Machine Learning Methods for Feedforward Power Flow Control of Multi-Active-Bridge Converters
IEEE Transactions on Power Electronics(2023)
摘要
Controlling the multiway power flow in a multi-active-bridge (MAB) converter is important for achieving high performance and sophisticated functions. Traditional feedforward methods for MAB converter control rely on precise lumped circuit models. This article presents a machine learning (ML) method for the feedforward power flow control of an MAB converter without a precise circuit model. A feedforward neural network was developed to capture the nonlinear characteristics and predict the phases needed to achieve the targeted power flow. The neural network was trained with a large amount of data, collected with a set of known phase angles. This trained network was used to predict the phases to achieve the targeted power flow. A six-port MAB converter was built and tested to validate the methodology and demonstrate the “machine-learning-in-the-loop” implementation. Transfer learning was proven to be effective in reducing the size of the training data needed to obtain an accurate ML model. ML-based feedforward power flow control can achieve comparable accuracy as traditional model-based methods and can function without a precise lumped circuit element model of the MAB converter.
更多查看译文
关键词
Artificial intelligence (AI),machine learning (ML),machine-learning-in-the-loop,multi-active-bridge (MAB) converter,neural network (NN),power flow control,transfer learning
AI 理解论文
溯源树
样例
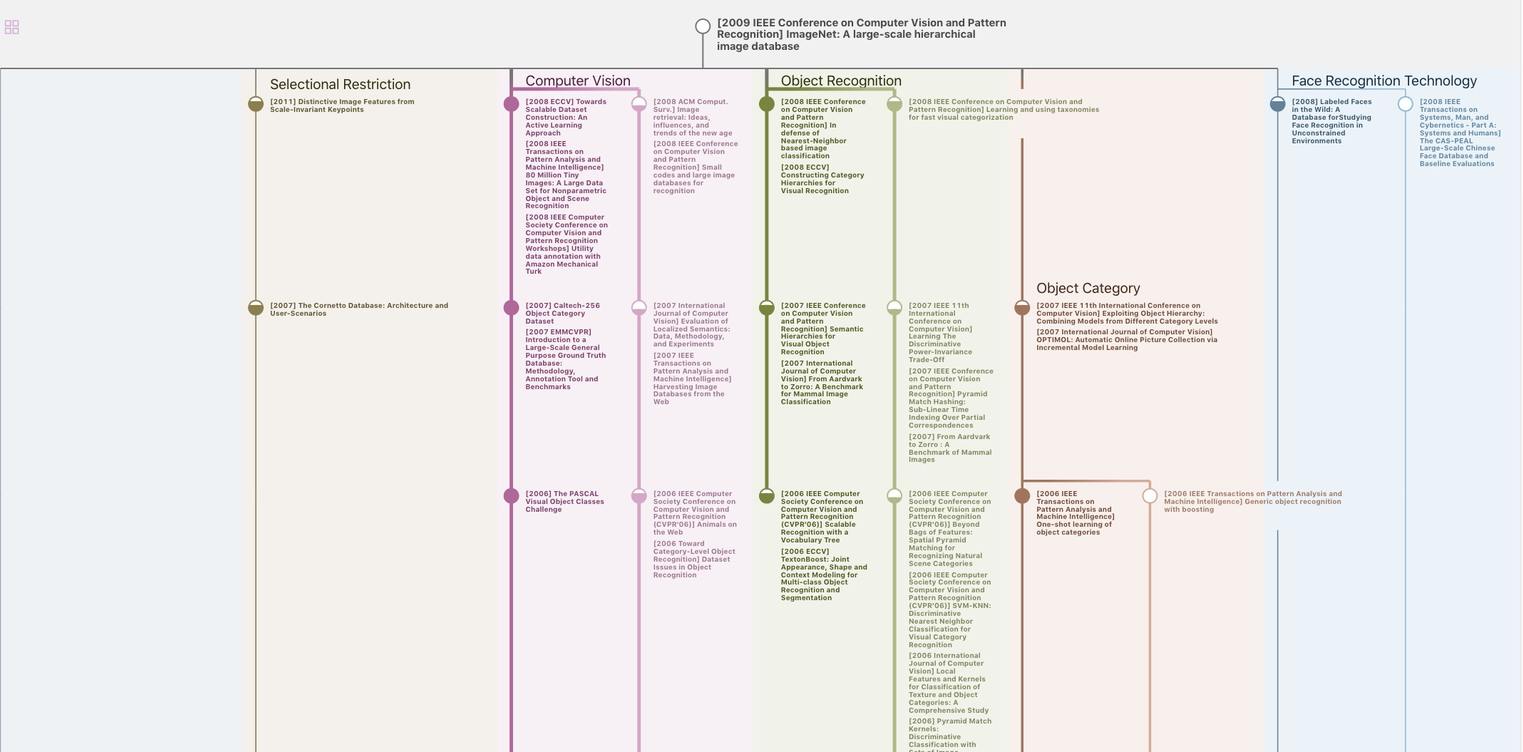
生成溯源树,研究论文发展脉络
Chat Paper
正在生成论文摘要