In-situ prediction of machining errors of thin-walled parts: an engineering knowledge based sparse Bayesian learning approach
Journal of Intelligent Manufacturing(2024)
摘要
Thin-walled parts such as blades are widely used in aerospace field, and their machining quality directly affects the service performance of core components. Due to obvious time-varying nonlinear effect and complex machining system, it is a great challenge to realize accurate and fast prediction of machining errors of such parts. To solve the above problems, an engineering knowledge based sparse Bayesian learning approach is proposed to realize in-situ prediction of machining errors of thin-walled blades. Firstly, an engineering knowledge based strategy is proposed to improve the generalization ability of the model by integrating multi-source engineering knowledge, including machining information, physical information and online monitoring information. Then, principal component analysis method is utilized for the dimensional reduction of features. Sparse Bayesian learning approach is developed for model training, which significantly reduce the complexity of the regression model. Finally, the superiority and effectiveness of the proposed approach have been proven in blade milling experiments. Experimental results show that the average deviation of the proposed in-situ prediction model is about 11 μm, and the model complexity is reduced by 66%.
更多查看译文
关键词
Machining errors,Thin-walled parts,In-situ prediction,Engineering knowledge based,Sparse Bayesian learning
AI 理解论文
溯源树
样例
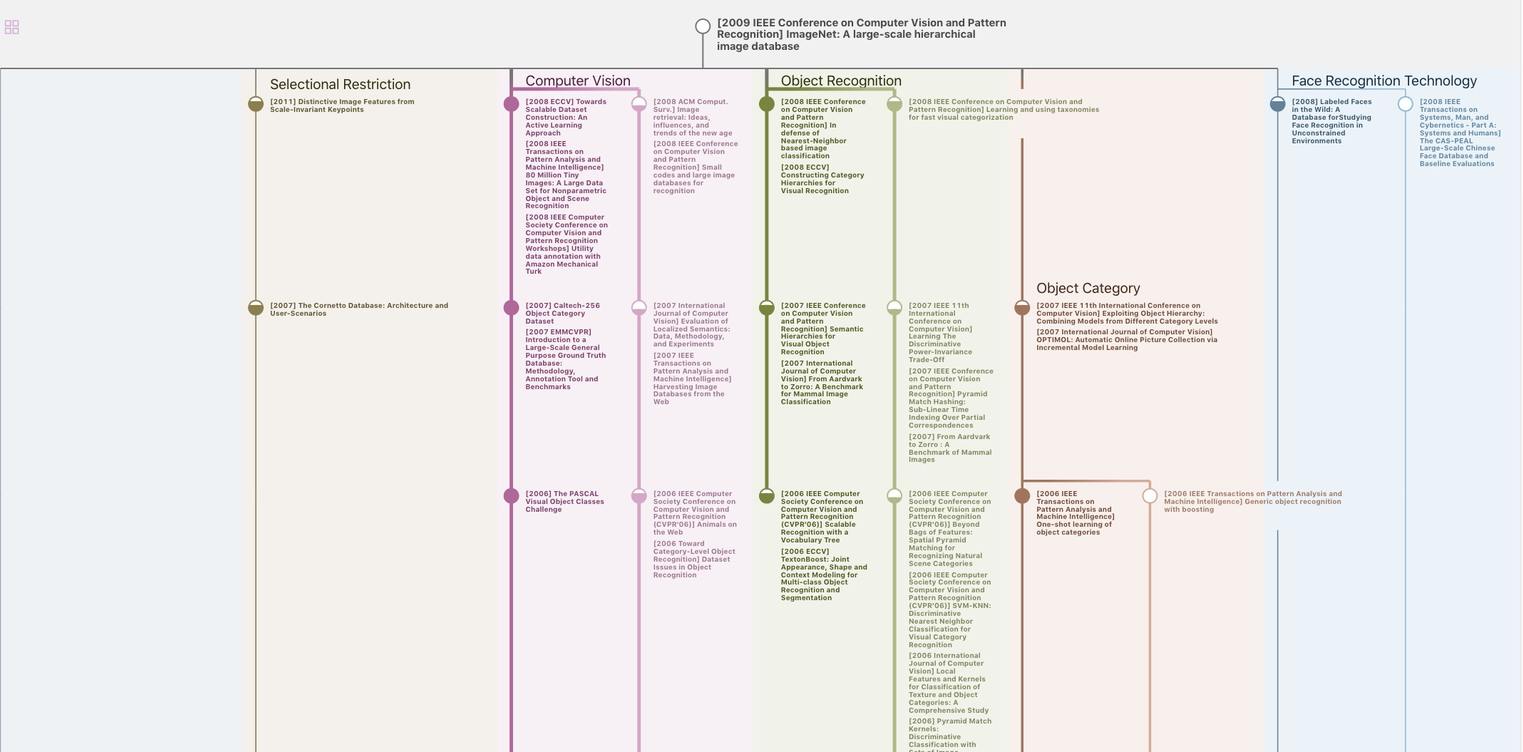
生成溯源树,研究论文发展脉络
Chat Paper
正在生成论文摘要