Relating Regularization and Generalization through the Intrinsic Dimension of Activations
arxiv(2022)
摘要
Given a pair of models with similar training set performance, it is natural to assume that the model that possesses simpler internal representations would exhibit better generalization. In this work, we provide empirical evidence for this intuition through an analysis of the intrinsic dimension (ID) of model activations, which can be thought of as the minimal number of factors of variation in the model's representation of the data. First, we show that common regularization techniques uniformly decrease the last-layer ID (LLID) of validation set activations for image classification models and show how this strongly affects generalization performance. We also investigate how excessive regularization decreases a model's ability to extract features from data in earlier layers, leading to a negative effect on validation accuracy even while LLID continues to decrease and training accuracy remains near-perfect. Finally, we examine the LLID over the course of training of models that exhibit grokking. We observe that well after training accuracy saturates, when models ``grok'' and validation accuracy suddenly improves from random to perfect, there is a co-occurent sudden drop in LLID, thus providing more insight into the dynamics of sudden generalization.
更多查看译文
关键词
generalization,intrinsic
AI 理解论文
溯源树
样例
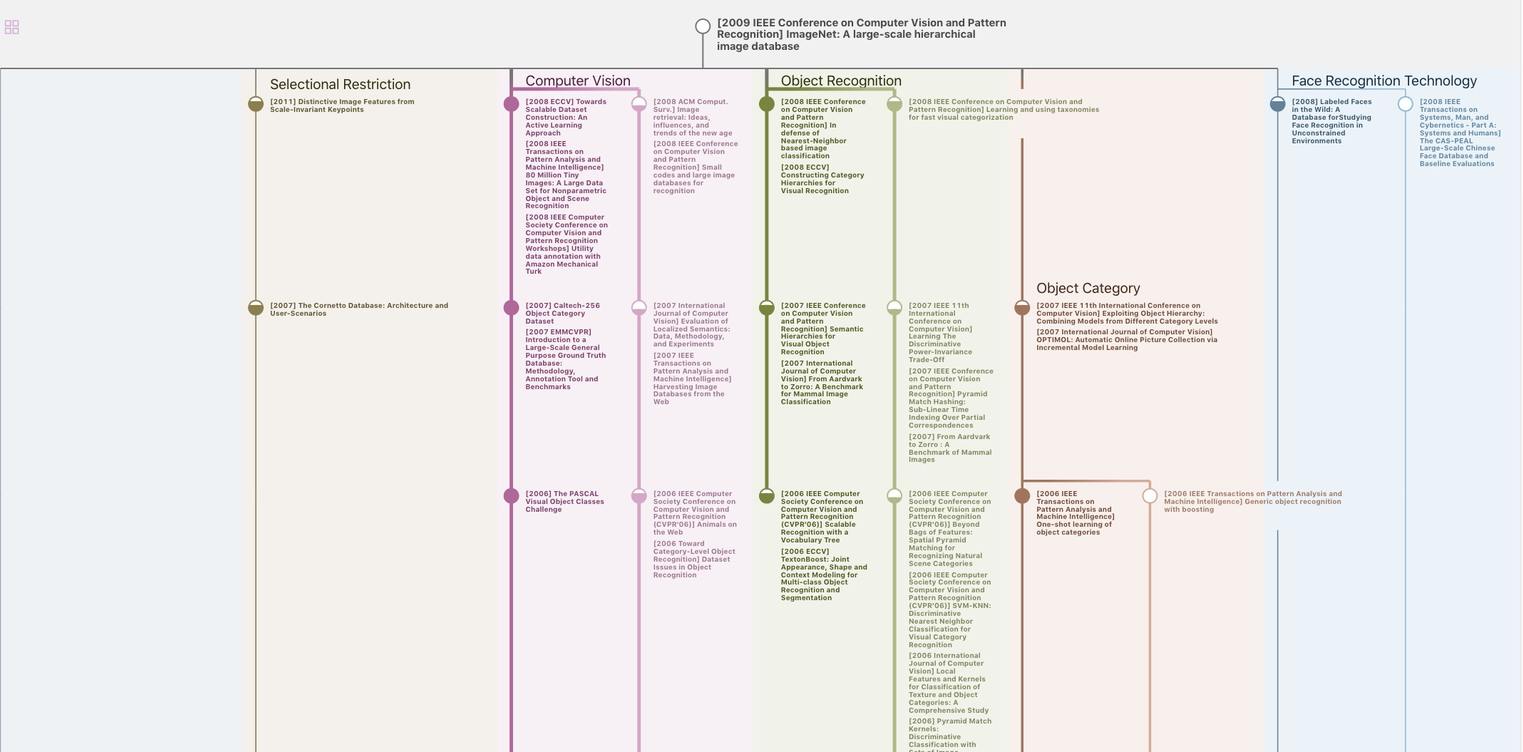
生成溯源树,研究论文发展脉络
Chat Paper
正在生成论文摘要