LocPoseNet: Robust Location Prior for Unseen Object Pose Estimation
arxiv(2023)
摘要
Object location priors have been shown to be critical for the standard 6D object pose estimation setting, where the training and testing objects are the same. Specifically, they can be used to initialize the 3D object translation and facilitate 3D object rotation estimation. Unfortunately, the object detectors that are used for this purpose do not generalize to unseen objects, i.e., objects from new categories at test time. Therefore, existing 6D pose estimation methods for previously-unseen objects either assume the ground-truth object location to be known, or yield inaccurate results when it is unavailable. In this paper, we address this problem by developing a method, LocPoseNet, able to robustly learn location prior for unseen objects. Our method builds upon a template matching strategy, where we propose to distribute the reference kernels and convolve them with a query to efficiently compute multi-scale correlations. We then introduce a novel translation estimator, which decouples scale-aware and scale-robust features to predict different object location parameters. Our method outperforms existing works by a large margin on LINEMOD and GenMOP. We further construct a challenging synthetic dataset, which allows us to highlight the better robustness of our method to various noise sources.
更多查看译文
关键词
unseen object locposenet estimation,robust location prior
AI 理解论文
溯源树
样例
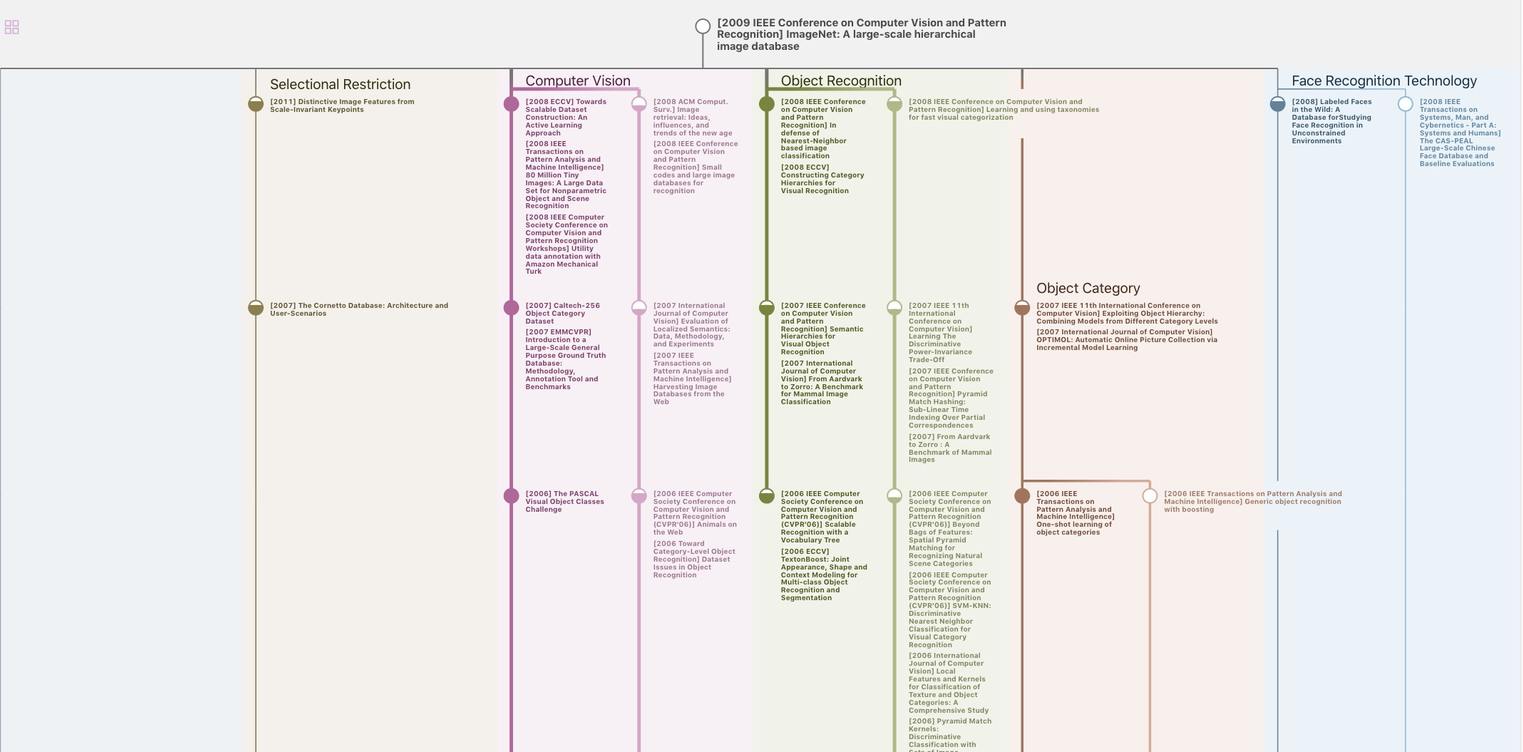
生成溯源树,研究论文发展脉络
Chat Paper
正在生成论文摘要