Linear Complexity Gibbs Sampling for Generalized Labeled Multi-Bernoulli Filtering
arxiv(2023)
摘要
Generalized Labeled Multi-Bernoulli (GLMB) densities arise in a host of multi-object system applications analogous to Gaussians in single-object filtering. However, computing the GLMB filtering density requires solving NP-hard problems. To alleviate this computational bottleneck, we develop a linear complexity Gibbs sampling framework for GLMB density computation. Specifically, we propose a tempered Gibbs sampler that exploits the structure of the GLMB filtering density to achieve an O(T (P + M)) complexity, where T is the number of iterations of the algorithm, P and M are the number hypothesized objects and measurements. This innovation enables the GLMB filter implementation to be reduced from an O ((TPM)-M-2) complexity to O (T (P + M + log T ) + PM). Moreover, the proposed framework provides the flexibility for trade-offs between tracking performance and computational load. Convergence of the proposed Gibbs sampler is established, and numerical studies are presented to validate the proposed GLMB filter implementation.
更多查看译文
关键词
Random finite sets,multi-object tracking,generalized labeled multi-Bernoulli,tempered Gibbs sampling
AI 理解论文
溯源树
样例
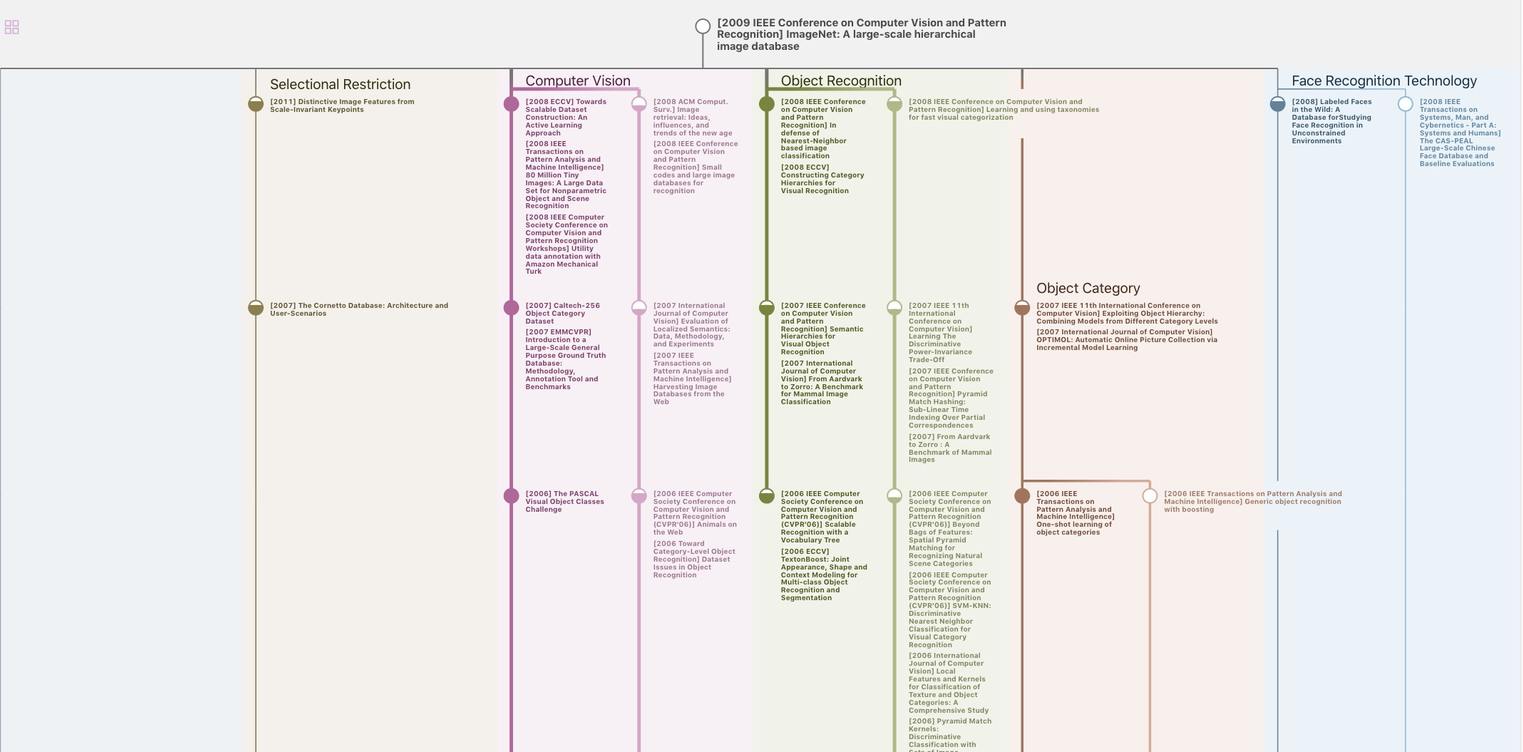
生成溯源树,研究论文发展脉络
Chat Paper
正在生成论文摘要