Computing and Communication Cost-Aware Service Migration Enabled by Transfer Reinforcement Learning for Dynamic Vehicular Edge Computing Networks
IEEE TRANSACTIONS ON MOBILE COMPUTING(2024)
摘要
Due to the high mobility of vehicles, service migration is inevitable in vehicular edge computing (VEC) networks. Frequent service migrations incur prohibitive migration cost including the computing cost (e.g., increased computing delay) and communication cost (e.g., occupied backhaul bandwidth). Yet existing service migration schemes are usually designed without considering the impact of the computing cost. This paper considers the impact of computing and communication cost jointly, and proposes a computing and communication cost-aware service migration scheme for VEC networks (i.e., CA-migration). Taking the service delay as a QoS metric for VEC networks, this paper formulates a migration optimization problem aiming to maximize the services' satisfaction degree of delay (i.e., the probability that the service delay is smaller than the service delay requirement), where both the communication cost and computing cost affect the services' satisfaction degree. Since the optimization problem is a constrained non-linear integer programming problem, it is difficult to solve. Moreover, the VEC networks are highly dynamic. Thus, a fast transfer reinforcement learning (fast-TRL) method combining transfer learning and reinforcement learning is proposed to provide an adaptive service migration scheme in dynamic VEC networks. Simulation results show that compared with existing schemes, the proposed CA-migration scheme can increase the satisfaction degree by up to 30%, and needs 25% less training time to obtain the optimal service migration policy.
更多查看译文
关键词
Vehicular edge computing,service migration,computing cost,services' satisfaction degree,fast transfer reinforcement learning
AI 理解论文
溯源树
样例
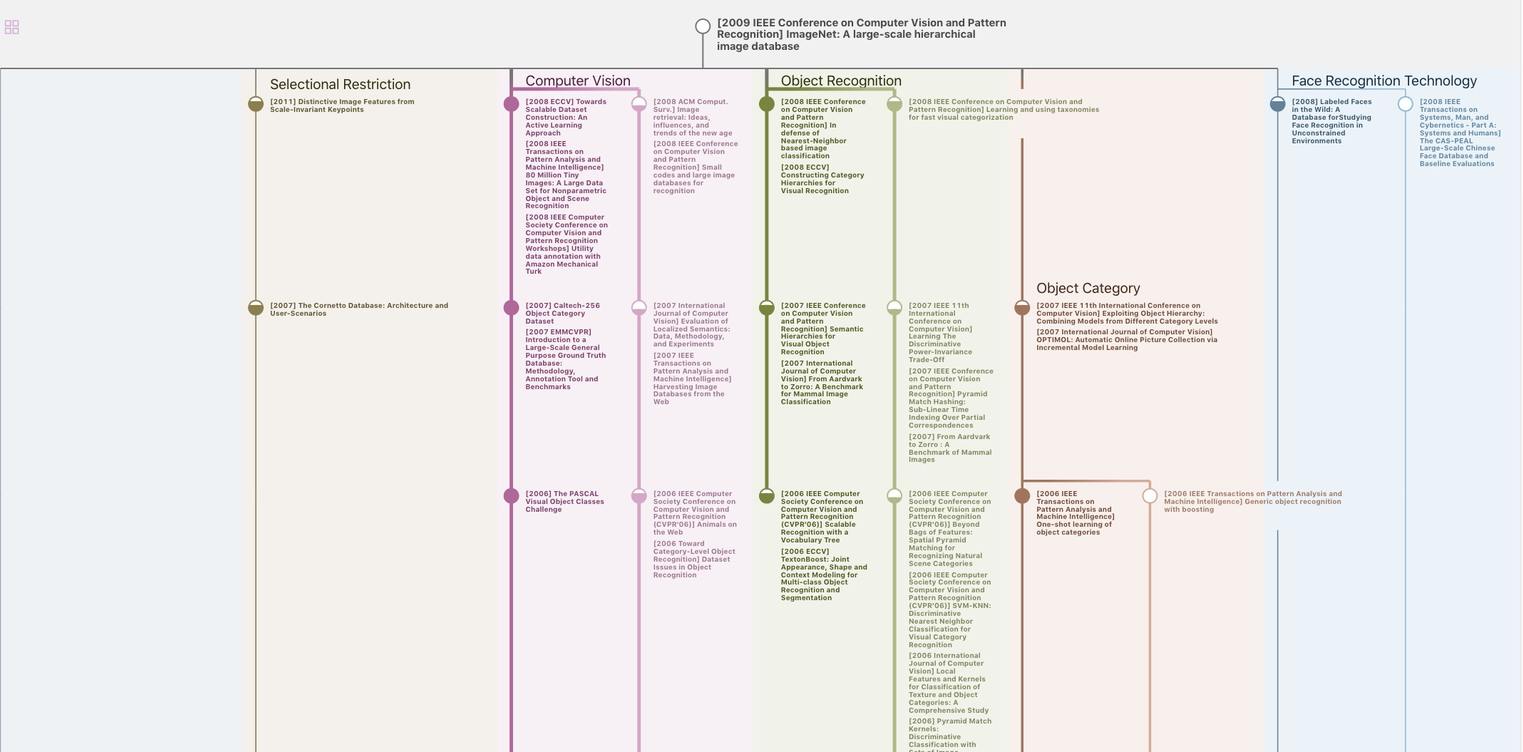
生成溯源树,研究论文发展脉络
Chat Paper
正在生成论文摘要