Radiomics for Discriminating Benign and Malignant Salivary Gland Tumors; Which Radiomic Feature Categories and MRI Sequences Should Be Used?
CANCERS(2022)
摘要
Simple Summary MRI radiomics shows promise in discriminating salivary gland tumors (SGTs) but a consistent radiomics signature has not emerged, partly due to the multitude of initial features fed into the radiomics pipeline. In this study, we investigated the impact of reducing the number of initial radiomic features on the performance of the radiomic models to discriminate between benign and malignant SGTs, by applying six feature categories separately and all feature categories in combination from three anatomical-based MRI sequences. The best models were built by a combination of T1-weighted + logarithm and fat-suppressed T2-weighted + exponential features, which reduced the initial features by 94.0% (from 1015 x 3 to 91 x 2) and achieved an average area under the curve of 0.846. Our results show reducing the number of radiomic features initially analyzed improved feature selection stability without compromising performance. This approach may improve future consensus building on a radiomics signature for discriminating SGTs. The lack of a consistent MRI radiomic signature, partly due to the multitude of initial feature analyses, limits the widespread clinical application of radiomics for the discrimination of salivary gland tumors (SGTs). This study aimed to identify the optimal radiomics feature category and MRI sequence for characterizing SGTs, which could serve as a step towards obtaining a consensus on a radiomics signature. Preliminary radiomics models were built to discriminate malignant SGTs (n = 34) from benign SGTs (n = 57) on T1-weighted (T1WI), fat-suppressed (FS)-T2WI and contrast-enhanced (CE)-T1WI images using six feature categories. The discrimination performances of these preliminary models were evaluated using 5-fold-cross-validation with 100 repetitions and the area under the receiver operating characteristic curve (AUC). The differences between models' performances were identified using one-way ANOVA. Results show that the best feature categories were logarithm for T1WI and CE-T1WI and exponential for FS-T2WI, with AUCs of 0.828, 0.754 and 0.819, respectively. These AUCs were higher than the AUCs obtained using all feature categories combined, which were 0.750, 0.707 and 0.774, respectively (p < 0.001). The highest AUC (0.846) was obtained using a combination of T1WI + logarithm and FS-T2WI + exponential features, which reduced the initial features by 94.0% (from 1015 x 3 to 91 x 2). CE-T1WI did not improve performance. Using one feature category rather than all feature categories combined reduced the number of initial features without compromising radiomic performance.
更多查看译文
关键词
radiomics,salivary gland neoplasms,conventional magnetic resonance imaging
AI 理解论文
溯源树
样例
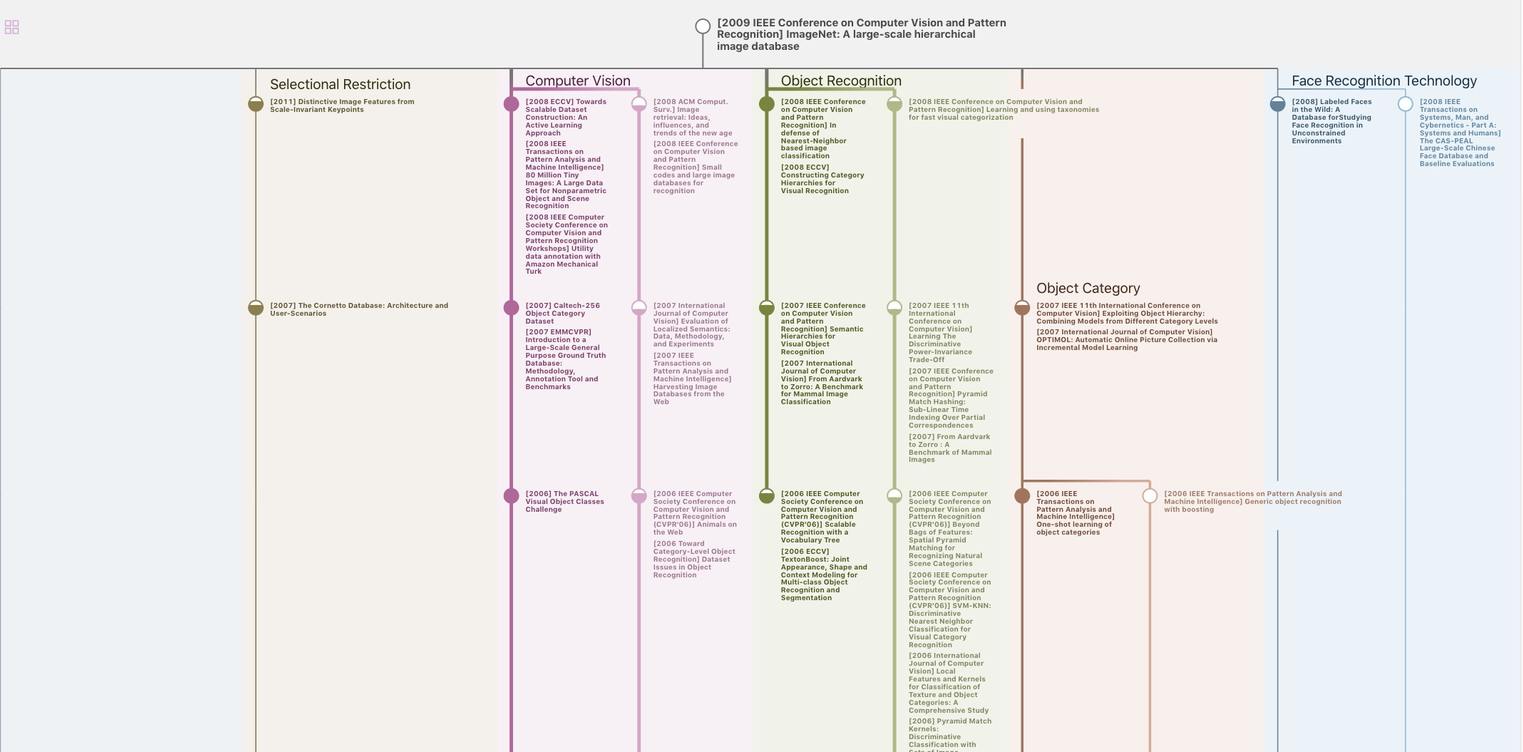
生成溯源树,研究论文发展脉络
Chat Paper
正在生成论文摘要