Implicit Mixture of Interpretable Experts for Global and Local Interpretability
arxiv(2022)
摘要
We investigate the feasibility of using mixtures of interpretable experts (MoIE) to build interpretable image classifiers on MNIST10. MoIE uses a black-box router to assign each input to one of many inherently interpretable experts, thereby providing insight into why a particular classification decision was made. We find that a naively trained MoIE will learn to 'cheat', whereby the black-box router will solve the classification problem by itself, with each expert simply learning a constant function for one particular class. We propose to solve this problem by introducing interpretable routers and training the black-box router's decisions to match the interpretable router. In addition, we propose a novel implicit parameterization scheme that allows us to build mixtures of arbitrary numbers of experts, allowing us to study how classification performance, local and global interpretability vary as the number of experts is increased. Our new model, dubbed Implicit Mixture of Interpretable Experts (IMoIE) can match state-of-the-art classification accuracy on MNIST10 while providing local interpretability, and can provide global interpretability albeit at the cost of reduced classification accuracy.
更多查看译文
关键词
interpretable experts,interpretability,local
AI 理解论文
溯源树
样例
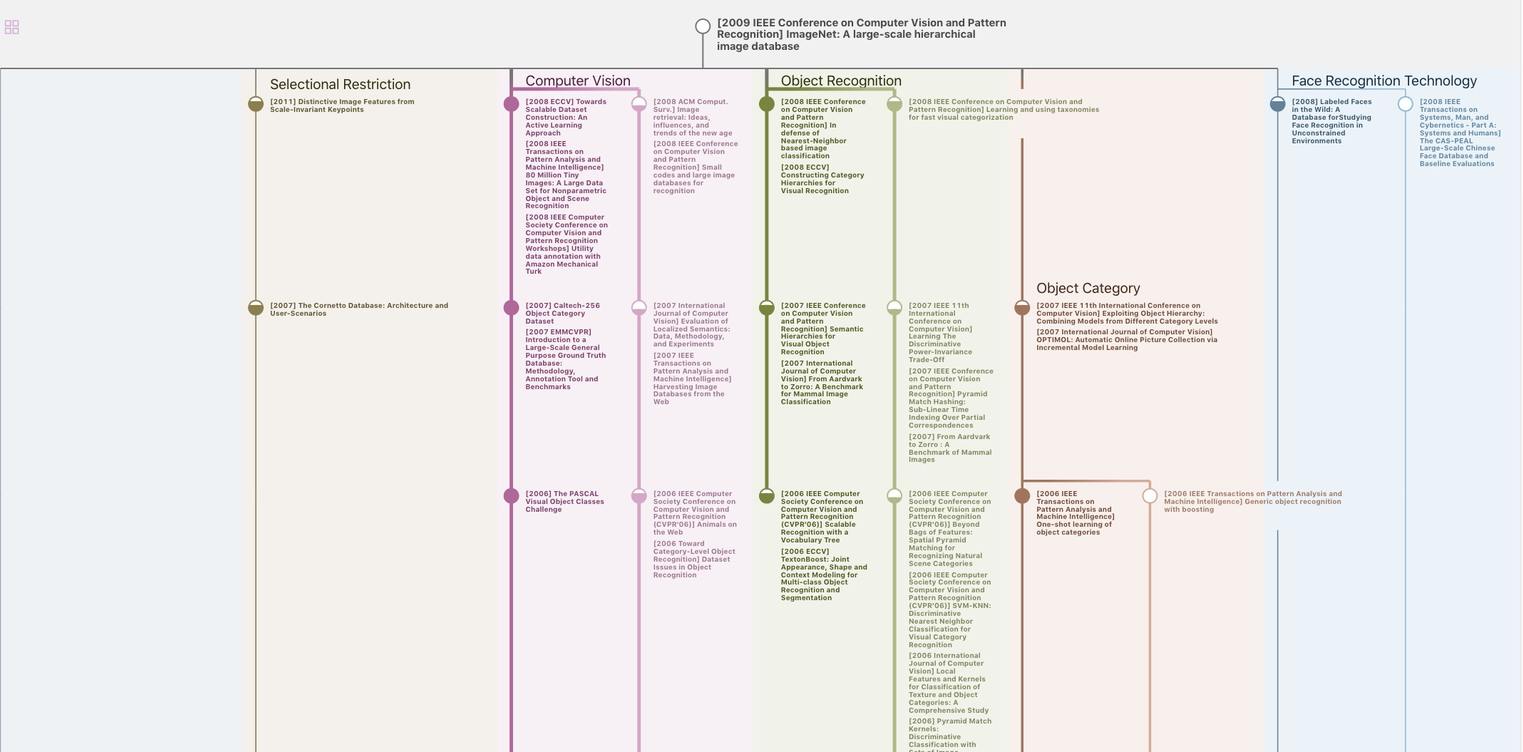
生成溯源树,研究论文发展脉络
Chat Paper
正在生成论文摘要