A biology-inspired recurrent oscillator network for computations in high-dimensional state space
biorxiv(2022)
摘要
Biological neuronal networks have the propensity to oscillate. However, it is unclear whether these oscillations are a mere byproduct of neuronal interactions or serve computational purposes. Therefore, we implemented hallmark features of the cerebral cortex in recurrent neuronal networks (RNNs) simulated in silico and examined their performance on common pattern recognition tasks after training with a gradient-based learning rule. We find that by configuring network nodes as damped harmonic oscillators (DHOs), performance is substantially improved over non-oscillating architectures and that the introduction of heterogeneous nodes, conduction delays, and network modularity further improved performance. We furthermore provide a proof of concept of how unsupervised Hebbian learning can work in such networks. Analyses of network activity illustrate how the nonlinear dynamics of coupled DHOs drive performance, and provide plausible a posteriori explanations for a number of physiological phenomena whose function so far has been elusive.
### Competing Interest Statement
The authors have declared no competing interest.
更多查看译文
AI 理解论文
溯源树
样例
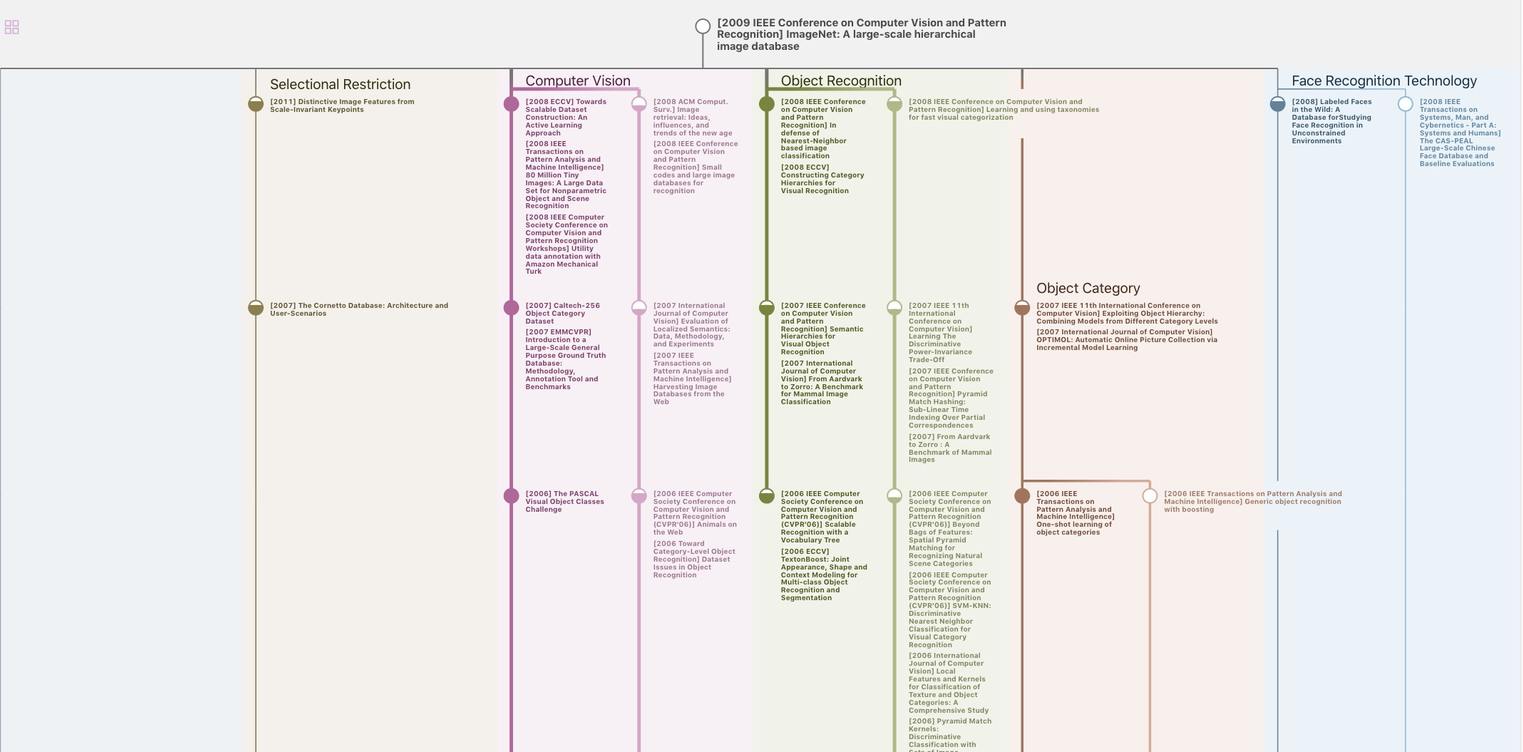
生成溯源树,研究论文发展脉络
Chat Paper
正在生成论文摘要