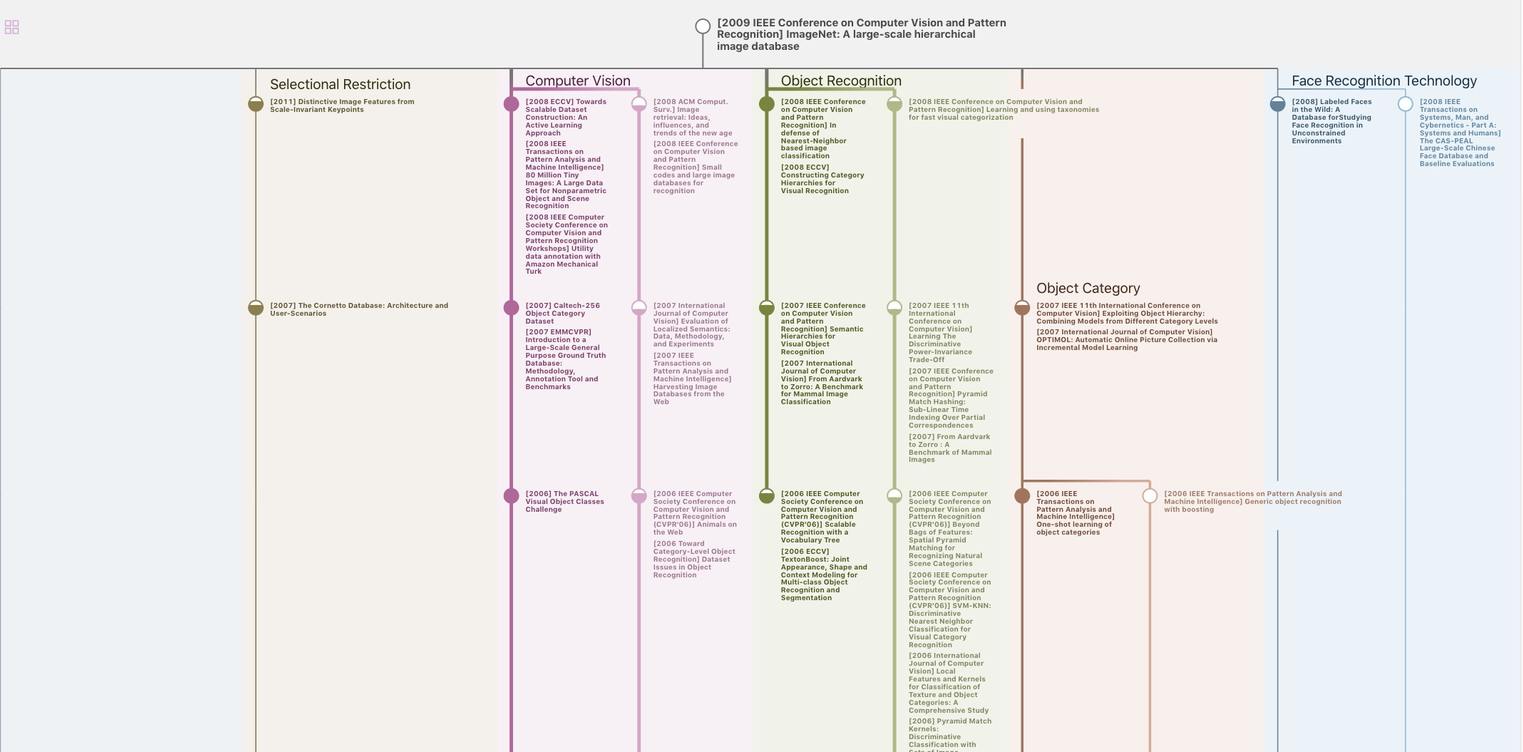
生成溯源树,研究论文发展脉络
Chat Paper
正在生成论文摘要