Enhanced Local Optimization Framework for Light Field Disparity Estimation
2022 10th European Workshop on Visual Information Processing (EUVIP)(2022)
摘要
Light Field depth estimation has become a very relevant topic over the last decade. With advances in augmented and virtual reality technologies, estimating the depth of a scene with accuracy became more and more important. One of the most successful families of algorithms in the field relies on minimising an objective function for all pixels by first computing the function for an arbitrary set of depth values. However, the choice of these values tends to constrain the accuracy of the disparity map. To overcome this problem, a local optimisation framework that enables accurate depth map estimation without requiring pre-selected depth values is proposed. The framework introduces a novel simulated annealing-based approach that takes advantage of neighbouring pixels to achieve good depth estimates in only 12 iterations, in the presented results. The framework is tested with three different state-of-the-art cost metrics, achieving an average reduction of 4.96 in Mean Squared Error ×100 and 5.53% in badpix 0.07 relative to the traditional approach.
更多查看译文
关键词
Light Field,Optimisation,Disparity,Depth
AI 理解论文
溯源树
样例
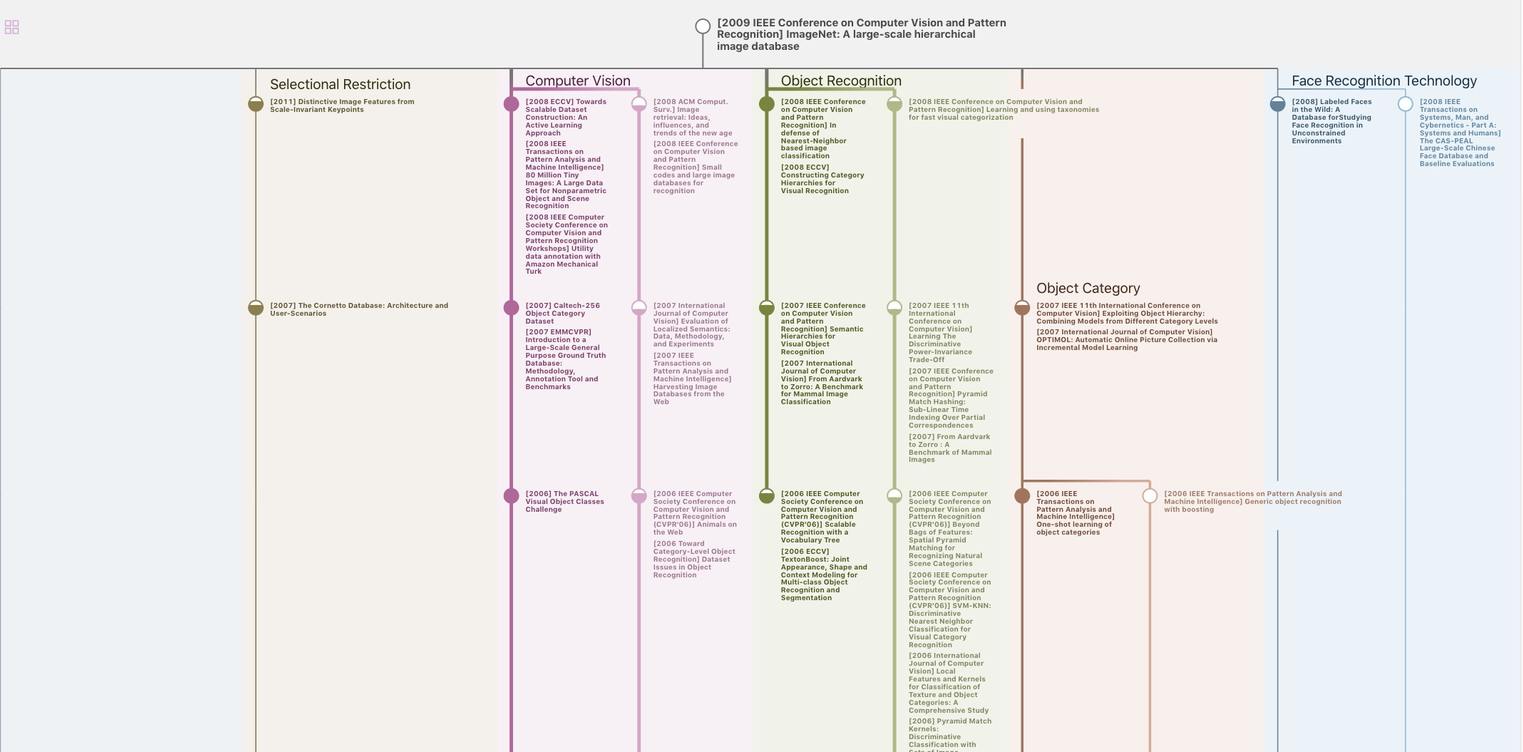
生成溯源树,研究论文发展脉络
Chat Paper
正在生成论文摘要