Viewport-Sphere-Branch Network for Blind Quality Assessment of Stitched 360 Omnidirectional Images
IEEE Transactions on Circuits and Systems for Video Technology(2023)
摘要
Compared with conventional images/videos, omnidirectional data records rich information with higher resolution and wider Field-of-View. Moreover, the stitching distortions introduced in the panoramic content generation process make the quality assessment task more challenging. Targeting at designing an accurate and fast stitched 360 degrees omnidirectional image quality evaluator, we propose a Viewport-Sphere-Branch Network (VSBNet) via dual-branch quality estimation. Specifically, for the viewport quality estimation, we extract distorted viewports around the stitching seams and conduct distortion rectification through a progressively complementary network to obtain pseudo-reference viewports. The qualitative and quantitative experiments validate that pseudo-reference viewports are reliable. Then, the differences between distorted and pseudo-reference viewports are quantified through transformer architecture to obtain quality scores of viewports. The introduction of pseudo-reference viewports can effectively improve the performance of the viewport quality prediction branch. To establish general scenario awareness and accurately evaluate the immersive experience, we extract feature representation through deformable convolutions to eliminate 2D-to-Sphere intrinsic sampling distortions and use multilayer perceptron to predict score of the whole sphere. The final prediction score is obtained by aggregating the quality scores from viewport and sphere branches. We evaluate the proposed VSBNet on two benchmark databases and results demonstrate that the combination of two branches can obtain more accurate results. Overall, our method is superior to existing full reference and no reference models designed for conventional images and 360 degrees omnidirectional images.
更多查看译文
关键词
Stitched image,blind image quality assessment,deformable convolutional networks,distortion rectification
AI 理解论文
溯源树
样例
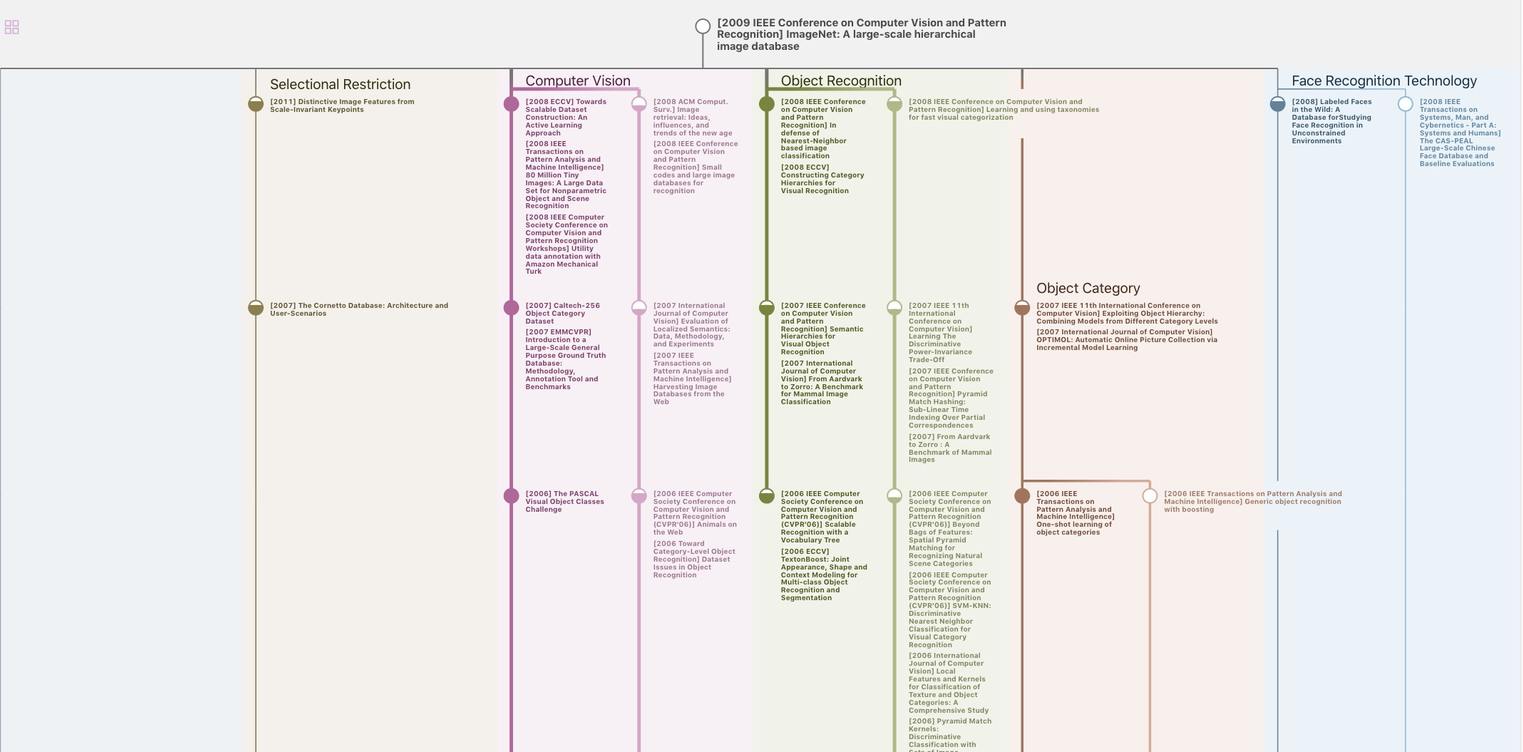
生成溯源树,研究论文发展脉络
Chat Paper
正在生成论文摘要