Discovering Dynamic Patterns From Spatiotemporal Data With Time-Varying Low-Rank Autoregression
IEEE TRANSACTIONS ON KNOWLEDGE AND DATA ENGINEERING(2024)
摘要
The problem of discovering interpretable dynamic patterns from spatiotemporal data is studied in this paper. For that purpose, we develop a time-varying reduced-rank vector autoregression (VAR) model whose coefficient matrices are parameterized by low-rank tensor factorization. Benefiting from the tensor factorization structure, the proposed model can simultaneously achieve model compression and pattern discovery. In particular, the proposed model allows one to characterize nonstationarity and time-varying system behaviors underlying spatiotemporal data. To evaluate the proposed model, extensive experiments are conducted on various spatiotemporal datasets representing different nonlinear dynamical systems, including fluid dynamics, sea surface temperature, USA surface temperature, and NYC taxi trips. Experimental results demonstrate the effectiveness of the proposed model for analyzing spatiotemporal data and characterizing spatial/temporal patterns. In the spatial context, the spatial patterns can be automatically extracted and intuitively characterized by the spatial modes. In the temporal context, the complex time-varying system behaviors can be revealed by the temporal modes in the proposed model. Thus, our model lays an insightful foundation for understanding complex spatiotemporal data in real-world dynamical systems.
更多查看译文
关键词
Pattern discovery,spatiotemporal data,tensor factorization,time-varying system,vector autoregression
AI 理解论文
溯源树
样例
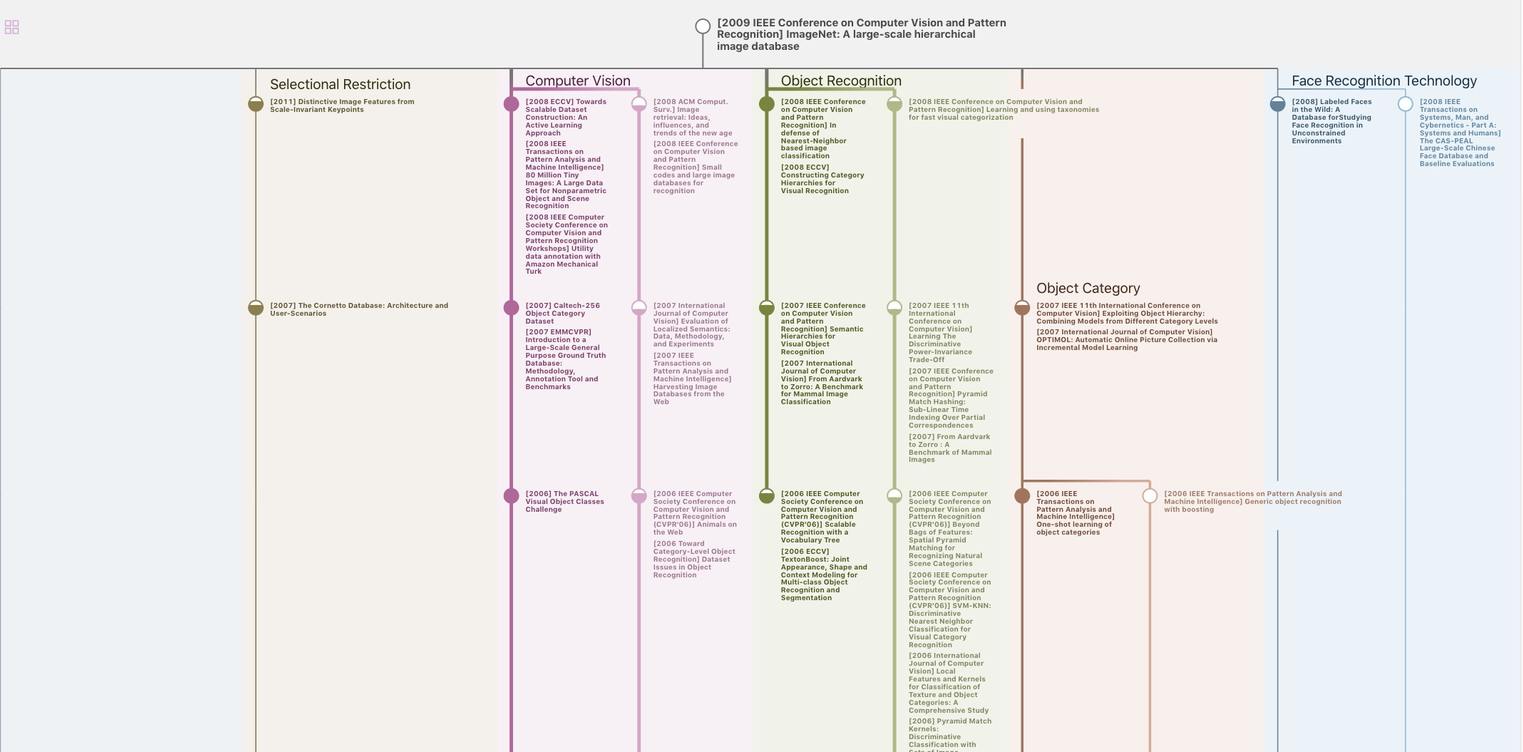
生成溯源树,研究论文发展脉络
Chat Paper
正在生成论文摘要