Configuration through Optimization for In-Memory Computing Hardware and Simulators
2022 IEEE Workshop on Signal Processing Systems (SiPS)(2022)
摘要
In-Memory Computing (IMC) technologies are designed to reduce computing latency and improve energy efficiency by processing data in the memory. This attribute can greatly improve the efficiency of neural network training and inference. However, the output variance and the lack of input-output simulators hinder the adoption of IMC hardware in real products. In this paper, we aim to simulate unknown IMC hardware with existing simulators to reduce the development time for a new simulator. By assuming that the output behavior should be similar if the devices are similar, we formulate the hardware-simulator matching by the configuration optimization problem. Specifically, we design an optimization goal based on measuring the correlation coefficient between the summarized model outputs, per-class accuracy, running on the hardware and the simulator. The experiment results show that our method can construct a smooth landscape to search for the optimal simulator setting and thus can be a practical solution.
更多查看译文
关键词
In-Memory Computing,Optimization,Machine Learning
AI 理解论文
溯源树
样例
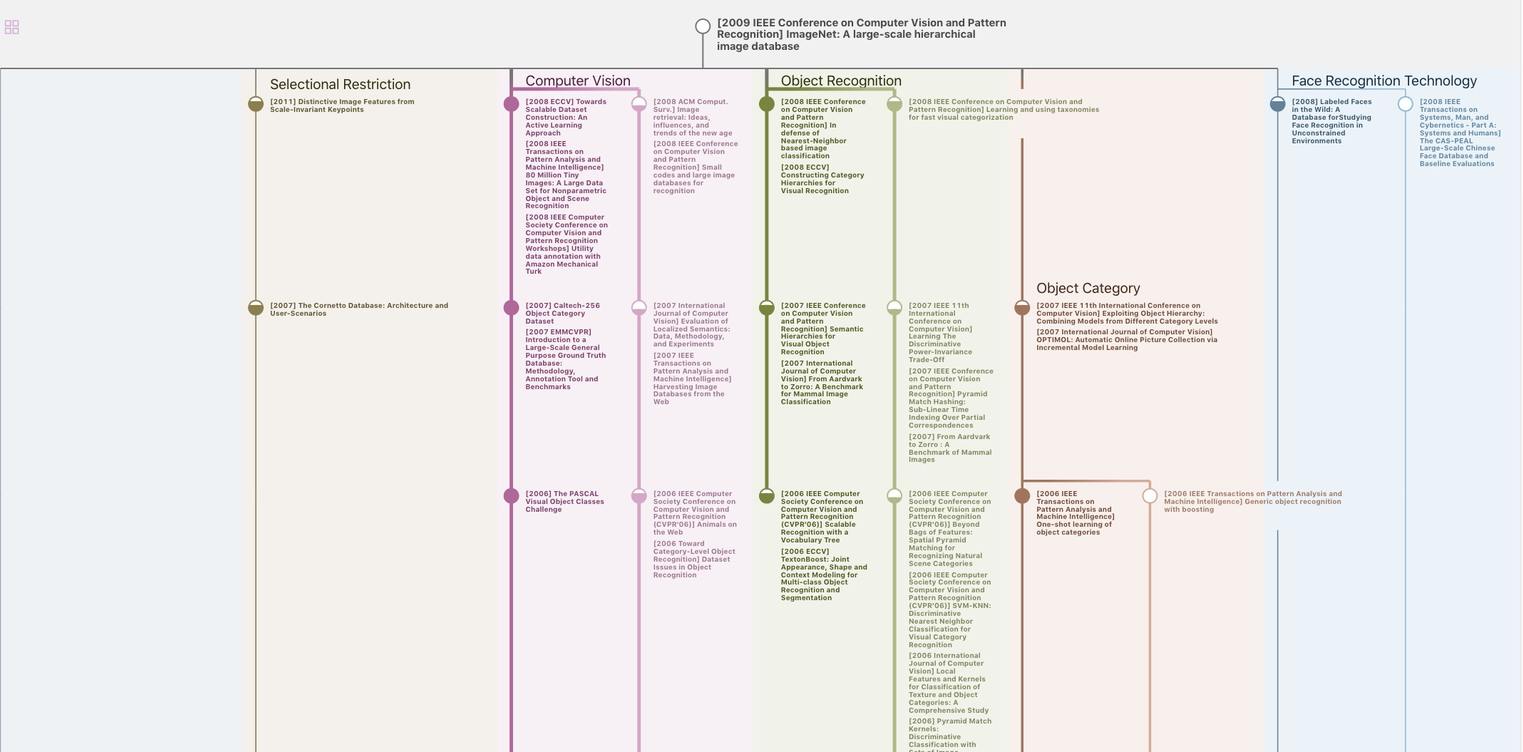
生成溯源树,研究论文发展脉络
Chat Paper
正在生成论文摘要