Camera-aware representation learning for person re-identification
Neurocomputing(2023)
摘要
Person re-identification (ReID) aims to associate the same person across non-overlapping cameras. However, most of existing works neglect the issue of camera-imbalanced data distribution. Consequently, pedestrian representation learning gives preference to the head cameras, which have comparatively more training data, and disregards the tail cameras, which have relatively less training data. In this paper, we propose a novel framework for camera-aware representation learning to overcome this issue, named CARL. On the proxy level representation learning, CARL presents a multiple-center softmax loss to correct the head camera bias and presents a hard sub-center mining strategy to improve the discrimination of tail camera samples. On the pair-wise level representation learning, CARL builds a camera-balanced memory bank (CBM) to re-balance the sample pair distribution and proposes a camera-paired loss for pair-wise metric learning. Extensive experiments and ablation studies on MSMT17, the current largest ReID dataset with massive camera-imbalanced data distribution, demonstrate that our CARL is superior to previous metric learning based ReID methods and achieves state-of-the-art performance.
更多查看译文
关键词
Camera-imbalanced data distribution,Sub-center hard mining,Camera-balanced memory bank,Multiple-center Softmax
AI 理解论文
溯源树
样例
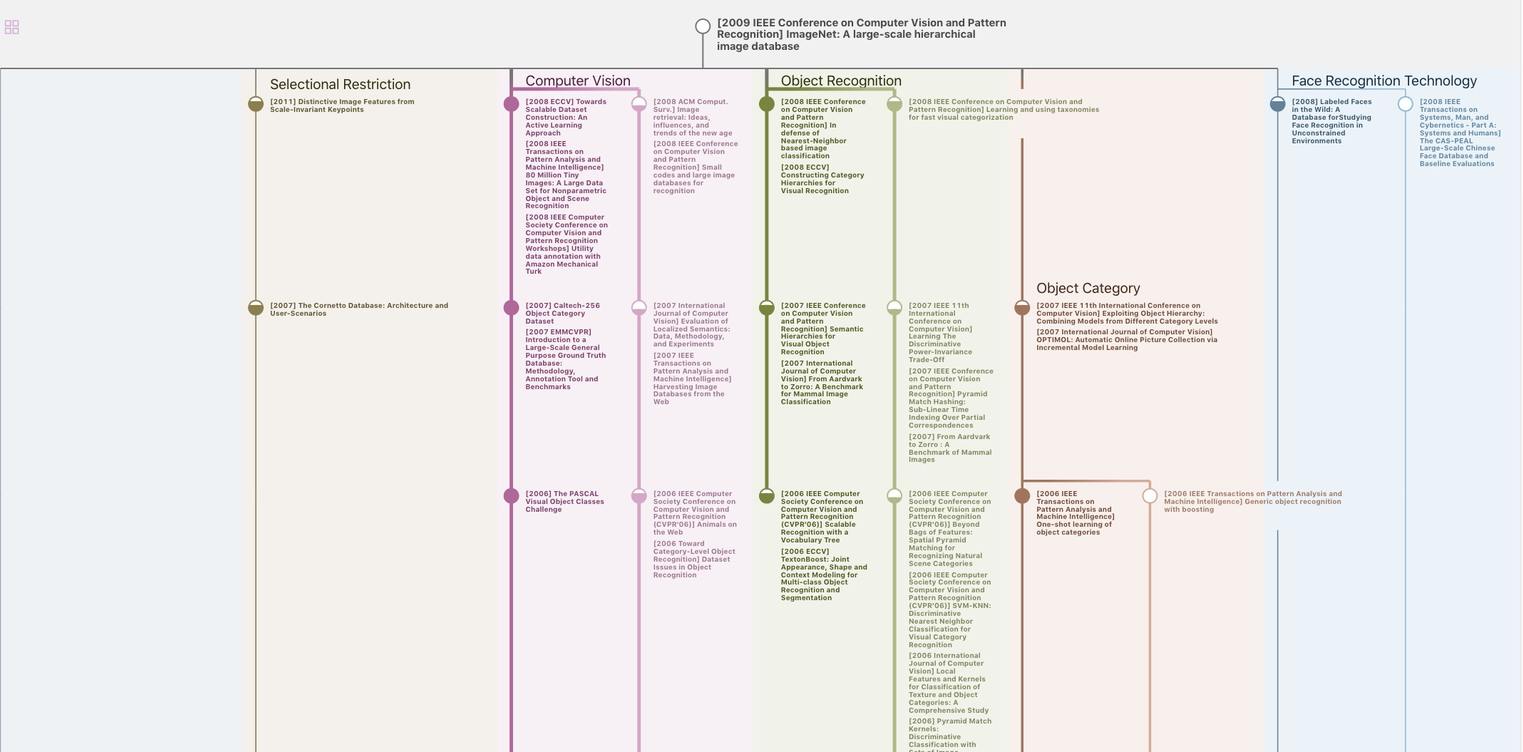
生成溯源树,研究论文发展脉络
Chat Paper
正在生成论文摘要