Learning Outcome Modeling in Computer-Based Assessments for Learning: A Sequential Deep Collaborative Filtering Approach
IEEE Transactions on Learning Technologies(2023)
摘要
Learning outcome modeling is a technical underpinning for the successful evaluation of learners' learning outcomes through computer-based assessments. In recent years, collaborative filtering approaches have gained popularity as a technique to model learners' item responses. However, how to model the temporal dependencies between item responses using a collaborative filtering approach for learning outcome modeling is still under investigation. Leveraging the advantages of deep learning, this study proposes a novel deep-learning-based collaborative filtering approach for learning outcome modeling. Unlike conventional collaborative filtering approaches, the proposed model, utilizing recurrent neural networks, is capable of sequentially predicting learners' future learning outcomes based on their history learning records. Moreover, the proposed model has the capacity to discover item-skill associations from the scratch without expert input based on attentive modeling. The experimental results demonstrate that the proposed model outperforms a popular deep-learning approach and can be successfully used to discover item-skill associations for both real-world and synthetic datasets.
更多查看译文
关键词
Data models,Predictive models,Computational modeling,Bayes methods,Recommender systems,Deep learning,Collaboration,Attentive modeling,collaborative filtering (CF),deep learning,item-skill associations,learning outcome modeling,recurrent neural networks (RNNs)
AI 理解论文
溯源树
样例
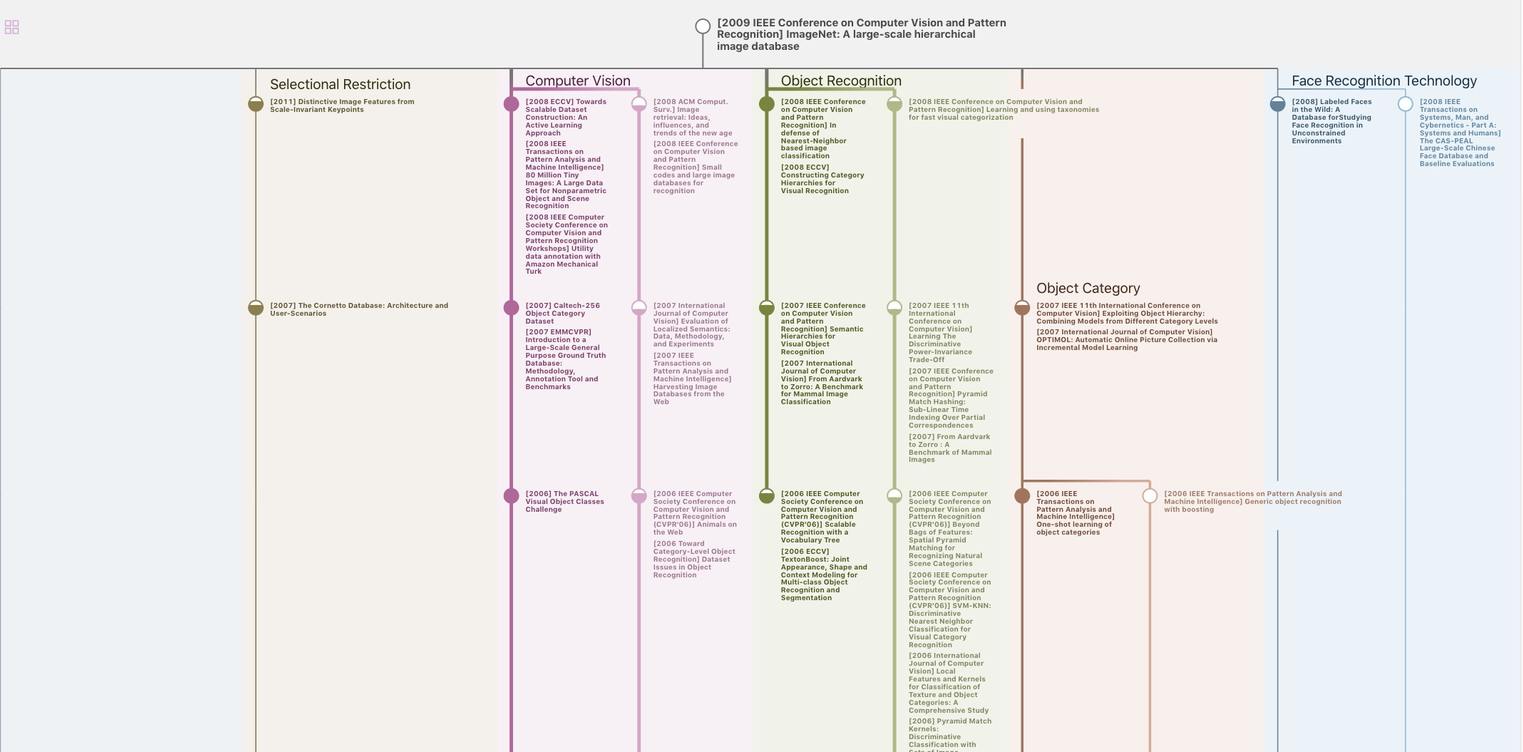
生成溯源树,研究论文发展脉络
Chat Paper
正在生成论文摘要