On Designing Light-Weight Object Trackers through Network Pruning: Use CNNs or Transformers?
arxiv(2023)
摘要
Object trackers deployed on low-power devices need to be light-weight, however, most of the current state-of-the-art (SOTA) methods rely on using compute-heavy backbones built using CNNs or transformers. Large sizes of such models do not allow their deployment in low-power conditions and designing compressed variants of large tracking models is of great importance. This paper demonstrates how highly compressed light-weight object trackers can be designed using neural architectural pruning of large CNN and transformer based trackers. Further, a comparative study on architectural choices best suited to design light-weight trackers is provided. A comparison between SOTA trackers using CNNs, transformers as well as the combination of the two is presented to study their stability at various compression ratios. Finally results for extreme pruning scenarios going as low as 1% in some cases are shown to study the limits of network pruning in object tracking. This work provides deeper insights into designing highly efficient trackers from existing SOTA methods.
更多查看译文
关键词
object tracking,light-weight,convolutional network,Transformers,network pruning
AI 理解论文
溯源树
样例
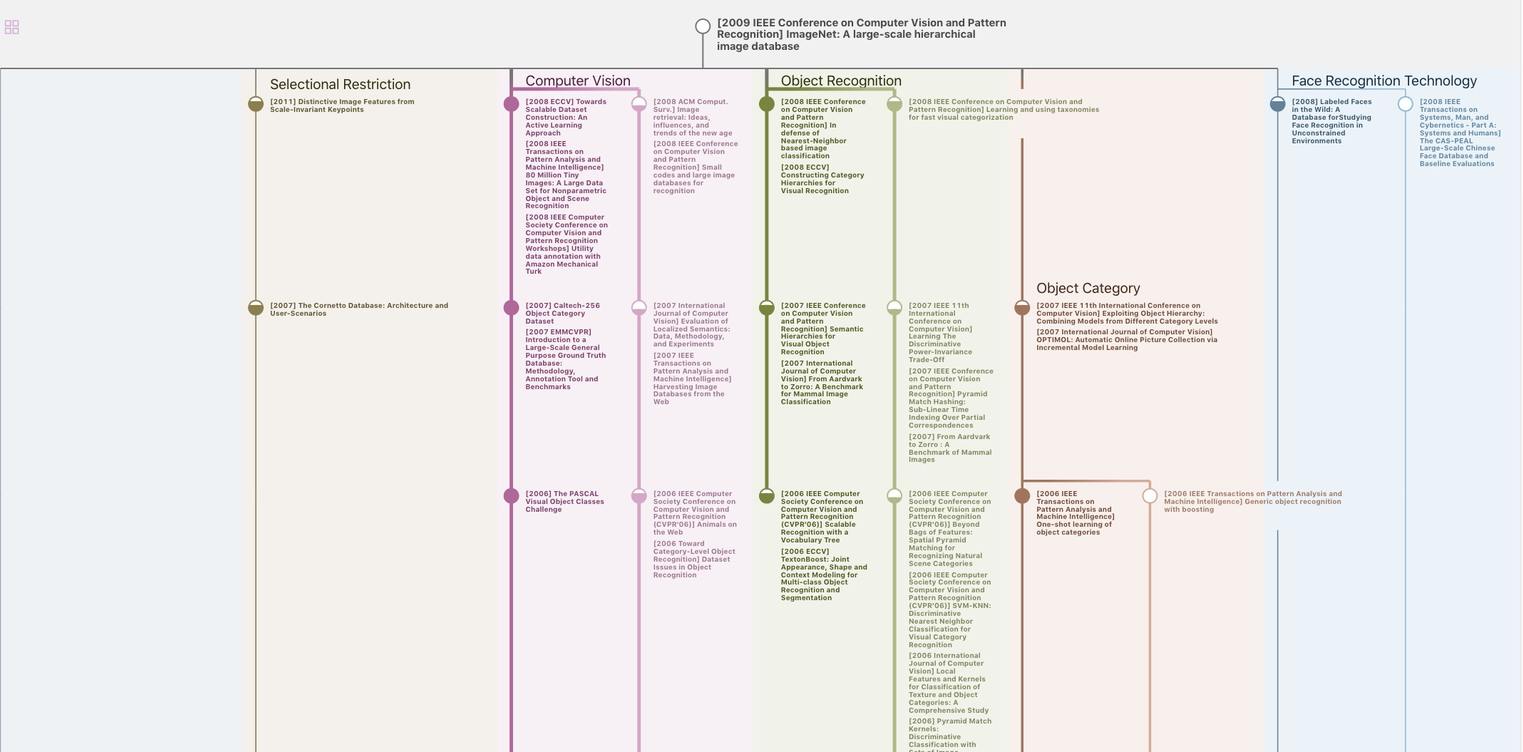
生成溯源树,研究论文发展脉络
Chat Paper
正在生成论文摘要