Causal Selection of Covariates in Regression Calibration for Mismeasured Continuous Exposure
arxiv(2022)
摘要
Regression calibration has been used to correct for the bias in causal effect due to measurement error in continuous exposures, but no systematic discussion exists on how to adjust for covariates in measurement error model (MEM) and outcome model used by the regression calibration method. In this paper, we investigate the methods for the selection of the minimal and most efficient covariate adjustment sets for regression calibration method developed by Rosner, Spiegelman and Willet (RSW, 1990) under a causal inference framework. We utilize directed acyclic graph to illustrate how subject matter knowledge can aid the selection of such adjustment sets. We show that in order to unbiasedly correct for measurement error, researchers need to collect data on any common causes (1)of true exposure and outcome and (2)of measurement error and outcome, in both main and validation study sample. Researchers need to adjust for this minimal covariate set in both MEM and outcome model. When such variable(s) are only available in the main study, researchers should still adjust for them in the outcome model to reduce bias, provided that these covariates are weakly associated with measurement error. We also show that adjusting for outcome risk factors that are independent of true exposure and measurement error in outcome model only may increase efficiency, while adjusting for any non-risk factors that are associated with true exposure almost always result in efficiency loss in realistic settings. Finally, we extend the originally proposed estimators to a non-parametric setting where effect modification by covariates is allowed.
更多查看译文
AI 理解论文
溯源树
样例
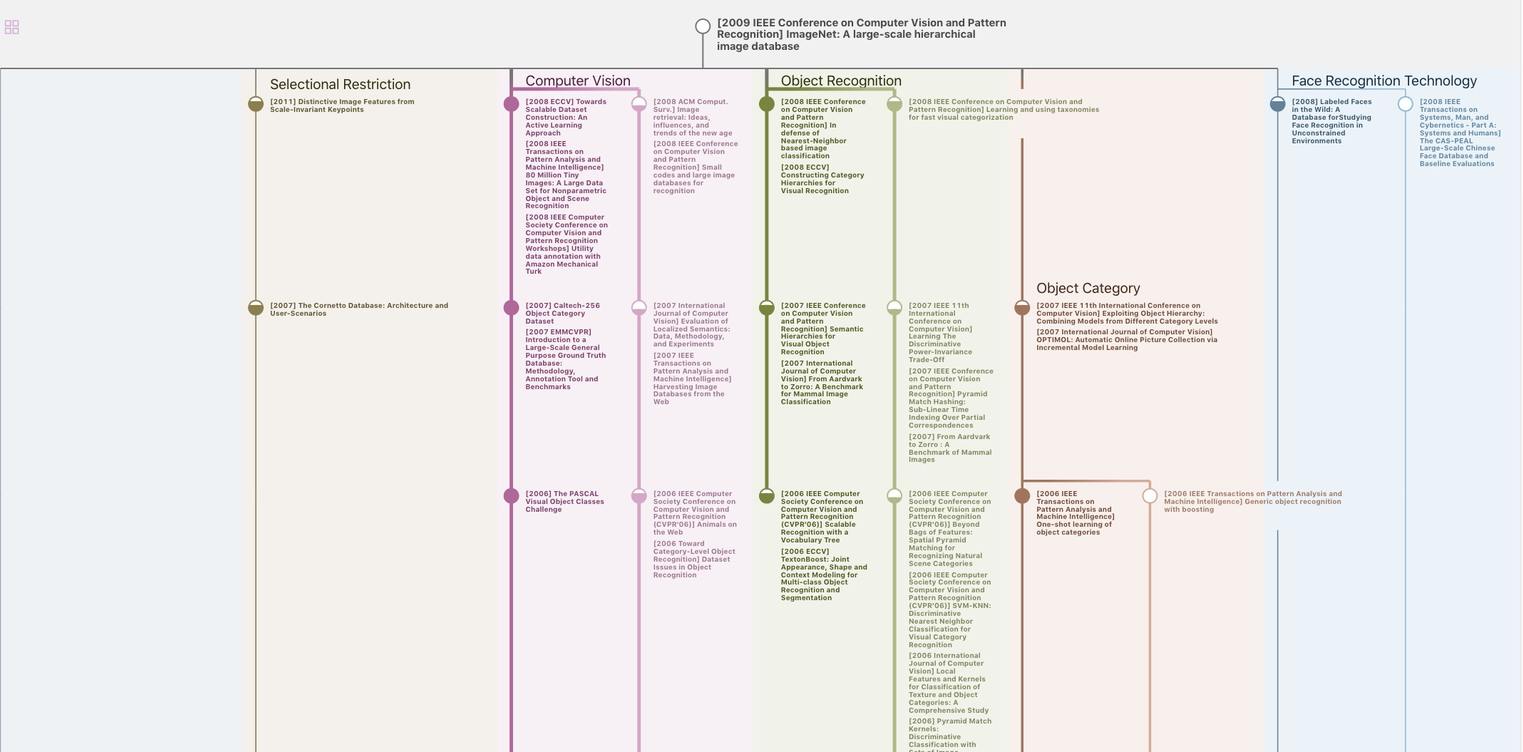
生成溯源树,研究论文发展脉络
Chat Paper
正在生成论文摘要