Retraining a BERT Model for Transfer Learning in Requirements Engineering: A Preliminary Study
2022 IEEE 30th International Requirements Engineering Conference (RE)(2022)
摘要
In recent years, advanced deep learning language models such as BERT, ELMO, ULMFiT and GPT have demonstrated strong performance on many general natural language processing (NLP) tasks. BERT, in particular, has also achieved promising results on some domain-specific tasks, including the requirements classification task. However, in spite of its great potential, BERT under-performs on domain specific tasks. In this paper, we present BERT4RE, a BERT-based model retrained on requirements texts, aiming to support a wide range of requirements engineering (RE) tasks, including classifying requirements, detecting language issues, identifying key domain concepts, and establishing requirements traceability links. We demonstrate the transferability of BERT4RE, by fine-tuning it for the task of identifying key domain concepts. Our preliminary study shows that BERT4RE achieved better results than the BERT
base
model on the demonstrated RE task.
更多查看译文
关键词
Requirements Engineering,Requirements Classification,Language Models,BERT,Domain-Specific Language Models,Transfer Learning,Deep Learning,Machine Learning,Natural Language Processing
AI 理解论文
溯源树
样例
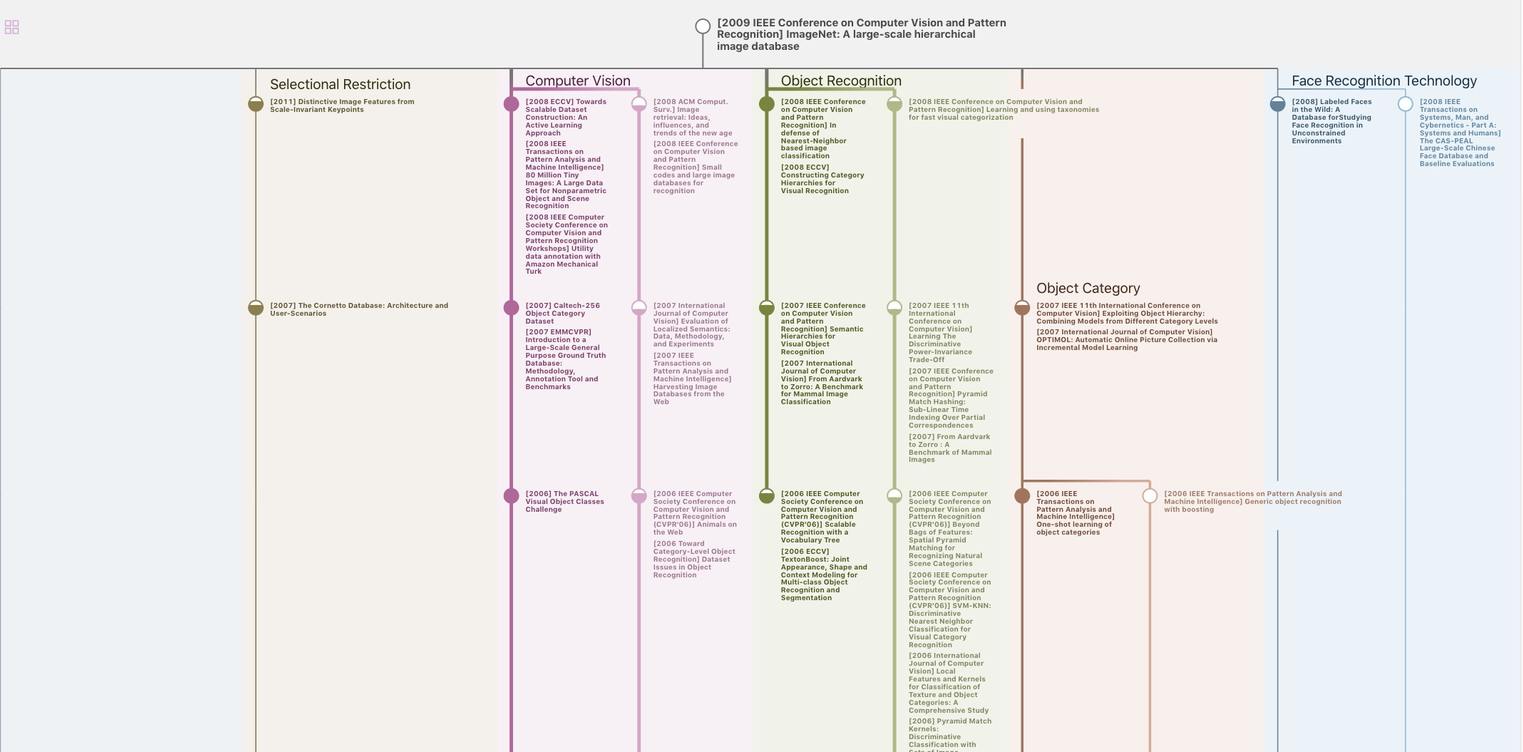
生成溯源树,研究论文发展脉络
Chat Paper
正在生成论文摘要