Deep Learning based Aerial Image Segmentation for Computing Green Area Factor
2022 10th European Workshop on Visual Information Processing (EUVIP)(2022)
摘要
The Green Area Factor(GYF) is an aggregate norm used as an index to quantify how much eco-efficient surface exists in a given area. Although the GYF is a single number, it expresses several different contributions of natural objects to the ecosystem. It is used as a planning tool to create and manage attractive urban environments ensuring the existence of required green/blue elements. Currently, the GYF model is gaining rapid attraction by different communities. However, calculating the GYF value is challenging as significant amount of manual effort is needed. In this study, we present a novel approach for automatic extraction of the GYF value from aerial imagery using semantic segmentation results. For model training and validation a set of RGB images captured by Drone imaging system is used. Each image is annotated into trees, grass, soil/open surface, building, and road. A modified U-net deep learning architecture is used for the segmentation of various objects by classifying each pixel into one of the semantic classes. From the segmented image we calculate the class-wise fractional area coverages that are used as input into the simplified GYF model called Sundbyberg for calculating the GYF value. Experimental results yield that the deep learning method provides about 92% mean IoU for test image segmentation and corresponding GYF value is 0.34.
更多查看译文
关键词
green Area index,deep learning,CNN,image,segmentation,urban planning,semantic classification
AI 理解论文
溯源树
样例
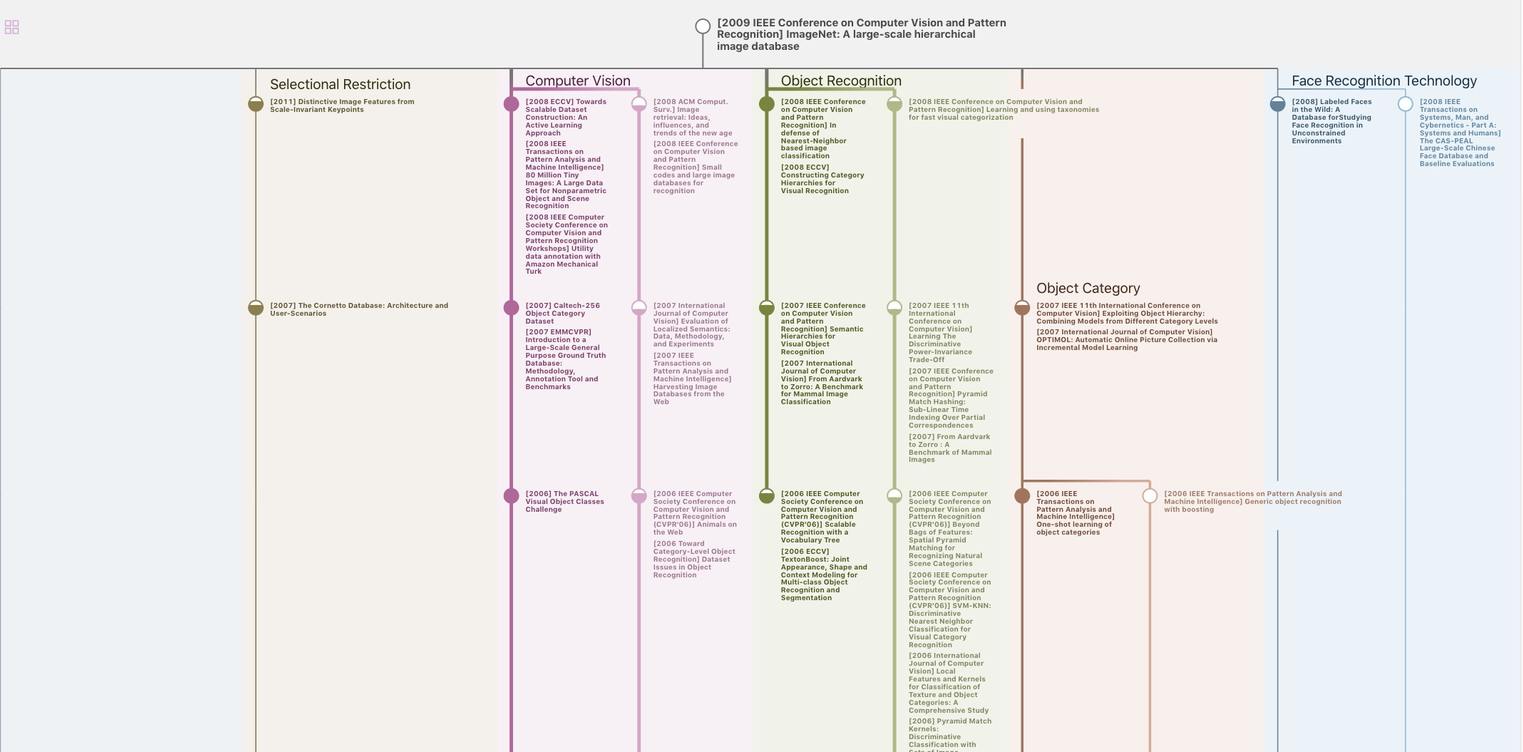
生成溯源树,研究论文发展脉络
Chat Paper
正在生成论文摘要