Jammer robust model-aided deep learning-based target detection for cognitive sonar
The Journal of the Acoustical Society of America(2022)
摘要
This work extends the development of an adaptive deep learning-based target detection algorithm for a channel matrix-based cognitive sonar framework that makes use of both a primary and secondary dataset. The channel matrix-based framework models all waveform dependent components, i.e. the sonar echo and clutter, via transfer function matrices, whereas all other waveform independent noise is characterized via the data covariance. By leveraging "lessons learned" from a derived model-based generalized likelihood ratio test (GLRT), a data driven deep learning algorithm was made adaptive to waveform dependent clutter returns in our previous work. The algorithm, however, remains fragile to waveform independent jamming and interference, and the extensive training required to obtain robust performance is practically infeasible. Thus, in this work further modifications are included to robustify the algorithm against these waveform independent components by leveraging knowledge from the model-based GLRT. The performance of the proposed algorithm is tested using various scenarios with jamming and interference signals.
更多查看译文
关键词
target detection,model-aided,learning-based
AI 理解论文
溯源树
样例
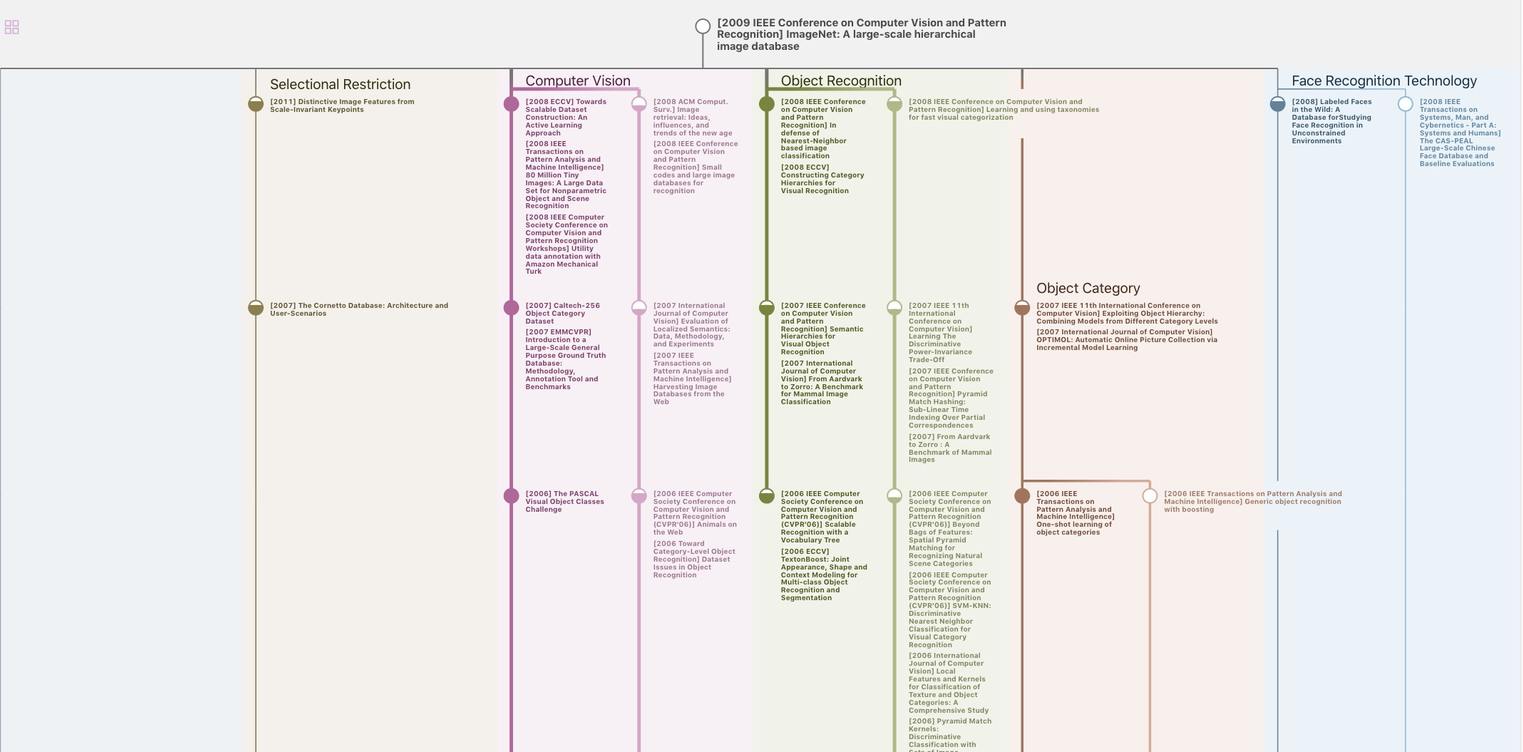
生成溯源树,研究论文发展脉络
Chat Paper
正在生成论文摘要