Masked Face Transformer
IEEE TRANSACTIONS ON INFORMATION FORENSICS AND SECURITY(2024)
摘要
The COVID-19 pandemic makes wearing masks mandatory. Existing CNN-based face recognition (FR) systems suffer from severe performance degradation as masks occlude the vital facial regions. Recently, Vision Transformers have shown promising performance in various vision tasks with quadratic computation costs. Swin Transformer first proposes a successive window attention mechanism allowing the cross-window connection and more computational efficiency. Despite its potential, the deployment of Swin Transformer in masked face recognition encounters two challenges: 1) the attention range is insufficient to capture locally compatible face regions. 2) Masked face recognition can be defined as an occlusion-robust classification task with a known occlusion position, i.e., the position of the mask is minor-varying, which is overlooked but efficient in improving the model's recognition accuracy. To alleviate the above problem, we propose a Masked Face Transformer (MFT) with Masked Face-compatible Attention (MFA). The proposed MFA 1) introduces two additional window partition configurations, e.g., row shift and column shift, to enlarge the attention range in Swin with invariant computation costs, and 2) suppresses the interaction between the masked and non-masked regions to retain their discrepancies. Additionally, as mask occlusion leads to a separation between the masked and non-masked samples of the same identity, we propose to explore the relationship between them by a ClassFormer module to enhance intra-class aggregation. Extensive experiments show that MFT outperforms state-of-the-art masked face recognition methods in both simulated and real masked face testing datasets.
更多查看译文
关键词
Face recognition,Transformers,Feature extraction,Training,Task analysis,Costs,COVID-19,Masked face recognition,face recognition,transformer
AI 理解论文
溯源树
样例
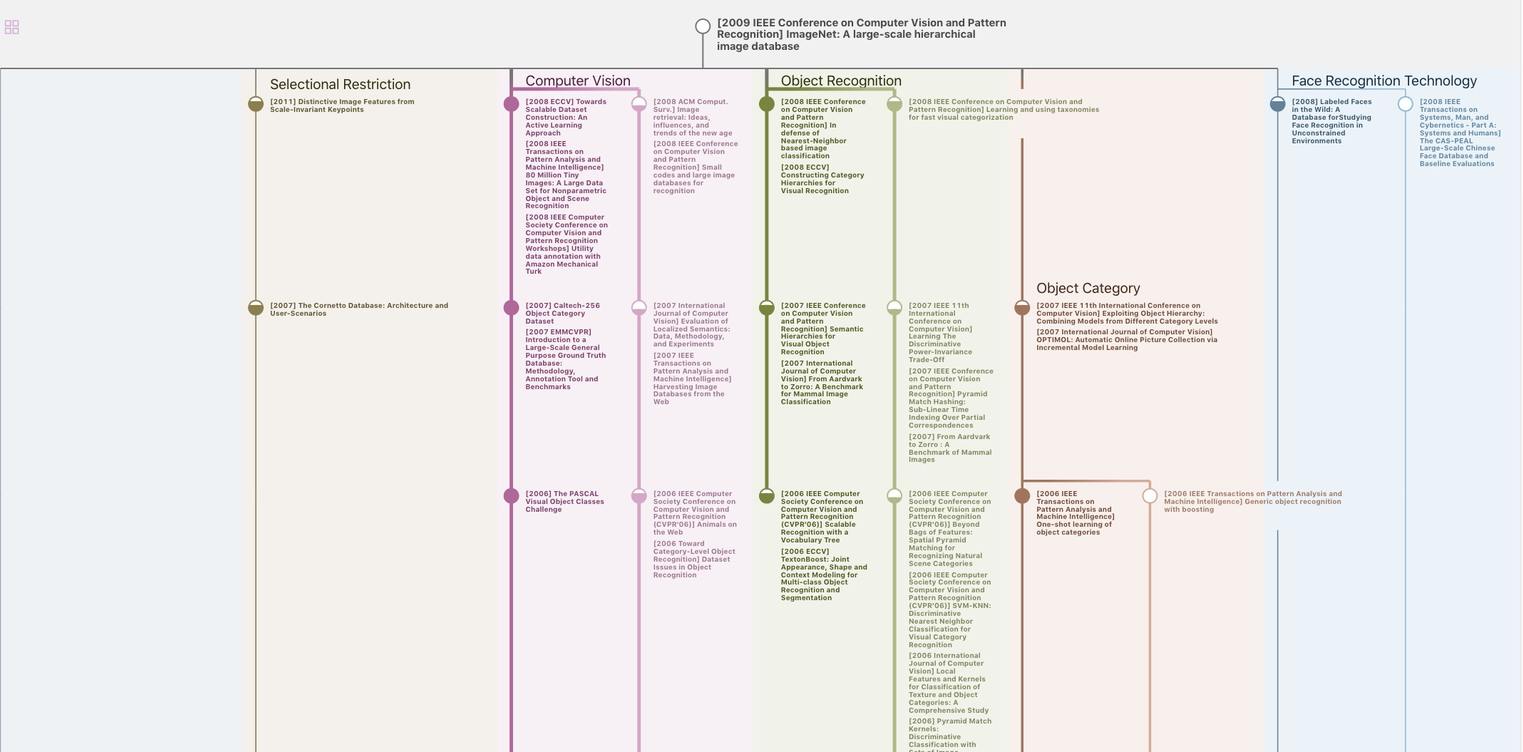
生成溯源树,研究论文发展脉络
Chat Paper
正在生成论文摘要