Tensor Decomposition of Large-scale Clinical EEGs Reveals Interpretable Patterns of Brain Physiology
arxiv(2023)
摘要
Identifying abnormal patterns in electroencephalography (EEG) remains the cornerstone of diagnosing several neurological diseases. The current clinical EEG review process relies heavily on expert visual review, which is unscalable and error-prone. In an effort to augment the expert review process, there is a significant interest in mining population-level EEG patterns using unsupervised approaches. Current approaches rely either on two-dimensional decompositions (e.g., principal and independent component analyses) or deep representation learning (e.g., auto-encoders, self-supervision). However, most approaches do not leverage the natural multi-dimensional structure of EEGs and lack interpretability. In this study, we propose a tensor decomposition approach using the canonical polyadic decomposition to discover a parsimonious set of population-level EEG patterns, retaining the natural multi-dimensional structure of EEG recordings (timexspacexfrequency). We then validate their clinical value using a cohort of patients with varying stages of cognitive impairment. Our results show that the discovered patterns reflect physiologically meaningful features and accurately classify the stages of cognitive impairment (healthy vs mild cognitive impairment vs Alzheimer's dementia) with substantially fewer features compared to classical and deep learning-based baselines. We conclude that the decomposition of population-level EEG tensors recovers expert-interpretable EEG patterns that can aid in studying smaller specialized clinical cohorts.
更多查看译文
关键词
EEG,neurological disorders,tensor decomposition,unsupervised learning,interpretability
AI 理解论文
溯源树
样例
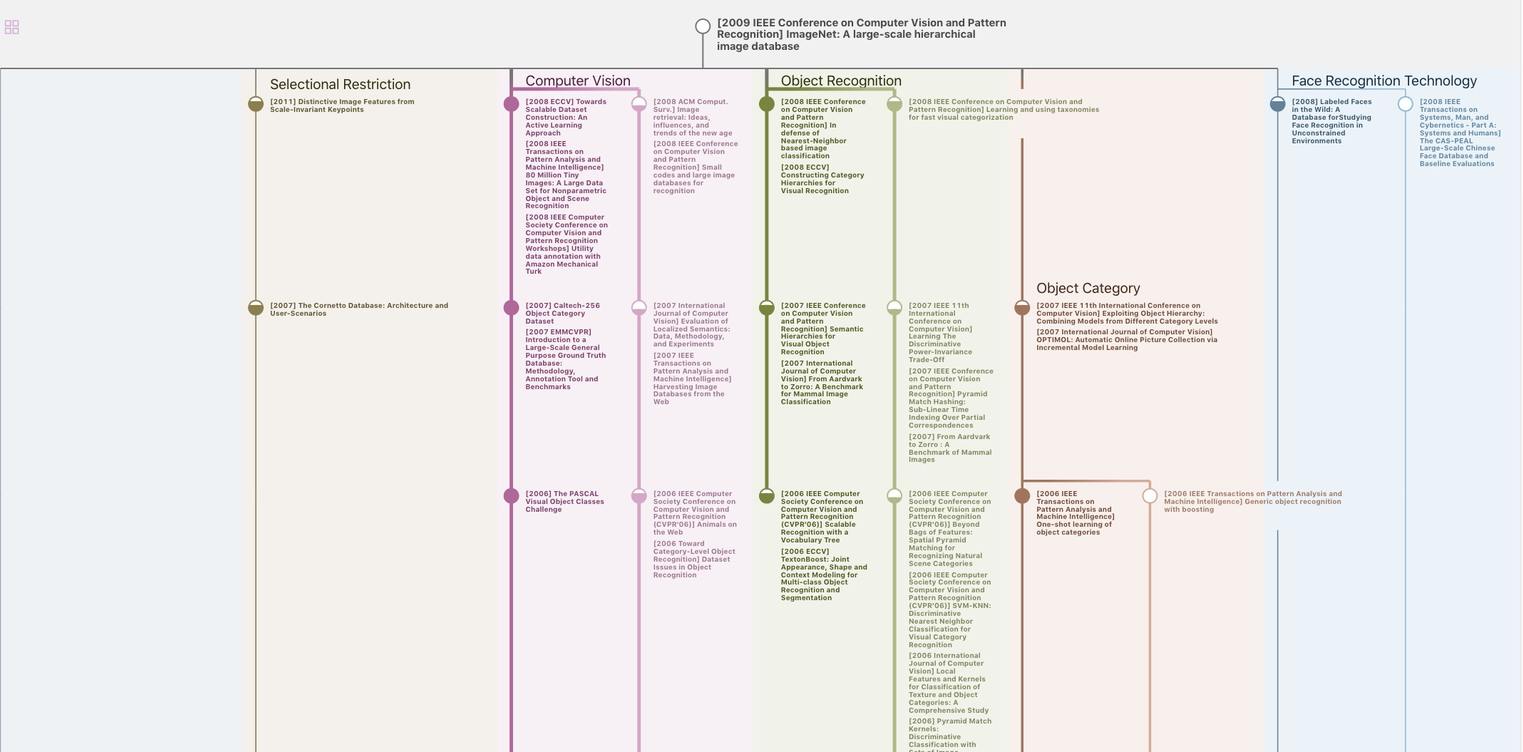
生成溯源树,研究论文发展脉络
Chat Paper
正在生成论文摘要