Emergent perceptual biases from state-space geometry in spiking recurrent neural networks trained to discriminate time intervals
biorxiv(2023)
摘要
A stimulus held in working memory is perceived as contracted towards the average stimulus. This contraction bias has been extensively studied in psychophysics, but little is known about its origin from neural activity. By training recurrent networks of spiking neurons to discriminate temporal intervals, we explored the causes of this bias and how behavior relates to population firing activity. We found that the trained networks exhibited animal-like behavior. Various geometric features of neural trajectories in state space encoded warped representations of the durations of the first interval modulated by sensory history. Formulating a novel normative model, we showed that these representations conveyed a Bayesian estimate of the interval durations, thus relating activity and behavior. Importantly, our findings demonstrate that Bayesian computations already occur during the sensory phase of the first stimulus and persist throughout its maintenance in working memory, until the time of stimulus comparison.
### Competing Interest Statement
The authors have declared no competing interest.
更多查看译文
关键词
emergent perceptual biases,recurrent neural networks,neural networks,time intervals,state-space
AI 理解论文
溯源树
样例
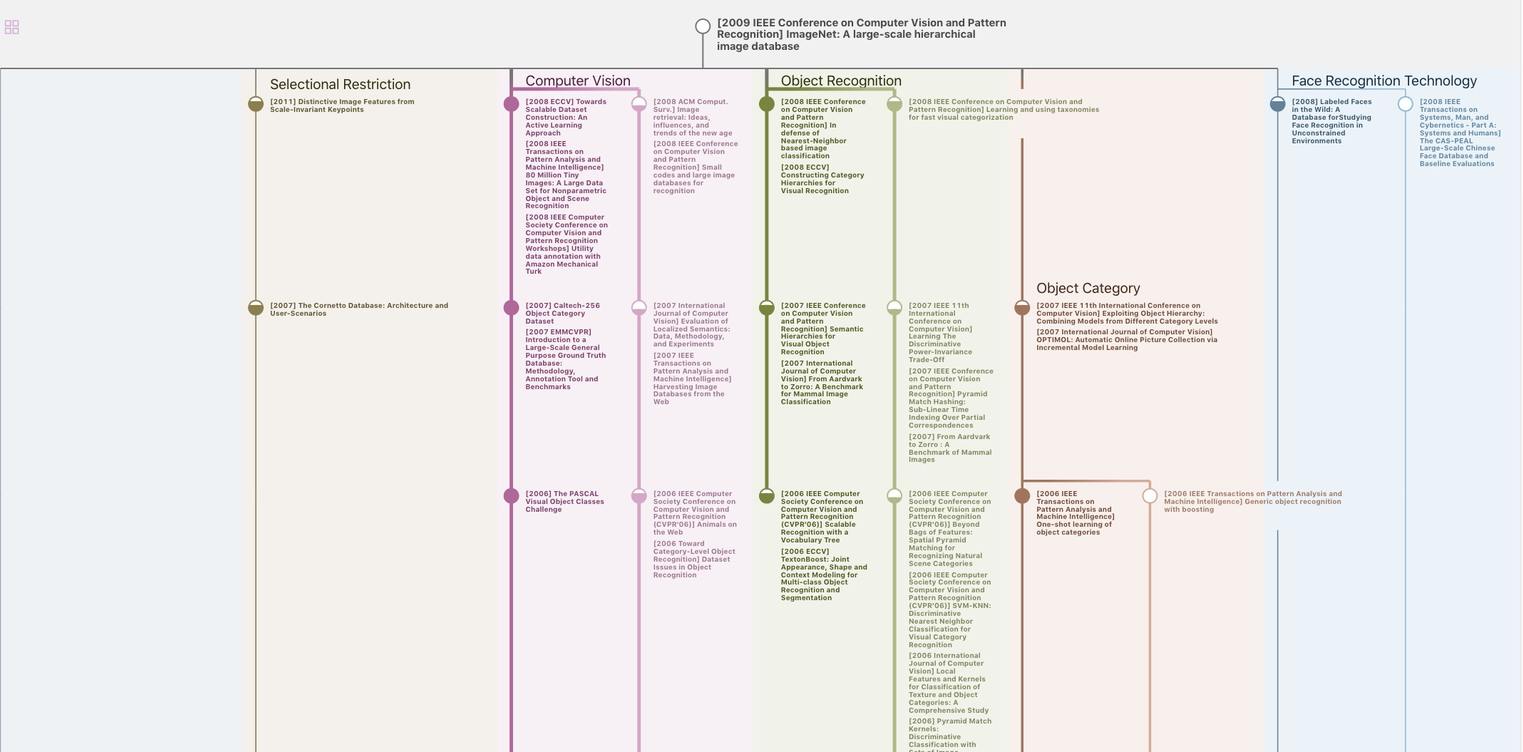
生成溯源树,研究论文发展脉络
Chat Paper
正在生成论文摘要