Improving Balance in Automatic Chord Recognition with Random Forests
2022 30th European Signal Processing Conference (EUSIPCO)(2022)
摘要
Automatic Chord Recognition (ACR) is a popular task to which Deep Learning (DL) has recently been successfully applied. ACR is considered as a classification problem wherein temporal frames of a piece of music are labelled according to some given chord vocabulary. When performing ACR using DL and larger chord vocabularies, an imbalance may be observed where popular chord classes are recognised more easily. In this paper, we propose random forest (RF) approaches in conjunction with existing DL strategies to mitigate such imbalance in ACR. We find improved balance in ACR is achieved when using DL-extracted chroma features alongside a RF balanced per-chord sampling strategy. Similar scores are achieved for accuracy and the balanced Average Chord Quality Accuracy (ACQA) metric on a Sevenths chord vocabulary, although the Sevenths accuracy is diminished relative to less balanced cases. Mapping the Sevenths estimations onto a MajMin vocabulary reveals little performance loss relative to initial MajMin estimations.
更多查看译文
关键词
automatic Chord Recognition,random forest,Automatic Chord Recognition,popular task,given chord vocabulary,performing ACR,larger chord vocabularies,popular chord classes,RF,per-chord sampling strategy,balanced Average Chord Quality Accuracy metric,Sevenths chord vocabulary,balanced cases,MajMin vocabulary
AI 理解论文
溯源树
样例
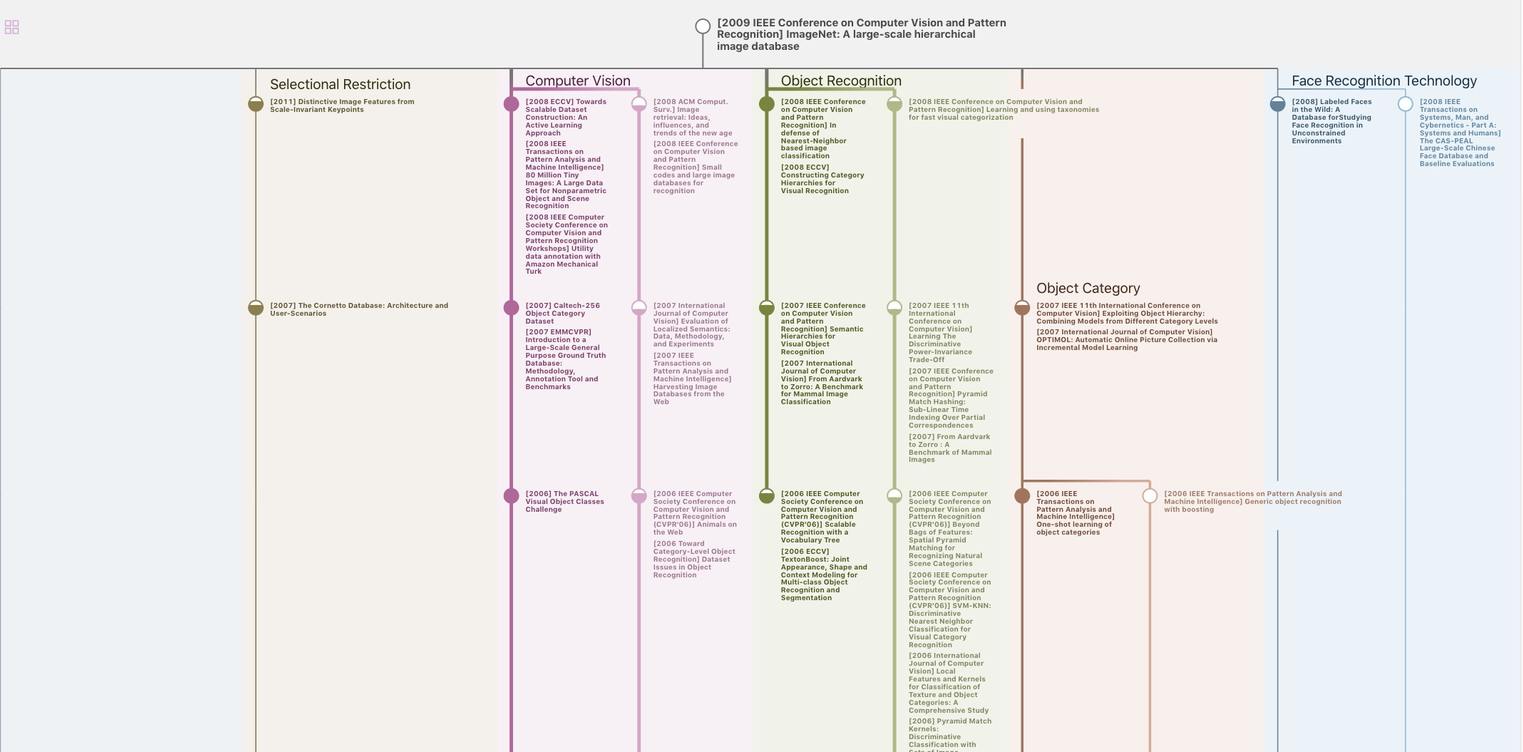
生成溯源树,研究论文发展脉络
Chat Paper
正在生成论文摘要