MUSIED: A Benchmark for Event Detection from Multi-Source Heterogeneous Informal Texts
emnlp 2022(2022)
摘要
Event detection (ED) identifies and classifies event triggers from unstructured texts, serving as a fundamental task for information extraction. Despite the remarkable progress achieved in the past several years, most research efforts focus on detecting events from formal texts (e.g., news articles, Wikipedia documents, financial announcements). Moreover, the texts in each dataset are either from a single source or multiple yet relatively homogeneous sources. With massive amounts of user-generated text accumulating on the Web and inside enterprises, identifying meaningful events in these informal texts, usually from multiple heterogeneous sources, has become a problem of significant practical value. As a pioneering exploration that expands event detection to the scenarios involving informal and heterogeneous texts, we propose a new large-scale Chinese event detection dataset based on user reviews, text conversations, and phone conversations in a leading e-commerce platform for food service. We carefully investigate the proposed dataset's textual informality and multi-source heterogeneity characteristics by inspecting data samples quantitatively and qualitatively. Extensive experiments with state-of-the-art event detection methods verify the unique challenges posed by these characteristics, indicating that multi-source informal event detection remains an open problem and requires further efforts. Our benchmark and code are released at \url{https://github.com/myeclipse/MUSIED}.
更多查看译文
关键词
event detection,texts,benchmark,multi-source
AI 理解论文
溯源树
样例
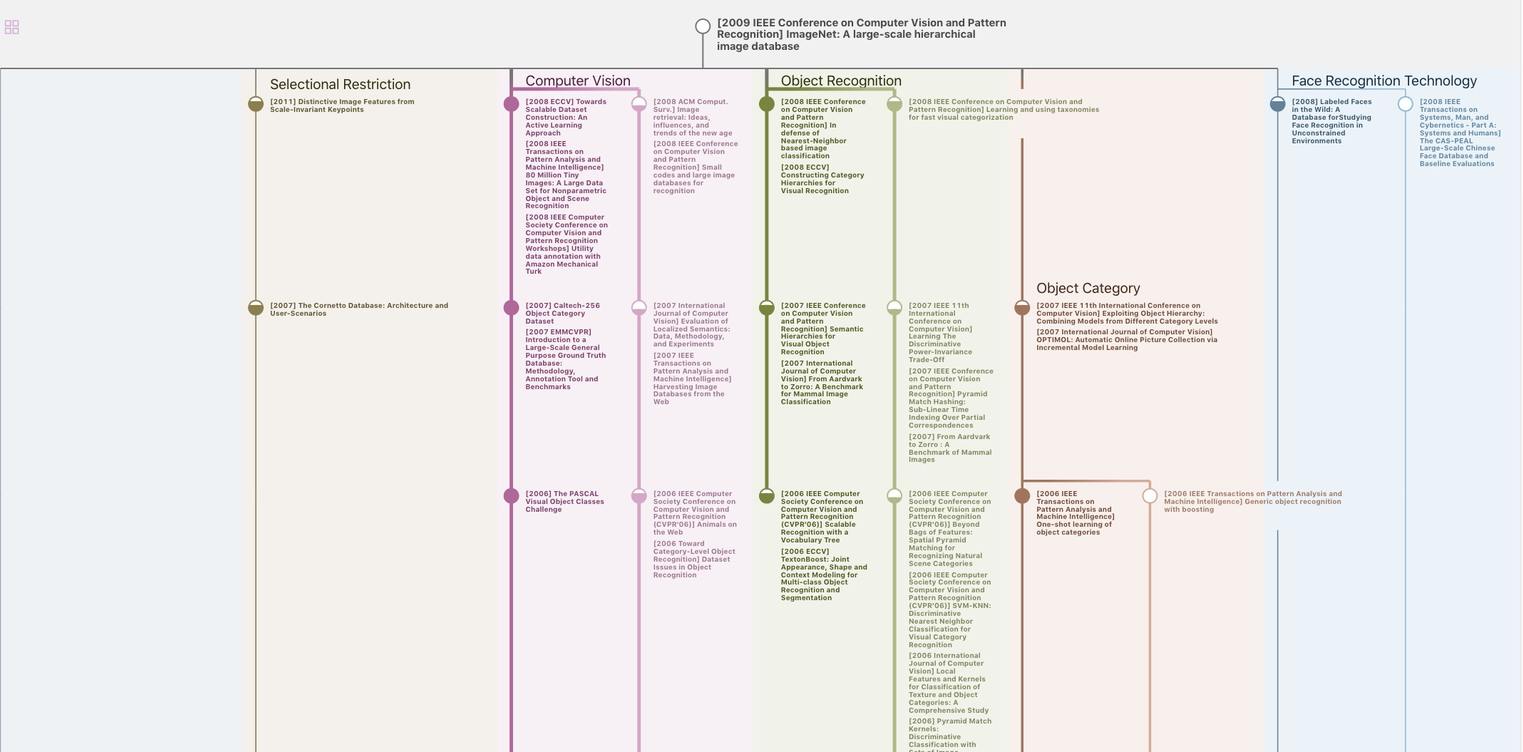
生成溯源树,研究论文发展脉络
Chat Paper
正在生成论文摘要