TempGNN: A Temperature-Based Graph Neural Network Model for System-Level Monitoring of Wind Turbines With SCADA Data
IEEE Sensors Journal(2022)
摘要
Accurate health monitoring and early fault warning are of critical importance to ensure the safe and reliable operation of wind turbines (WTs) and reduce operation and maintenance costs. For this reason, data-driven monitoring approaches have attracted considerable attention and have been widely studied. However, existing methods cannot well consider the interactions of different subsystems and components, thus often leading to missed detections and false alarms. To this end, we proposed a temperature-based graph neural network model named TempGNN to provide system-level monitoring for WTs with the available supervisory control and data acquisition (SCADA) data. TempGNN aims to automatically learn and capture the relationships between sensors from a graph structure data perspective. First, to eliminate the effects of time-varying operation conditions on temperature variables, a decoupled model is designed to obtain operation-independent temperature values. Then, an attention-based adaptive graph structure learning layer is designed to learn the weight matrix, and a spectral–temporal graph network block is introduced to capture spatial and temporal dependencies in graph data. Finally, anomaly detection can be performed through the calculation of health indicators defined with the residuals between the predicted outputs and the actual values. The effectiveness and robustness of the model were verified by two cases on a real SCADA dataset. The results show that our proposed TempGNN model can largely reduce false alarms and provide a reliable monitoring performance.
更多查看译文
关键词
Early fault detection,graph neural network (GNN),supervisory control and data acquisition (SCADA) data,temperature monitoring,wind turbine (WT)
AI 理解论文
溯源树
样例
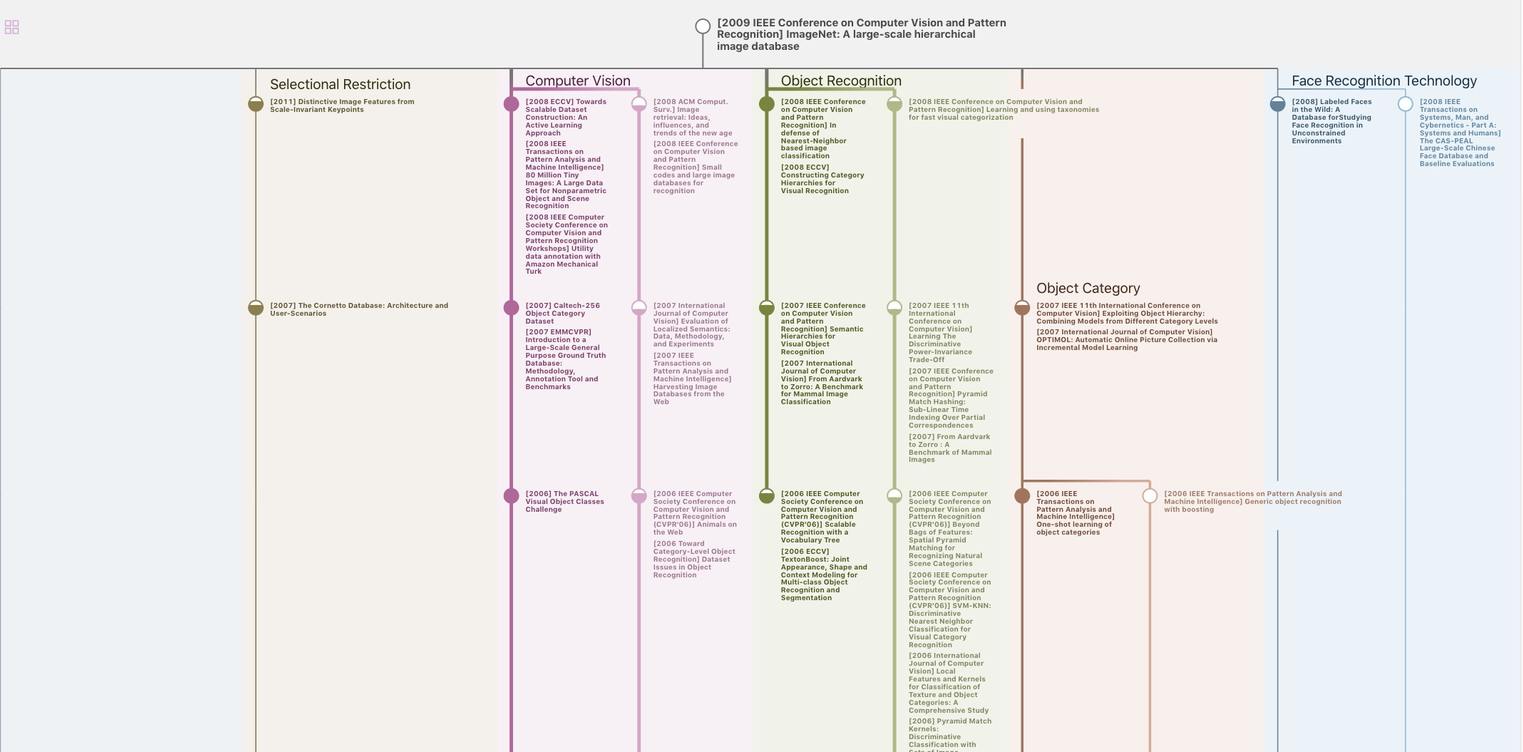
生成溯源树,研究论文发展脉络
Chat Paper
正在生成论文摘要