Predicting Hospital Readmissions in a Commercially Insured Population over Varying Time Horizons
JOURNAL OF GENERAL INTERNAL MEDICINE(2023)
摘要
Background Reducing hospital readmissions is a federal policy priority, and predictive models of hospital readmissions have proliferated in recent years; however, most such models tend to focus on the 30-day readmission time horizon and do not consider readmission over shorter (or longer) windows. Objectives To evaluate the performance of a predictive model of hospital readmissions over three different readmission timeframes in a commercially insured population. Design Retrospective multivariate logistic regression with an 80/20 train/test split. Participants A total of 2,213,832 commercially insured inpatient admissions from 2016 to 2017 comprising 782,768 unique patients from the Health Care Cost Institute. Main Measures Outcomes are readmission within 14 days, 15–30 days, and 31–60 days from discharge. Predictor variables span six different domains: index admission, condition history, demographic, utilization history, pharmacy, and environmental controls. Key Results Our model generates C-statistics for holdout samples ranging from 0.618 to 0.915. The model’s discriminative power declines with readmission time horizon: discrimination for readmission predictions within 14 days following discharge is higher than for readmissions 15–30 days following discharge, which in turn is higher than predictions 31–60 days following discharge. Additionally, the model’s predictive power increases nonlinearly with the inclusion of successive risk factor domains: patient-level measures of utilization and condition history add substantially to the discriminative power of the model, while demographic information, pharmacy utilization, and environmental risk factors add relatively little. Conclusion It is more difficult to predict distant readmissions than proximal readmissions, and the more information the model uses, the better the predictions. Inclusion of utilization-based risk factors add substantially to the discriminative ability of the model, much more than any other included risk factor domain. Our best-performing models perform well relative to other published readmission prediction models. It is possible that these predictions could have operational utility in targeting readmission prevention interventions among high-risk individuals.
更多查看译文
关键词
readmission,prediction,hospital quality
AI 理解论文
溯源树
样例
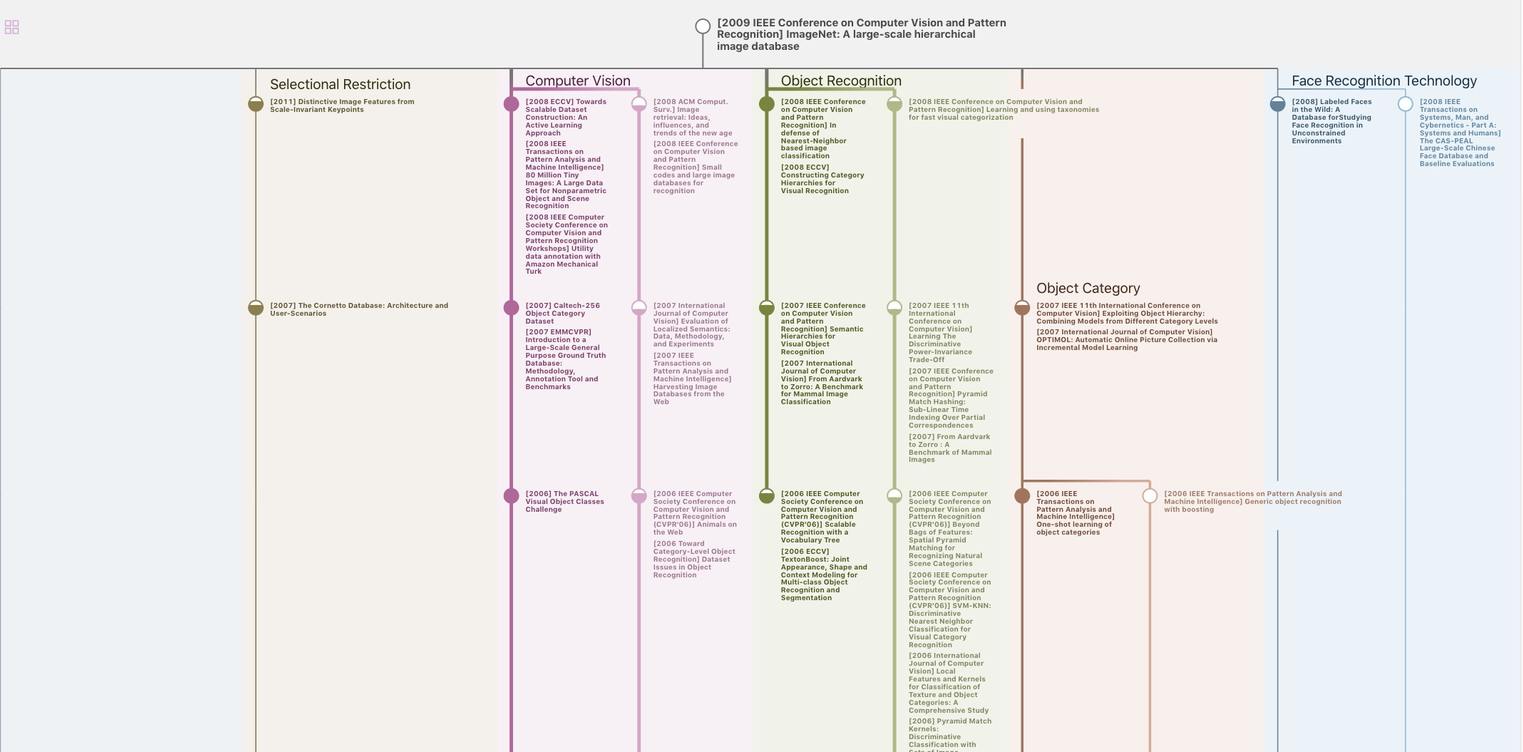
生成溯源树,研究论文发展脉络
Chat Paper
正在生成论文摘要