A preliminary attempt to use radiomic features in the diagnosis of extra-articular long head biceps tendinitis
Magma (New York, N.Y.)(2022)
摘要
Background This study aims to present a radiomic application in diagnosing the long head of biceps (LHB) tendinitis. Moreover, we evaluated whether machine learning-derived radiomic features recognize LHB tendinitis. Patients and methods A total of 170 patients were reviewed. All LHB tendinitis patients were diagnosed under arthroscopy. Radiomic features were extracted from preoperative magnetic resonance imaging (MRI), and the input dataset was divided into a training set and a test set. For feature selection, the t test and least absolute shrinkage and selection operator (LASSO) methods were used, and random forest (RF) and support vector machine (SVM) were used as machine learning classifiers. The sensitivity, specificity, accuracy, and area under the curve (AUC) of each model’s receiver operating characteristic (ROC) curves were calculated to evaluate model performance. Results In total, 851 radiomic features were extracted, with 109 radiomic features extracted using a t test and 20 radiomic features extracted using the LASSO method. The random forest classifier shows the highest sensitivity, specificity, accuracy, and AUC (0.52, 0.92, 0.73, and 0.72). Conclusion The classifier contract by 20 radiomic features demonstrated a good ability to predict extra-articular LHB tendinitis.However because of poor segmentation reliability, the value of Radiomic in LHB tendinitis still needs to be further explored.
更多查看译文
关键词
Long head of biceps tendon,MRI,Machine learning,Radiomic,Tendinitis
AI 理解论文
溯源树
样例
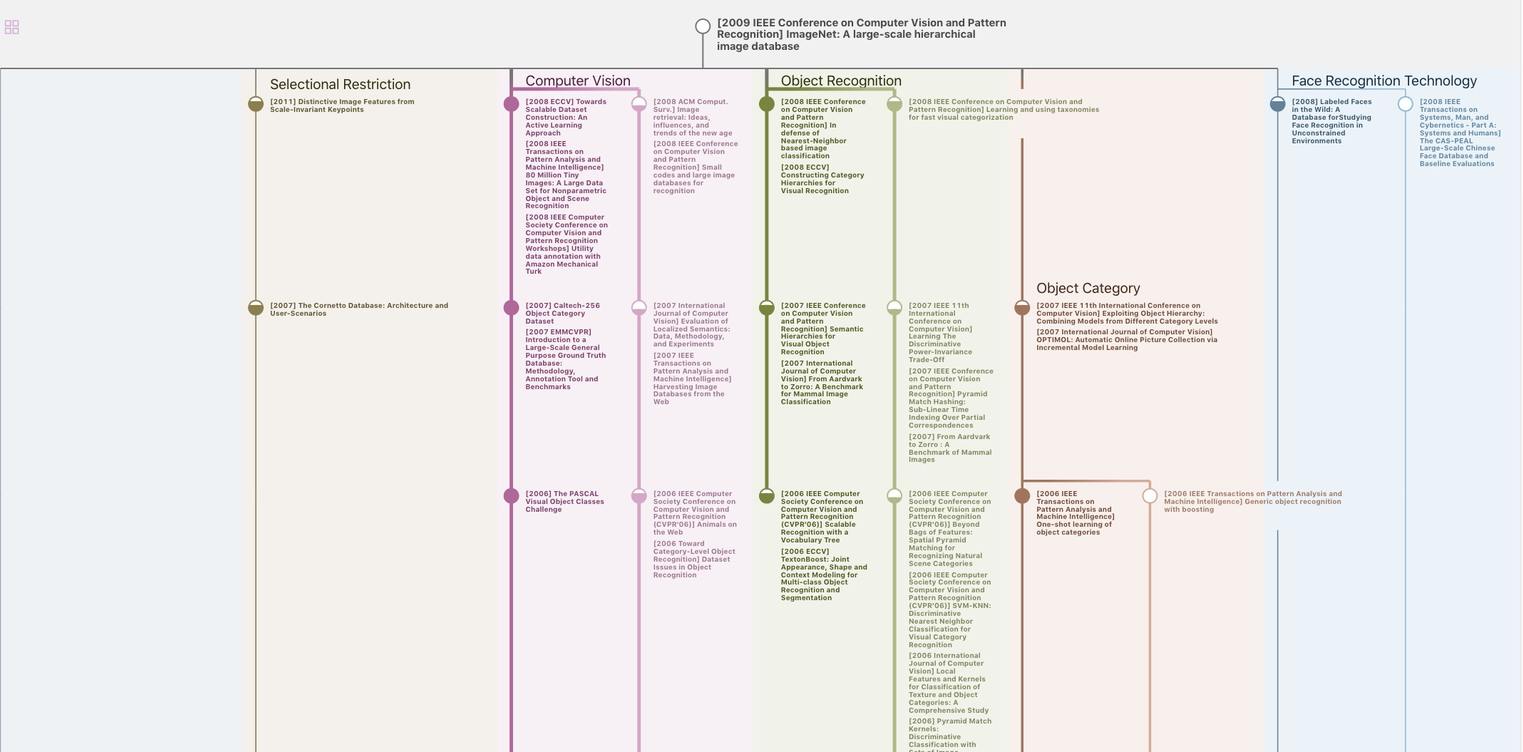
生成溯源树,研究论文发展脉络
Chat Paper
正在生成论文摘要