RbA: Segmenting Unknown Regions Rejected by All
arxiv(2023)
摘要
Standard semantic segmentation models owe their success to curated datasets with a fixed set of semantic categories, without contemplating the possibility of identifying unknown objects from novel categories. Existing methods in outlier detection suffer from a lack of smoothness and objectness in their predictions, due to limitations of the per-pixel classification paradigm. Furthermore, additional training for detecting outliers harms the performance of known classes. In this paper, we explore another paradigm with region-level classification to better segment unknown objects. We show that the object queries in mask classification tend to behave like one \vs all classifiers. Based on this finding, we propose a novel outlier scoring function called RbA by defining the event of being an outlier as being rejected by all known classes. Our extensive experiments show that mask classification improves the performance of the existing outlier detection methods, and the best results are achieved with the proposed RbA. We also propose an objective to optimize RbA using minimal outlier supervision. Further fine-tuning with outliers improves the unknown performance, and unlike previous methods, it does not degrade the inlier performance.
更多查看译文
关键词
unknown regions
AI 理解论文
溯源树
样例
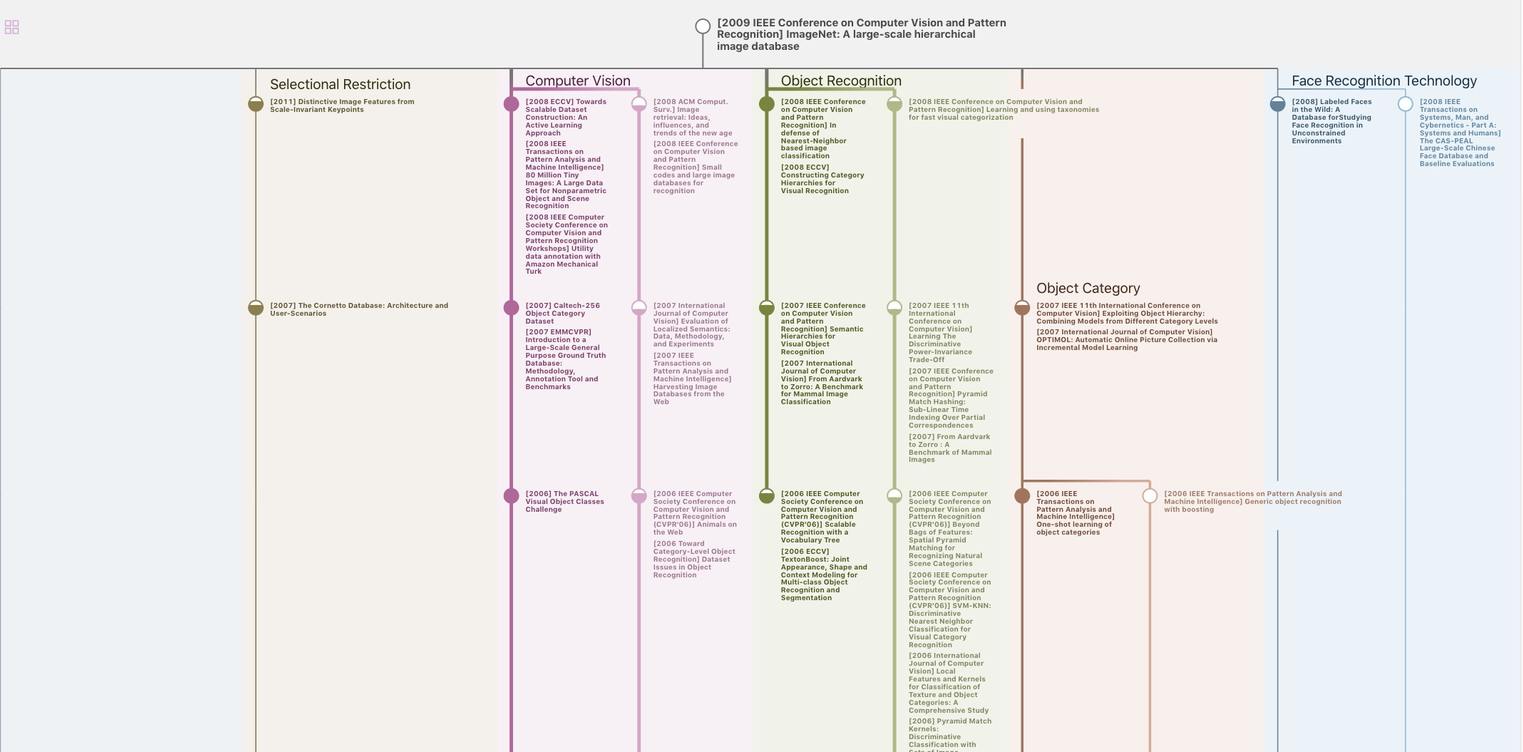
生成溯源树,研究论文发展脉络
Chat Paper
正在生成论文摘要