Depth-aware inverted refinement network for RGB-D salient object detection
Neurocomputing(2023)
摘要
Recent advances in multi-modal feature fusion boost the development of RGB-D salient object detection (SOD), and many remarkable RGB-D SOD models have been proposed. However, though some existing methods consider fusing the cross-level multi-modal features, they ignore the difference between inter-level having the multi-modal details in convolutional neural networks (CNNs) based RGB-D SOD. Therefore, exploring the correlations and differences of cross-level multi-modal features is a critical issue. In this paper, we present a novel depth-aware inverted refinement network (DAIR) to progressively guide the cross-level multi-modal features through backward propagation, which considerably preserves the different level details with multi-modal cues. Specifically, we innovatively design an end-to-end inverted refinement network to guide cross-level and cross-modal learning for revealing complementary relations of the cross-modal. The inverted refinement network also refines the low-level spatial details by the high-level global contextual cues. In particular, considering the difference of multi-modal and the effect of depth quality, a depth-aware intensified module (DAIM) is proposed with capturing the paired relationship of the pixel-level and inter-channel for the depth map. It promotes the representative capability of the depth details. Extensive experiments on nine challenging RGB-D SOD datasets demonstrate remarkable performance boosting of our proposed model against the fourteen state-of-the-art (SOTA) RGB-D SOD approaches.
更多查看译文
关键词
Salient object detection,RGB-D image,Inverted refinement,Cross-level multi-modal features
AI 理解论文
溯源树
样例
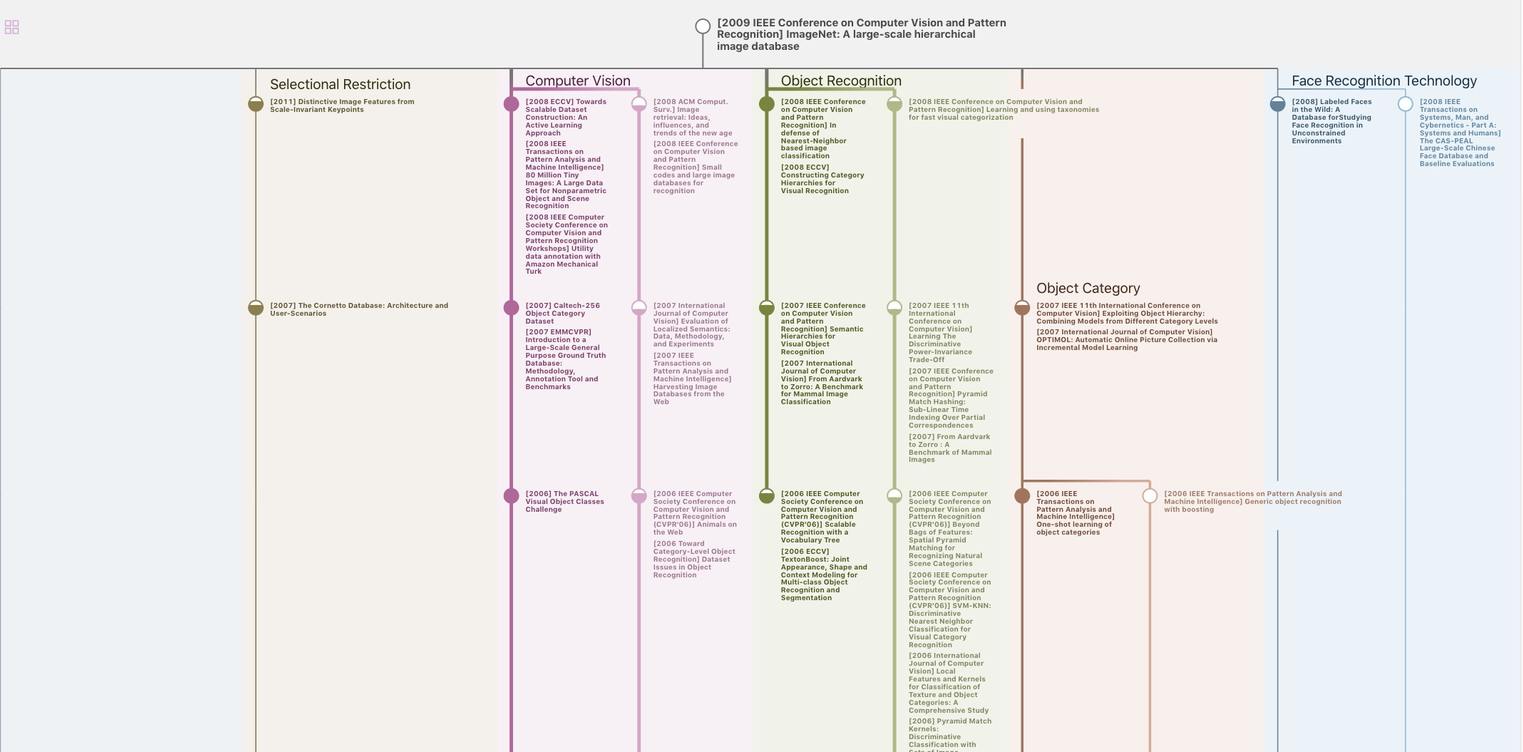
生成溯源树,研究论文发展脉络
Chat Paper
正在生成论文摘要