Data integration via analysis of subspaces (DIVAS)
TEST(2024)
摘要
Modern data collection in many data paradigms, including bioinformatics, often incorporates multiple traits derived from different data types (i.e., platforms). We call this data multi-block, multi-view, or multi-omics data. The emergent field of data integration develops and applies new methods for studying multi-block data and identifying how different data types relate and differ. One major frontier in contemporary data integration research is methodology that can identify partially shared structure between sub-collections of data types. This work presents a new approach: Data Integration Via Analysis of Subspaces (DIVAS). DIVAS combines new insights in angular subspace perturbation theory with recent developments in matrix signal processing and convex–concave optimization into one algorithm for exploring partially shared structure. Based on principal angles between subspaces, DIVAS provides built-in inference on the results of the analysis, and is effective even in high-dimension-low-sample-size (HDLSS) situations.
更多查看译文
关键词
Data integration,Matrix decomposition,Rotational bootstrap,Principal angle analysis,62H20
AI 理解论文
溯源树
样例
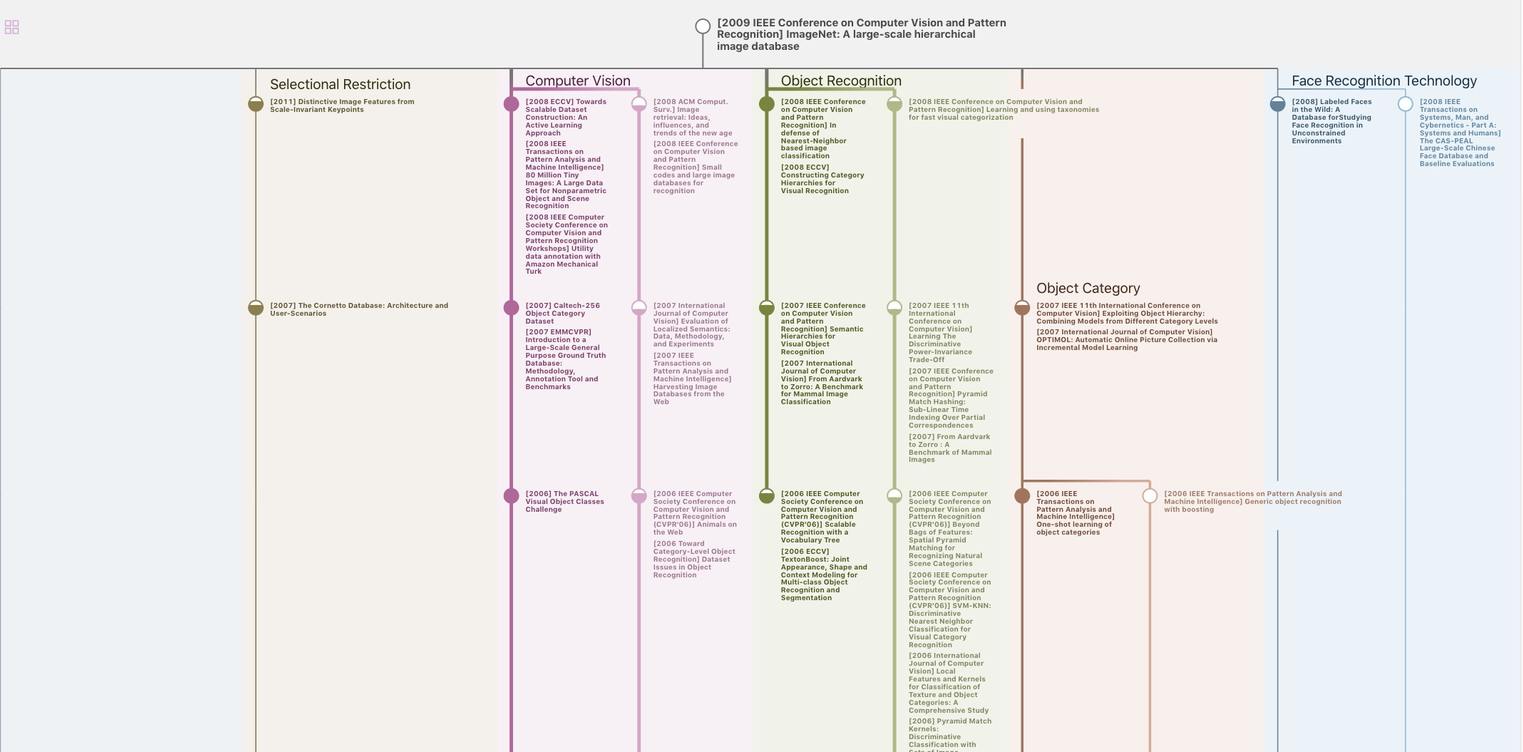
生成溯源树,研究论文发展脉络
Chat Paper
正在生成论文摘要