Electric Power Asynchronous Heterogeneous Data Accelerated Compression for Edge Computing
2022 IEEE 7th International Conference on Smart Cloud (SmartCloud)(2022)
摘要
With the development of edge computing and cloud computing in power scenarios, the cloud center collects a large amount of data from edge nodes every day, and the load of edge nodes is overloaded and the transmission delay increases, making it difficult to store and use data in the cloud center. The communication capabilities, storage capabilities and computing capabilities of nodes face greater challenges. Load data is the most important structural data in the asynchronous heterogeneous data of electric power. In order to reduce the amount of data generated during the transmission process of the edge network, compression technology can be used to effectively compress the load data. Before using the traditional integrated neural network model to compress the time series load data, it is necessary to calculate the variance of the window data, and compare the obtained variance with the empirical threshold, so as to divide the load data into stable data and unstable data. Due to the complex logic of data preprocessing, the overall compression calculation is time-consuming, and the robustness of the data classification algorithm is not high due to the need to manually set empirical parameters. In this paper, the multilayer perceptron is applied to load data classification, combined with the integrated neural network model, to construct an edge-side data compression scheme that can be applied to smart grid scenarios. This scheme achieves faster compression speed on the basis of ensuring the original compression ratio.
更多查看译文
关键词
data compression,MLP,edge computing,smart grid,time series data
AI 理解论文
溯源树
样例
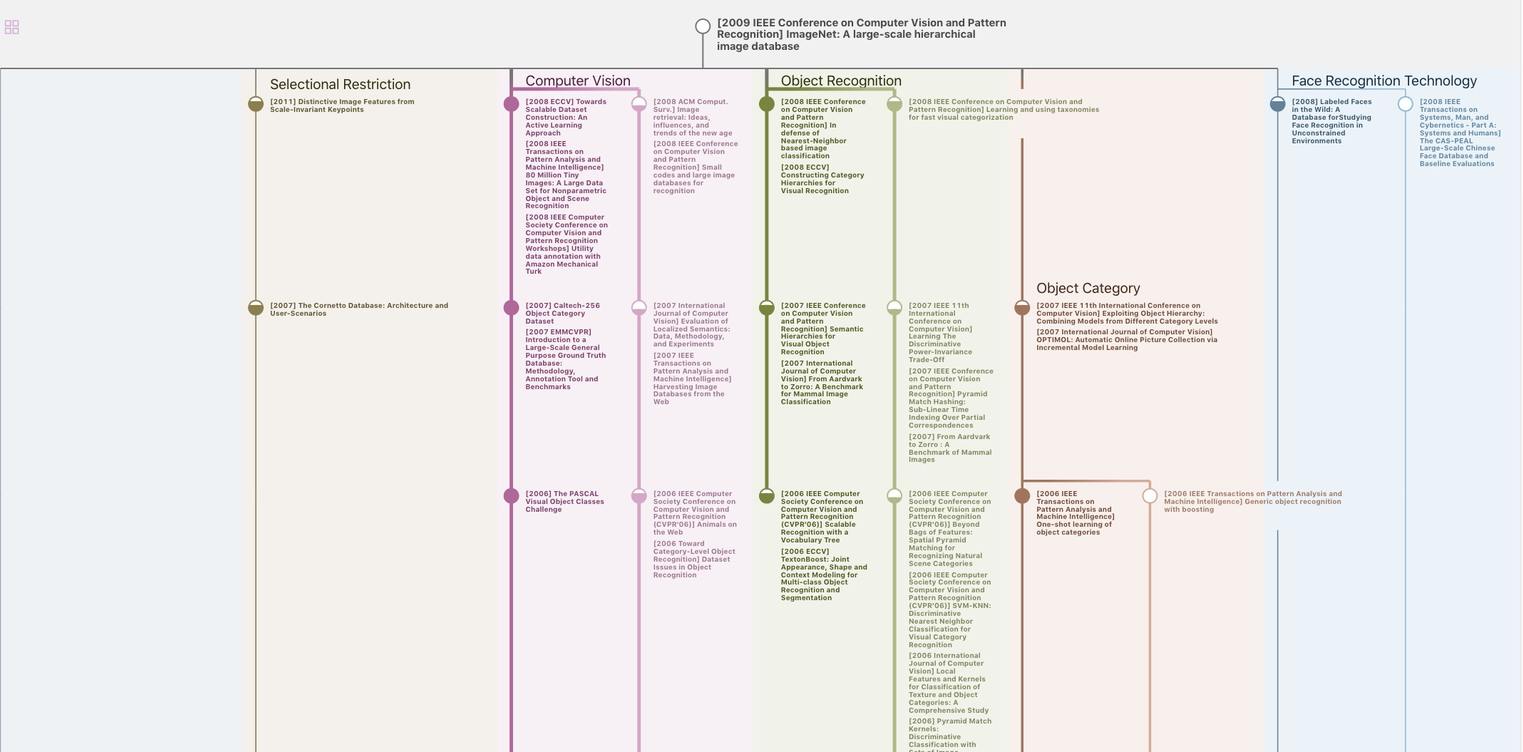
生成溯源树,研究论文发展脉络
Chat Paper
正在生成论文摘要