Learning deep abdominal CT registration through adaptive loss weighting and synthetic data generation
arxiv(2023)
摘要
PurposeThis study aims to explore training strategies to improve convolutional neural network-based image-to-image deformable registration for abdominal imaging. MethodsDifferent training strategies, loss functions, and transfer learning schemes were considered. Furthermore, an augmentation layer which generates artificial training image pairs on-the-fly was proposed, in addition to a loss layer that enables dynamic loss weighting. ResultsGuiding registration using segmentations in the training step proved beneficial for deep-learning-based image registration. Finetuning the pretrained model from the brain MRI dataset to the abdominal CT dataset further improved performance on the latter application, removing the need for a large dataset to yield satisfactory performance. Dynamic loss weighting also marginally improved performance, all without impacting inference runtime. ConclusionUsing simple concepts, we improved the performance of a commonly used deep image registration architecture, VoxelMorph. In future work, our framework, DDMR, should be validated on different datasets to further assess its value.
更多查看译文
关键词
deep abdominal ct registration,adaptive loss weighting
AI 理解论文
溯源树
样例
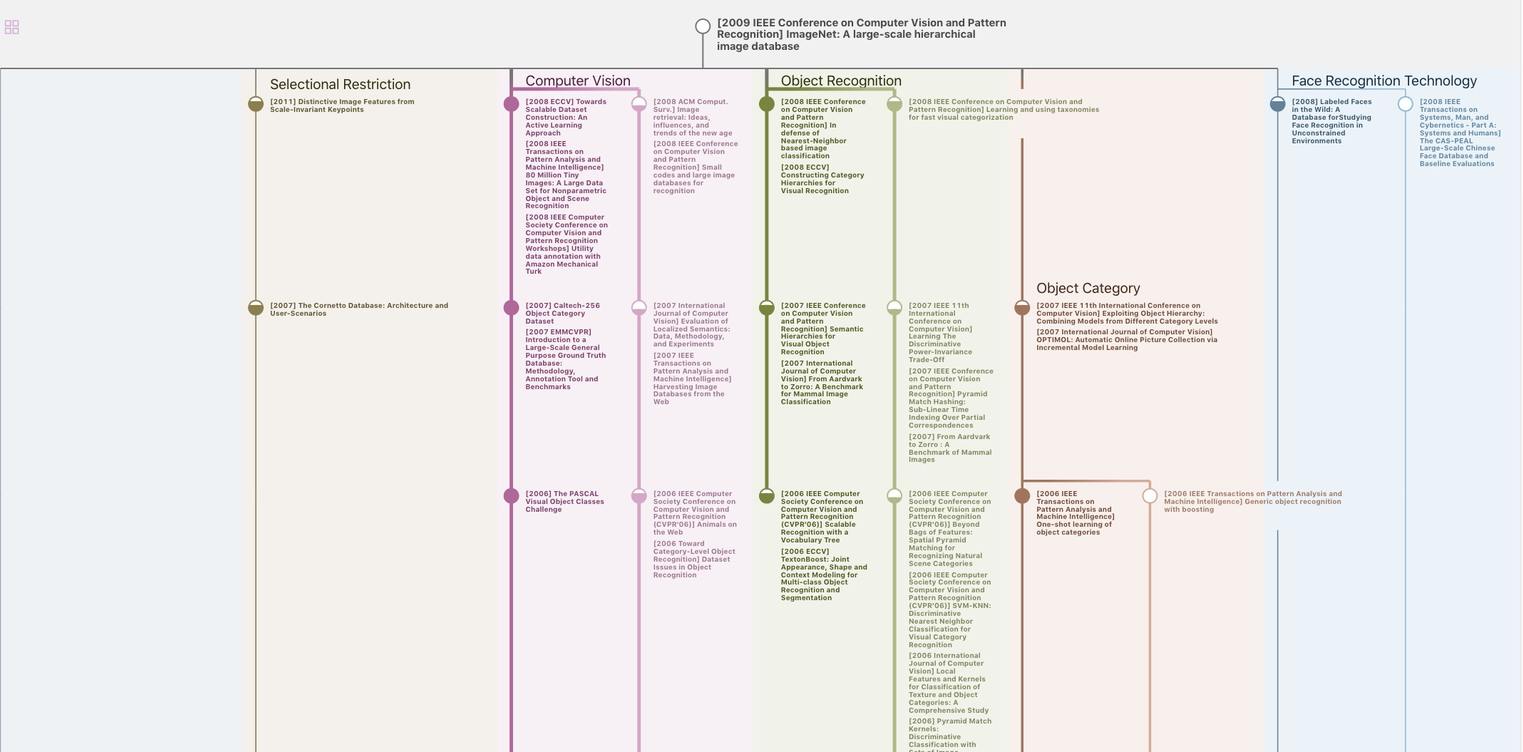
生成溯源树,研究论文发展脉络
Chat Paper
正在生成论文摘要