ResNeRF: Geometry-Guided Residual Neural Radiance Field for Indoor Scene Novel View Synthesis
arxiv(2022)
摘要
We represent the ResNeRF, a novel geometry-guided two-stage framework for indoor scene novel view synthesis. Be aware of that a good geometry would greatly boost the performance of novel view synthesis, and to avoid the geometry ambiguity issue, we propose to characterize the density distribution of the scene based on a base density estimated from scene geometry and a residual density parameterized by the geometry. In the first stage, we focus on geometry reconstruction based on SDF representation, which would lead to a good geometry surface of the scene and also a sharp density. In the second stage, the residual density is learned based on the SDF learned in the first stage for encoding more details about the appearance. In this way, our method can better learn the density distribution with the geometry prior for high-fidelity novel view synthesis while preserving the 3D structures. Experiments on large-scale indoor scenes with many less-observed and textureless areas show that with the good 3D surface, our method achieves state-of-the-art performance for novel view synthesis.
更多查看译文
关键词
residual neural radiance field,novel view synthesis,indoor scene
AI 理解论文
溯源树
样例
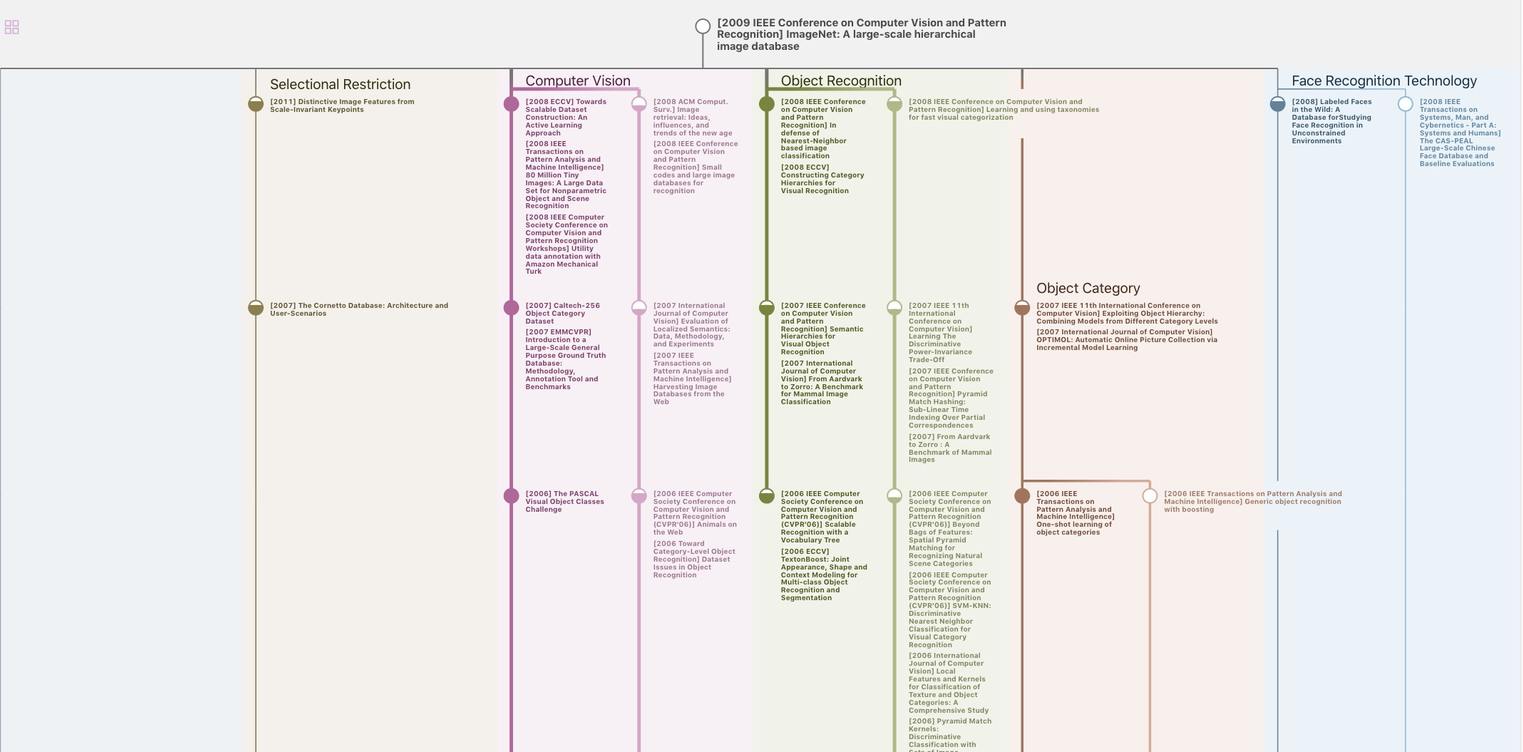
生成溯源树,研究论文发展脉络
Chat Paper
正在生成论文摘要