Fingerprinting and analysis of Bluetooth devices with automata learning
arxiv(2022)
摘要
Automata learning is a technique to automatically infer behavioral models of black-box systems. Today’s learning algorithms enable the deduction of models that describe complex system properties, e.g., timed or stochastic behavior. Despite recent improvements in the scalability of learning algorithms, their practical applicability is still an open issue. Little work exists that actually learns models of physical black-box systems. To fill this gap in the literature, we present a case study on applying automata learning on the Bluetooth Low Energy (BLE) protocol. It shows that not only the size of the system limits the applicability of automata learning. Also, the interaction with the system under learning creates a major bottleneck that is rarely discussed. In this article, we propose a general automata learning architecture for learning a behavioral model of the BLE protocol implemented by a physical device. With this framework, we can successfully learn the behavior of six investigated BLE devices. Furthermore, we extended the learning technique to learn security critical behavior, e.g., key-exchange procedures for encrypted communication. The learned models depict several behavioral differences and inconsistencies to the BLE specification. This shows that automata learning can be used for fingerprinting black-box devices, i.e., characterizing systems via their specific learned models. Moreover, learning revealed a crashing scenario for one device.
更多查看译文
关键词
Active automata learning,Model inference,Learning-based testing,Fingerprinting,Bluetooth Low Energy,IoT
AI 理解论文
溯源树
样例
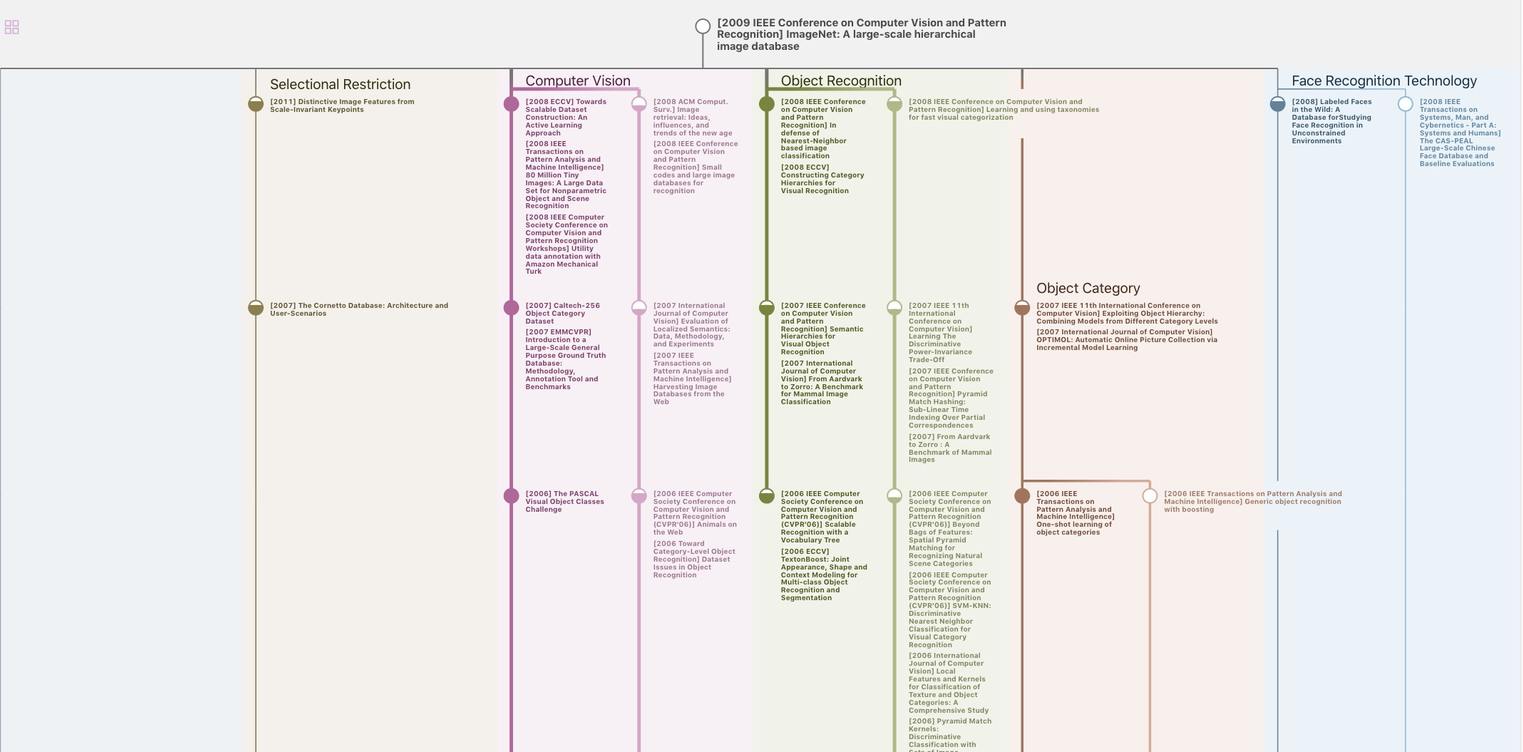
生成溯源树,研究论文发展脉络
Chat Paper
正在生成论文摘要