Characterizing the memory capacity of transmon qubit reservoirs
2022 IEEE International Conference on Quantum Computing and Engineering (QCE)(2022)
摘要
Quantum Reservoir Computing (QRC) exploits the dynamics of quantum ensemble systems for machine learning. Numerical experiments show that quantum systems consisting of 5–7 qubits possess computational capabilities comparable to conventional recurrent neural networks of 100 to 500 nodes. Unlike traditional neural networks, we do not understand the guiding principles of reservoir design for high-performance information processing. Understanding the memory capacity of quantum reservoirs continues to be an open question. In this study, we focus on the task of characterizing the memory capacity of quantum reservoirs built using transmon devices provided by IBM. Our hybrid reservoir achieved a Normalized Mean Square Error (NMSE) of 6 × 10
–4
for the NARMA (Non-linear Auto-regressive Moving Average) task which is comparable to recent benchmarks. The Memory Capacity characterization of a n-qubit reservoir showed a systematic variation with the complexity of the topology and exhibited a peak for the configuration with n – 1 self-loops. Such a peak provides a basis for selecting the optimal design for forecasting tasks.
更多查看译文
关键词
Quantum Reservoir Computing,Memory Capacity,Time-series forecasting,Data Science
AI 理解论文
溯源树
样例
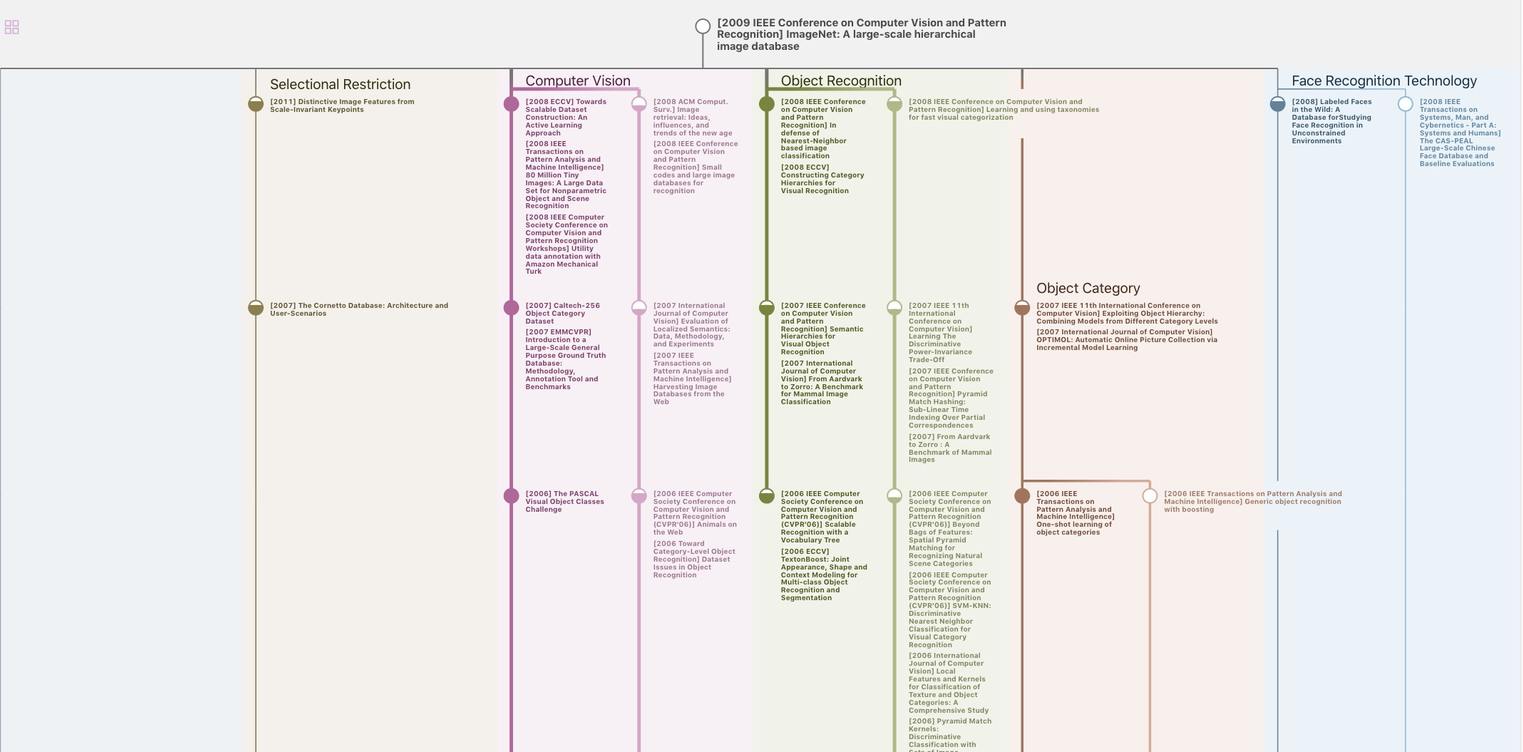
生成溯源树,研究论文发展脉络
Chat Paper
正在生成论文摘要